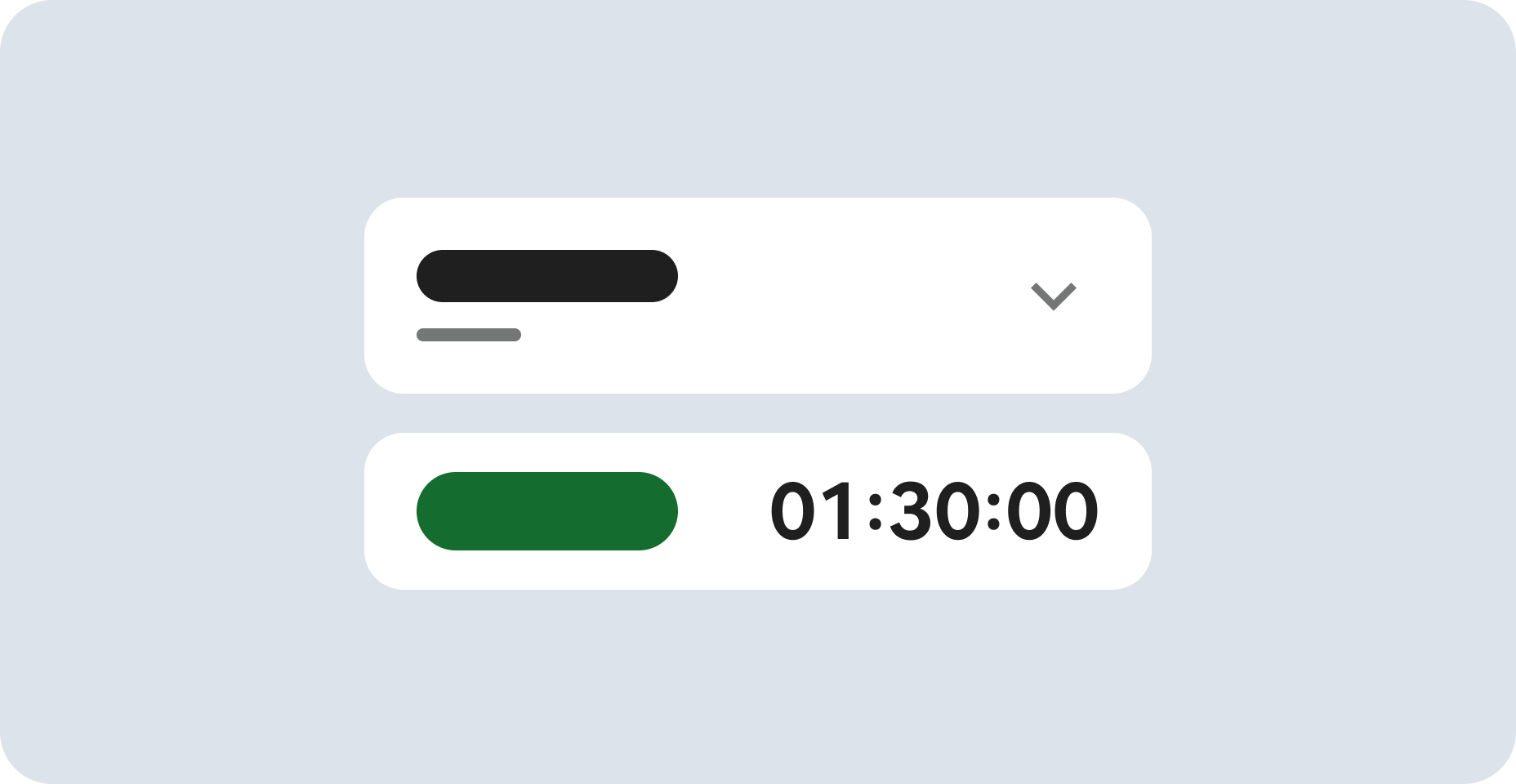
Before you begin
- Labs create a Google Cloud project and resources for a fixed time
- Labs have a time limit and no pause feature. If you end the lab, you'll have to restart from the beginning.
- On the top left of your screen, click Start lab to begin
Enable sensitive data protection for Cloud Storage
/ 40
Enable sensitive data protection for BigQuery
/ 30
Protect sensitive data in Gen AI model responses
/ 30
In a challenge lab you’re given a scenario and a set of tasks. Instead of following step-by-step instructions, you will use the skills learned from the labs in the course to figure out how to complete the tasks on your own! An automated scoring system (shown on this page) will provide feedback on whether you have completed your tasks correctly.
When you take a challenge lab, you will not be taught new Google Cloud concepts. You are expected to extend your learned skills, like changing default values and reading and researching error messages to fix your own mistakes.
To score 100% you must successfully complete all tasks within the time period!
This lab is recommended for students who have enrolled in the Discover and Protect Sensitive Data Across Your Ecosystem course. Are you ready for the challenge?
You are a data engineer at Cymbal Cars and have been tasked with identifying and protecting sensitive data for your customers (car owners) across your organization's data ecosystem.
Your colleagues have previously completed some work to identify and redact sensitive data in your organization's Cloud Storage files and BigQuery tables (particularly US Social Security numbers) and in your organization's Gen AI model responses.
To ensure your Cloud Storage files and BigQuery assets continue to be periodically scanned and protected, you want to set up Sensitive Data Protection discovery and run jobs to identify and redact other sensitive data such as credit card numbers.
For your organization's Gen AI models, you also want to expand on your colleague's previous work to redact responses when credentials are identified in responses.
