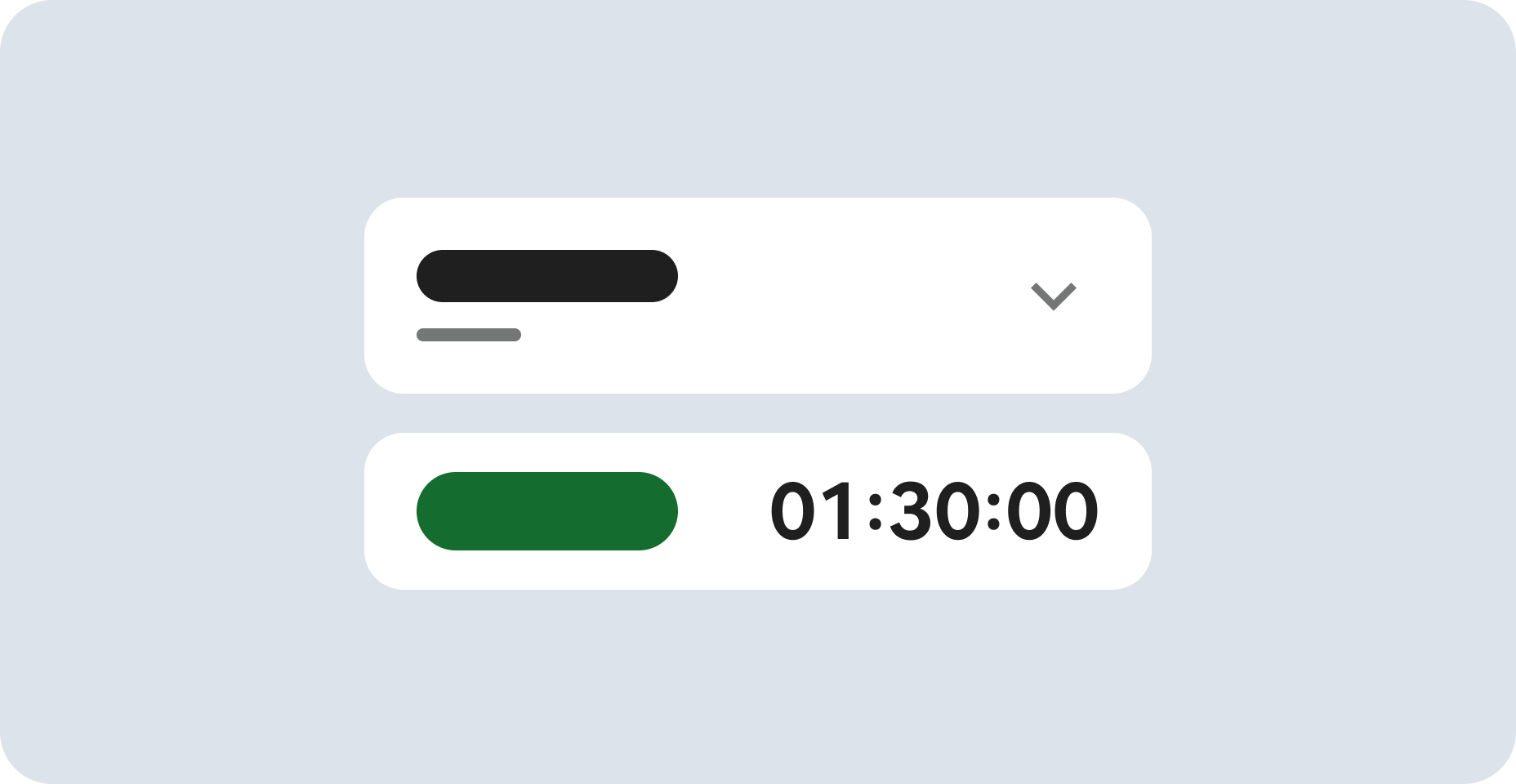
Before you begin
- Labs create a Google Cloud project and resources for a fixed time
- Labs have a time limit and no pause feature. If you end the lab, you'll have to restart from the beginning.
