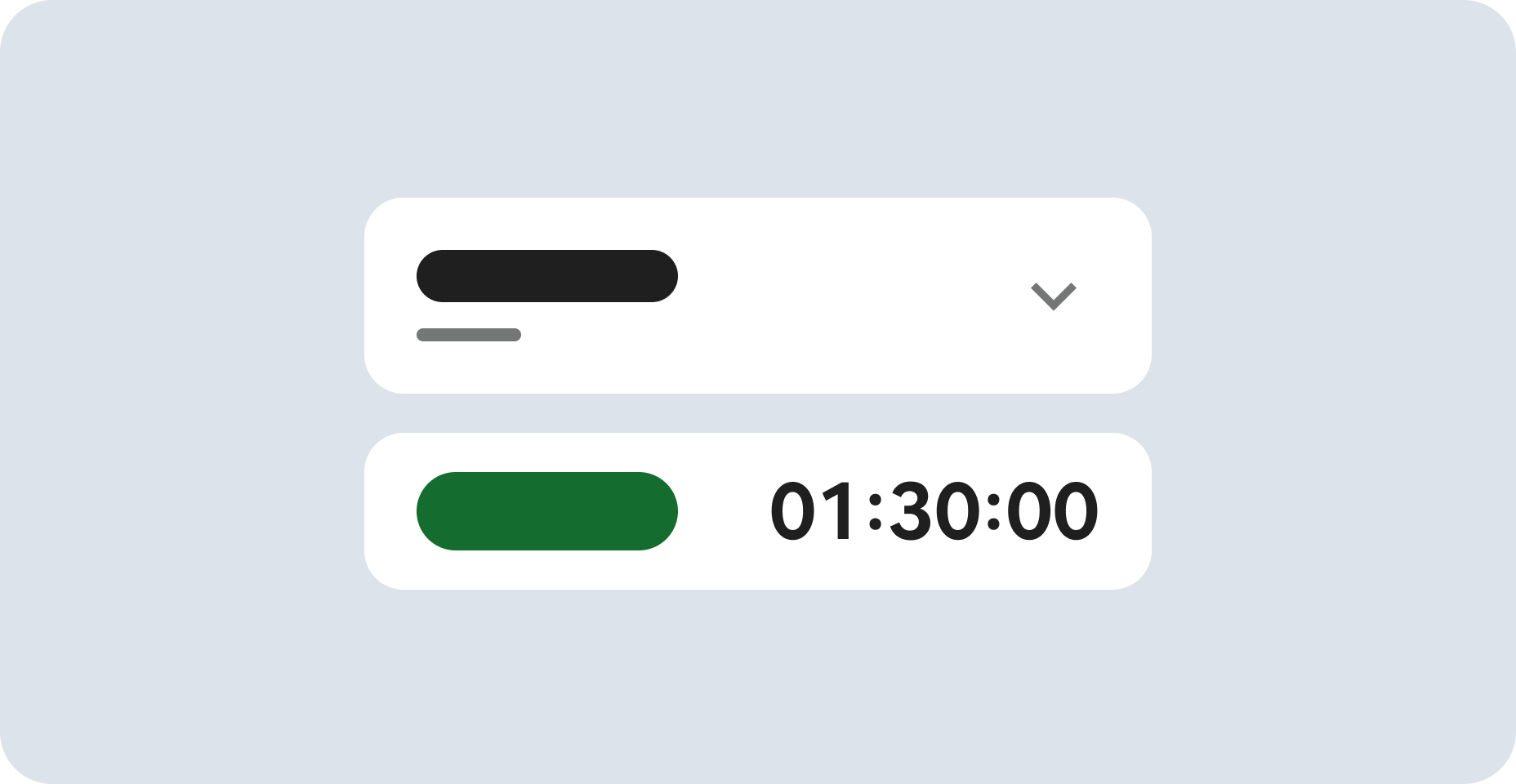
Before you begin
- Labs create a Google Cloud project and resources for a fixed time
- Labs have a time limit and no pause feature. If you end the lab, you'll have to restart from the beginning.