In this challenge, you use your knowledge of Sensitive Data Protection tools to implement discovery and protection for data in Cloud Storage and BigQuery and use the Python Client for Cloud Data Loss Prevention (DLP) API to identify and redact Gen AI model responses that contain credentials.
Throughout the lab, use the following details for this lab environment:
Read these instructions. Labs are timed and you cannot pause them. The timer, which starts when you click Start Lab, shows how long Google Cloud resources are made available to you.
This hands-on lab lets you do the lab activities in a real cloud environment, not in a simulation or demo environment. It does so by giving you new, temporary credentials you use to sign in and access Google Cloud for the duration of the lab.
To complete this lab, you need:
Your team has a Cloud Storage bucket named gs://sample-chat-log-data-10.csv
).
Your goals are to identify and redact credit card numbers in the new CSV files and enable daily discovery for the bucket to monitor for new instances of sensitive data moving forward.
To help you achieve these goals, complete the following subtasks.
Expand the hints below for some helpful guidance to get started!
Helpful hint for discovery scan!
Property | Value |
---|---|
Select scope | Scan selected project |
Managed schedules | Edit Default schedule to specify Reprofile Daily for On a schedule and When inspect template changes |
Select inspection template | Create a new inspection template |
Save data profile copies to BigQuery | Set Dataset ID to cs_discovery and Table ID to cs_data_profiles in the current project |
Set location to store configuration | Multi_region > us (multiple regions in United States) |
Display name for configuration | Cloud Storage Daily Discovery |
Helpful hint for de-identify template!
Property | Value |
---|---|
Template ID | us_ccn_deidentify |
Data transformation type | Record |
Display name | De-identify Credit Card Numbers |
Location type | Multi_region > global (Global) |
Field for Transformation Rule | message |
Transformation type | Match on infoType |
Transformation Method | Replace with infoType name |
Helpful hint for de-identify job!
Property | Value |
---|---|
Job ID | us_ccn_deidentify |
Location type | Multi_region > us (multiple regions in United States) |
URL | gs:// |
Scan recursively | Enable this option |
Sampling | 100% |
Sampling method | No sampling |
Structured de-identification template | Specify the path to the de-identify template you created in step 2 |
Export transformation details to BigQuery | Set Dataset ID to cs_transformations and Table ID to deidentify_ccn in the current project |
Cloud Storage output location | gs:// |
Click Check my progress to verify the objective.
Data on car owners and their purchases are also stored in BigQuery for analytics, and some of the datasets contain sensitive data. You have been tasked with creating a tag in IAM for sensitive personally identifiable information (SPII) and using it to grant conditional access for certain users to access only BigQuery datasets that have a tag of no SPII.
To help you achieve this goal, complete the following subtasks.
Expand the hints below for some helpful guidance to get started!
Helpful hint for creating the tag!
Property | Value |
---|---|
Tag key | SPII |
Tag key description | Flag for sensitive personally identifiable information (SPII) |
Tag key value 1 | Yes |
Tag key value 1 description | Contains sensitive personally identifiable information (SPII) |
Tag key value 2 | No |
Tag key value 2 description | Does not contain sensitive personally identifiable information (SPII) |
Helpful hint for granting conditional access!
Property | Value |
---|---|
IAM Roles for Username 2 | Replace Viewer with Browser, and keep BigQuery Data Viewer to add a condition. |
Condition title | No SPII Access Only |
Condition type 1 and operator | Select tag and has value |
Value path for condition type 1 |
Unlike the car_owners dataset, the orders dataset does not contain SPII, but instead contains details on orders only.
Optional testing: If you would like to see this conditional access in action, you can log into the project as Username 2, and go to BigQuery. Refresh the page until the dataset named orders is the only dataset remaining in the Explorer list because Username 2 now only has access to datasets tagged with No for SPII.
Note that it may take a few minutes for the condition to be applied.
Click Check my progress to verify the objective.
Your team already has a Python function that identifies and redacts or blocks sensitive data types in Gen AI model responses. You have been asked to expand the function to block Gen AI model responses that contain US Vehicle Identification Numbers, which are sensitive data consisting of a unique 17-digit code assigned to every on-road motor vehicle in North America.
To help you achieve this goal, complete the following subtasks using the notebook provided in this lab environment:
Is 4Y1SL65848Z411439 an example of a US Vehicle Identification Number (VIN)?
Be sure to use the pre-created notebook named deidentify-model-response-challenge-lab.ipynb in the workbench instance named vertex-ai-jupyterlab.
Helpful hint for updating and testing the Python function!
Click Check my progress to verify the objective.
In this lab, you created and scheduled a discovery scan configuration for Cloud Storage, and then you created a de-identify template and used it to run a de-identify job on Cloud Storage files. You also created IAM tags and applied them to BigQuery data to grant conditional access. Last, you updated a Python function to redact and block Gen AI model responses containing sensitive data as identified by the Cloud Data Loss Prevention (DLP) API.
...helps you make the most of Google Cloud technologies. Our classes include technical skills and best practices to help you get up to speed quickly and continue your learning journey. We offer fundamental to advanced level training, with on-demand, live, and virtual options to suit your busy schedule. Certifications help you validate and prove your skill and expertise in Google Cloud technologies.
Manual Last Updated March 28, 2025
Lab Last Tested March 28, 2025
Copyright 2025 Google LLC All rights reserved. Google and the Google logo are trademarks of Google LLC. All other company and product names may be trademarks of the respective companies with which they are associated.
This content is not currently available
We will notify you via email when it becomes available
Great!
We will contact you via email if it becomes available
One lab at a time
Confirm to end all existing labs and start this one