- On the top left of your screen, click Start lab to begin
Enable relevant APIs.
/ 5
Application is developed with Story tab.
/ 5
Application marketing campaign tab is developed.
/ 5
Application image playground with furniture recommendation tab is developed.
/ 5
Image playground with oven instructions tab is developed.
/ 10
Image playground with ER diagrams tab is developed
/ 10
Image playground with math reasoning tab is developed.
/ 10
Application video playground with video description tab is developed.
/ 10
Video playground with video tags tab is developed.
/ 10
Video playground with video highlights tab is developed.
/ 10
Video playground with video geolocation tab is developed.
/ 10
Application is deployed to Cloud Run.
/ 10
Gemini is a family of generative AI models that is designed for multimodal use cases. It comes in three sizes: Ultra, Pro and Nano. Gemini 1.0 Pro is available for developers and enterprises to build for your own use cases. Gemini 1.0 Pro accepts text as input and generates text as output. There is also a dedicated Gemini 1.0 Pro Vision multimodal endpoint that accepts text and imagery as input, and generates text as output. SDKs are available to help you build apps in Python, Android (Kotlin), Node.js, Swift and JavaScript.
On Google Cloud, the Vertex AI Gemini API provides a unified interface for interacting with Gemini models. The API supports multimodal prompts as input and output text or code. There are currently two models available in the Gemini API:
Gemini 1.0 Pro model (gemini-pro): Designed to handle natural language tasks, multiturn text and code chat, and code generation.
Gemini 1.0 Pro Vision model (gemini-pro-vision): Supports multimodal prompts. You can include text, images, and video in your prompt requests and get text or code responses.
Vertex AI is a machine learning (ML) platform that lets you train and deploy ML models and AI applications, and customize large language models (LLMs) for use in your AI-powered applications. Vertex AI allows for customization of Gemini with full data control and benefits from additional Google Cloud features for enterprise security, safety, privacy and data governance and compliance. To learn more about Vertex AI, view the link in the Next Steps section at the end of the lab.
In this lab, you use the Vertex AI SDK for Python to call the Vertex AI Gemini API.
In this lab, you learn how to perform the following tasks:
For each lab, you get a new Google Cloud project and set of resources for a fixed time at no cost.
Sign in to Qwiklabs using an incognito window.
Note the lab's access time (for example, 1:15:00
), and make sure you can finish within that time.
There is no pause feature. You can restart if needed, but you have to start at the beginning.
When ready, click Start lab.
Note your lab credentials (Username and Password). You will use them to sign in to the Google Cloud Console.
Click Open Google Console.
Click Use another account and copy/paste credentials for this lab into the prompts.
If you use other credentials, you'll receive errors or incur charges.
Accept the terms and skip the recovery resource page.
Cloud Shell is a virtual machine that contains development tools. It offers a persistent 5-GB home directory and runs on Google Cloud. Cloud Shell provides command-line access to your Google Cloud resources. gcloud
is the command-line tool for Google Cloud. It comes pre-installed on Cloud Shell and supports tab completion.
Click the Activate Cloud Shell button () at the top right of the console.
Click Continue.
It takes a few moments to provision and connect to the environment. When you are connected, you are also authenticated, and the project is set to your PROJECT_ID.
(Output)
(Example output)
(Output)
(Example output)
Sign in to the Google Cloud console with your lab credentials, and open the Cloud Shell terminal window.
To set your project ID and region environment variables, in Cloud Shell, run the following commands:
In order to use various Google Cloud services in this lab, you must enable a few APIs:
To verify the objective, click Check my progress.
In this task, you set up a Python virtual environment, and install the application dependencies.
To confirm that Cloud Shell is authorized, in Cloud Shell, run the following command:
If you're asked to authorize Cloud Shell, click Authorize.
To create the app directory, run the following command:
Change to the ~/gemini-app
directory:
The application files are created in the ~/gemini-app
directory. This directory will contain the Python application source files, dependencies, and a Docker file which we will use later in this lab.