- On the top left of your screen, click Start lab to begin
Get Started with Vector Search
/ 30
Create an Index
/ 40
Query the Index
/ 30
Vector Search can search from billions of semantically similar or semantically related items. A vector similarity-matching service has many use cases such as implementing recommendation engines, search engines, chatbots, and text classification. Semantic matching can be simplified into a few steps. First, you must generate embedding representations of many items (done outside of Vector Search). Secondly, you upload your embeddings to Google Cloud, and then link your data to Vector Search. After your embeddings are added to Vector Search, you can create an index to run queries to get recommendations or results.
The use of embeddings is not limited to words or text. You can generate semantic embeddings for many kinds of data, including images, audio, video, and user preferences. For generating a multimodal embedding with Vertex AI, see Get multimodal embeddings. In this lab, you will learn how to use Vertex AI Embeddings for text to create text embeddings and use them to create a vector search index.
In this lab, you will perform the following tasks:
Read these instructions. Labs are timed and you cannot pause them. The timer, which starts when you click Start Lab, shows how long Google Cloud resources are made available to you.
This hands-on lab lets you do the lab activities in a real cloud environment, not in a simulation or demo environment. It does so by giving you new, temporary credentials you use to sign in and access Google Cloud for the duration of the lab.
To complete this lab, you need:
Click the Start Lab button. If you need to pay for the lab, a dialog opens for you to select your payment method. On the left is the Lab Details pane with the following:
Click Open Google Cloud console (or right-click and select Open Link in Incognito Window if you are running the Chrome browser).
The lab spins up resources, and then opens another tab that shows the Sign in page.
Tip: Arrange the tabs in separate windows, side-by-side.
If necessary, copy the Username below and paste it into the Sign in dialog.
You can also find the Username in the Lab Details pane.
Click Next.
Copy the Password below and paste it into the Welcome dialog.
You can also find the Password in the Lab Details pane.
Click Next.
Click through the subsequent pages:
After a few moments, the Google Cloud console opens in this tab.
In the Google Cloud console, on the Navigation menu (), click Vertex AI > Workbench.
Find the
The JupyterLab interface for your Workbench instance opens in a new browser tab.
In the left panel, double-click the intro-textemb-vectorsearch.ipynb notebook file to open it in the right window.
In the Select Kernel dialog, choose Python 3 from the list of available kernels.
Scroll down to the Text Embeddings in Action section, and run the setup cells.
When setting your environment variables, use
Navigate to the Getting Started with Vertex AI Embeddings for Text section and run through the cells to create the text embeddings.
Navigate to the Getting Started with Vector Search section and run through the cells.
Save the embeddings in a JSON file.
Create a new Cloud Storage bucket and copy the file to it.
Click Check my progress to verify the objective.
Click Check my progress to verify the objective.
In this task, you will explore the Vector search notebook and try the public demo. Since index creation and deployment takes ~30 minutes, you can try the public demo and explore the notebook while you wait.
test_embeddings
variable to see different results.Click Check my progress to verify the objective.
Congratulations! In this lab, you learned how to create text embeddings and use them to create a vector search index. You are now ready to use text embeddings in your own applications!
Check out the following resources for more information on text embeddings and vector search:
...helps you make the most of Google Cloud technologies. Our classes include technical skills and best practices to help you get up to speed quickly and continue your learning journey. We offer fundamental to advanced level training, with on-demand, live, and virtual options to suit your busy schedule. Certifications help you validate and prove your skill and expertise in Google Cloud technologies.
Manual Last Updated January 28, 2025
Lab Last Tested January 28, 2025
Copyright 2025 Google LLC All rights reserved. Google and the Google logo are trademarks of Google LLC. All other company and product names may be trademarks of the respective companies with which they are associated.
This content is not currently available
We will notify you via email when it becomes available
Great!
We will contact you via email if it becomes available
One lab at a time
Confirm to end all existing labs and start this one