Create a virtual environment on top of the existing Python installation, so that any packages installed in this environment are isolated from the packages in the base environment. When used from within a virtual environment, installation tools such as pip
will install Python packages into the virtual environment.
To create the Python virtual environment, from within the gemini-app
folder, run the command:
Activate the Python virtual environment:
A Python requirements file is a simple text file that lists the dependencies required by your project. To start, there are three modules we need in our requirements file.
Our app is written using Streamlit, an open-source Python library that is used to create web apps for machine learning and data science. The app uses the Vertex AI SDK for Python library to interact with the Gemini API and models. Cloud Logging is used to log information from our application.
To create the requirements file, run the following command:
Install the application dependencies:
pip is the package installer for Python.
Wait until all the packages are installed before continuing to the next task.
The app source code will be written in mutliple .py source files. Let's start with the main entry point in app.py
.
To create the app.py
entry point code, run the following command:
View the contents of the app.py
file:
The app uses streamlit
to create a number of tabs in the UI. In this initial version of the app, we build the first tab Story that contains functionality to generate a story, and then incrementally build the other tabs in subsequent tasks in the lab.
The app first initializes the Vertex AI SDK
passing in the values of the PROJECT_ID, and REGION environment variables.
It then loads the gemini-pro
, and gemini-pro-vision
models using the GenerativeModel
class that represents a Gemini model. This class includes methods to help generate content from text, images, and video.
The app creates 4 tabs in the UI named Story, Marketing Campaign, Image Playground, and Video Playground.
The app code then invokes the render_tab1()
function to create the UI for the Story tab in the app's UI.
To write code that renders the Story tab in the app's UI, run the following command:
View the contents of the app_tab1.py
file:
The render_story_tab
function generates the UI controls in the tab by invoking functions to render the text input fields, and other options.
The generate_prompt
function generates the text prompt that is supplied to the Gemini API. The prompt string is created by concatenating user entered values in the tab UI for the character of the story, and options such as the story length (short, long), creativity level (low, high), and the story premise.
The function also returns a temperature
value based on the selected creativity level of the story. This value is supplied as the temperature
configuration parameter to the model, which controls the randomness of the model's predictions. The max_output_tokens
configuration parameter specifies the maximum number of output tokens to generate per message.
To generate the model response, a button is created in the tab UI. When the button is clicked, the get_gemini_pro_text_response
function is invoked, which we will code in the next step in the lab.
The response_utils.py
file contains functions to generate the model's responses.
To write code to generate the model's text response, run the following command:
View the contents of the response_utils.py
file:
The get_gemini_pro_text_response
function uses the GenerativeModel
and some of the other classes from the vertexai.preview.generative_models
package in the Vertex AI SDK for Python. From the generate_content
method of the class, a response is generated using the text prompt that is passed to the method.
A safety_settings
object is also passed to this method to control the model response by blocking unsafe content. The sample code in this lab uses safety setting values that instructs the model to always return content regardless of the probability of the content being unsafe. You can assess the content generated, and then adjust these settings if your application requires more restrictive configuration. To learn more, view the safety settings documentation.
In this task, you run the app locally using streamlit
, and test the app functionality.
To run the app locally, in Cloud Shell, execute the command:
The app starts and you are provided a URL to access the app.
To launch the app home page in your browser, click web preview in the Cloud Shell menubar, and then click Preview on port 8080.
You can also copy and paste the app URL in a separate browser tab to access the app.
Generate a story by providing your input, view the prompt, and view the response generated by the Gemini 1.0 Pro model.
To generate a story, in the Story tab, leave the default settings, and then click Generate my story.
Wait for the response to be generated, and then click the Story response tab.
To view the prompt that was used to generate the response, click the Prompt tab.
In the Cloud Shell window, end the app and return to the command prompt by pressing control-c.
To verify the objective, click Check my progress.
In this task, you use the Gemini 1.0 Pro text model to generate a marketing campaign for a company. You develop the code that generates a second tab in your app.
To write code that renders the Marketing Campaign tab in the app's UI, run the following command:
To add tab2 to the app, run the following command:
Generate a marketing campaign by providing your input, view the prompt, and view the response generated by the Gemini 1.0 Pro model.
To run the app locally, in Cloud Shell, execute the command:
The app starts and you are provided a URL to access the app.
To launch the app home page in your browser, click web preview in the Cloud Shell menubar, and then click Preview on port 8080.
To generate a marketing campaign, in the Marketing campaign tab, leave the default settings, and then click Generate campaign.
Wait for the response to be generated, and then click the Campaign response tab.
To view the prompt that was used to generate the response, click the Prompt tab.
Repeat the steps above to generate marketing campaigns with different values of the parameters such as the product category, target audience, location, and campaign goals.
In the Cloud Shell window, end the app and return to the command prompt by pressing control+c.
To verify the objective, click Check my progress.
In this task, you use the Gemini 1.0 Pro vision model to process images and receive recommendations and information from the images that are supplied to the model.
In this subtask, you implement the code for the Image Playground tab, and the code to interact with the model to generate recommendations from an image.
To write code that renders the Image Playground tab in the app's UI, run the following command:
View the contents of the app_tab3.py
file:
The render_image_playground_tab
function builds the UI that enables the app user to interact with the Gemini 1.0 Pro Vision model. It creates a set of tabs: "Furniture recommendation", "Oven instructions", "ER diagrams", "Math reasoning" in the UI. You write the code for the remaining tabs in subsequent tasks in this lab.
In the Furniture recommendation
tab, a living room scene is used to perform visual understanding. Along with a set of additional images of chairs, the code invokes the Gemini 1.0 Pro Vision multimodal API endpoint to get a recommendation of a chair that complements the living room scene.
The code uses more than one text prompt and the images of the living room and chairs, and provides that in a list to the model. The Part
class is used to obtain the image from the multi-part content URI that is hosted in a Cloud Storage bucket. The prompt also specifies a tabular format for the model output, and to include the rationale for the recommendation.
The response_utils.py
file contains functions to generate the model's responses.
Update the file to add code that generates the model's multimodal response:
To add tab3 to the app, run the following command:
Run the app using the command provided in previous steps in the lab.
To launch the app home page in your browser, click web preview in the Cloud Shell menubar, and then click Preview on port 8080.
Click Image Playground, and then click Furniture recommendation.
The tab displays the images of the living room, and chairs.
Click Generate recommendation.
View the response from the Gemini 1.0 Pro vision model.
The response is in tabular format as requested in the prompt. The model recommends two of the four chairs, and provides the rationale for the recommendation.
In the Cloud Shell window, end the app and return to the command prompt by pressing control+c.
To verify the objective, click Check my progress.
In this task, you use the Gemini 1.0 Pro Vision model to extract information from an image after analyzing it's layout of icons and text.
Equipped with the ability to extract information from visual elements on screens, Gemini can analyze screenshots, icons, and layouts to provide a holistic understanding of the depicted scene. In this task, you provide an image of a kitchen oven's control panel to the model, and then prompt the model to generate instructions for a specific function.
To implement code for the Oven instructions tab in the Image Playground tab in the app's UI, run the following command:
The code above builds the UI of the Oven instructions tab. An image of a kitchen oven's control panel is used along with text to prompt the model to generate instructions for a specific function that is available on the panel, in this case, resetting the clock.
Run the app using the command provided in previous steps in the lab.
To launch the app home page in your browser, click web preview in the Cloud Shell menubar, and then click Preview on port 8080.
Click Image Playground, and then click Oven instructions.
The tab displays an image of the oven control panel.
Click Generate instructions.
View the response from the Gemini 1.0 Pro Vision model.
The response contains the steps that can be used to reset the clock on the oven's control panel. It also includes instructions that indicate where to locate the button on the panel, showcasing the model's ability to analyze the layout of the panel in the image.
In the Cloud Shell window, end the app and return to the command prompt by pressing control+c.
To verify the objective, click Check my progress.
Gemini's multimodal capabilities enable it to comprehend diagrams and take actionable steps, such as document or code generation. In this task, you use the Gemini 1.0 Pro Vision model to analyze an Entity-Relationship (ER) diagram and generate documentation on the entities and relationships found in the diagram.
In this task, you provide an image of an ER diagram to the model, and then prompt the model to generate documentation.
To implement code for the ER diagrams tab in the Image Playground tab in the app's UI, run the following command:
The code above builds the UI of the ER diagrams tab. An image of an ER diagram is used along with text to prompt the model to generate documentation about the entities and relationships found in the diagram.
Run the app using the command provided in previous steps in the lab.
To launch the app home page in your browser, click web preview in the Cloud Shell menubar, and then click Preview on port 8080.
Click Image Playground, and then click ER diagrams.
The tab displays the ER diagram image.
Click Generate documentation.
View the response from the Gemini 1.0 Pro Vision model.
The response contains the list of entities and their relationships found in the diagram.
In the Cloud Shell window, end the app and return to the command prompt by pressing control+c.
To verify the objective, click Check my progress.
Gemini 1.0 Pro Vision can also recognize math formulas and equations and extract specific information from them. This capability is particularly useful for generating explanations for math problems.
In this task, you use the Gemini 1.0 Pro Vision model to extract and interpret a math formula from an image.
To implement code for the Math reasoning tab in the Image Playground tab in the app's UI, run the following command:
The code above builds the UI of the Math reasoning tab. An image of a math equation is used along with text to prompt the model to generate answers and other characteristics about the equation.
Run the app using the command provided in previous steps in the lab.
To launch the app home page in your browser, click web preview in the Cloud Shell menubar, and then click Preview on port 8080.
Click Image Playground, and then click Math reasoning.
The tab displays the image containing the math equation.
Click Generate answers.
View the response from the Gemini 1.0 Pro Vision model.
The response contains the answers to the questions supplied in the prompt to the model.
In the Cloud Shell window, end the app and return to the command prompt by pressing control+c.
To verify the objective, click Check my progress.
In this task, you use the Gemini 1.0 Pro vision model to process videos and generate tags and information from the videos that are supplied to the model.
The Gemini 1.0 Pro Vision model can also provide the description of what is going on in a video. In this subtask, you implement the code for the Video Playground tab, and the code to interact with the model to generate the description of a video.
To write code that renders the Video Playground tab in the app's UI, run the following command:
View the contents of the app_tab4.py
file:
The render_video_playground_tab
function builds the UI that enables the app user to interact with the Gemini 1.0 Pro Vision model. It creates a set of tabs: "Video description", "Video tags", "Video highlights", "Video geolocation" in the UI. You write the code for the remaining tabs in subsequent tasks in this lab.
The Video description
tab uses a prompt along with the video to generate a description of the video, and to identify other places that look similar to the place in the video.
To add tab4 to the app, run the following command:
Run the app using the command provided in previous steps in the lab.
To launch the app home page in your browser, click web preview in the Cloud Shell menubar, and then click Preview on port 8080.
Click Video Playground, and then click Video description.
The tab displays the video of a place. Click to play the video.
Click Generate video description.
View the response from the Gemini 1.0 Pro Vision model.
The response contains a description of the place, and 5 other places that look similar.
In the Cloud Shell window, end the app and return to the command prompt by pressing control+c.
To verify the objective, click Check my progress.
In this task, you use the Gemini 1.0 Pro Vision model to generate tags from a video.
To implement code for the Video tags tab in the Video Playground tab in the app's UI, run the following command:
The code above builds the UI of the Video tags tab. A video is used along with text to prompt the model to generate tags and answer questions about scenes in the video.
Run the app using the command provided in previous steps in the lab.
To launch the app home page in your browser, click web preview in the Cloud Shell menubar, and then click Preview on port 8080.
Click Video Playground, and then click Video tags.
The tab displays the video that will be used to prompt the model. Click to play the video.
Click Generate video tags.
View the response from the Gemini 1.0 Pro Vision model.
The response contains the answers to the questions that were provided in the prompt to the model. The questions and answers are output in tabular format and include 5 tags as requested.
In the Cloud Shell window, end the app and return to the command prompt by pressing control+c.
To verify the objective, click Check my progress.
In this task, you use the Gemini 1.0 Pro Vision model to generate highlights from a video that include information about the objects, people, and context shown in the video.
To implement code for the Video highlights tab in the Video Playground tab in the app's UI, run the following command:
The code above builds the UI of the Video highlights tab. A video is used along with text to prompt the model to generate highlights from the video.
Run the app using the command provided in previous steps in the lab.
To launch the app home page in your browser, click web preview in the Cloud Shell menubar, and then click Preview on port 8080.
Click Video Playground, and then click Video highlights.
The tab displays the video that will be used to prompt the model. Click to play the video.
Click Generate video highlights.
View the response from the Gemini 1.0 Pro Vision model.
The response contains the answers to the questions that were provided in the prompt to the model. The questions and answers are output in tabular format and list features from the video such as the girl's profession, the features of the phone that are used. The response also contains a summary description of the scenes in the video.
In the Cloud Shell window, end the app and return to the command prompt by pressing control+c.
To verify the objective, click Check my progress.
In this task, you use the Gemini 1.0 Pro Vision model to determine the location where the scene in the video takes place.
To implement code for the Video geolocation tab in the Video Playground tab in the app's UI, run the following command:
The code above builds the UI of the Video geolocation tab. A video is used along with text to prompt the model to answer questions about the video that include location information about entities seen in the video.
Run the app using the command provided in previous steps in the lab.
To launch the app home page in your browser, click web preview in the Cloud Shell menubar, and then click Preview on port 8080.
Click Video Playground, and then click Video geolocation.
The tab displays the video that will be used to prompt the model. Click to play the video.
Click Generate.
View the response from the Gemini 1.0 Pro Vision model.
The response contains the answers to the questions that were provided in the prompt to the model. The questions and answers are output in tabular format and include the location information as requested.
In the Cloud Shell window, end the app and return to the command prompt by pressing control+c.
To verify the objective, click Check my progress.
Now that you've tested the app locally, you can make it available to others by deploying the app to Cloud Run on Google Cloud. Cloud Run is a managed compute platform that lets you run application containers on top of Google's scalable infrastructure.
Make sure you are in the app
directory:
Verify that the PROJECT_ID, and REGION environment variables are set:
If these environment variables are not set, then run the command to set them:
Set environment variables for your service and artifact repository:
To create the repository in Artifact Registry, run the command:
Set up authentication to the repository:
We'll use a Dockerfile
to build the container image for our application. A Dockerfile
is a text document that contains all the commands that a user could call on the command line to assemble a container image. It is used with Docker, a container platform that builds and runs container images.
To create a Dockerfile
, run the command:
To build the container image for your app, run the command:
Cloud Build is a service that executes builds based on your specifications on Google Cloud, and produces artifacts such as Docker containers or Java archives.
Wait until the command finishes before advancing to the next step.
The final task is to deploy the service to Cloud Run with the image that was built and pushed to the repository in Artifact Registry.
To deploy your app to Cloud Run, run the command:
After the service is deployed, a URL to the service is generated in the output of the previous command. To test your app on Cloud Run, navigate to that URL in a separate browser tab or window.
Choose the app functionality that you want to test. The app will prompt the Vertex AI Gemini API to generate and display the responses.
To verify the objective, click Check my progress.
When you have completed your lab, click End Lab. Qwiklabs removes the resources you’ve used and cleans the account for you.
You will be given an opportunity to rate the lab experience. Select the applicable number of stars, type a comment, and then click Submit.
The number of stars indicates the following:
You can close the dialog box if you don't want to provide feedback.
For feedback, suggestions, or corrections, please use the Support tab.
In this lab you:
Copyright 2023 Google LLC All rights reserved. Google and the Google logo are trademarks of Google LLC. All other company and product names may be trademarks of the respective companies with which they are associated.
Ce contenu n'est pas disponible pour le moment
Nous vous préviendrons par e-mail lorsqu'il sera disponible
Parfait !
Nous vous contacterons par e-mail s'il devient disponible
One lab at a time
Confirm to end all existing labs and start this one