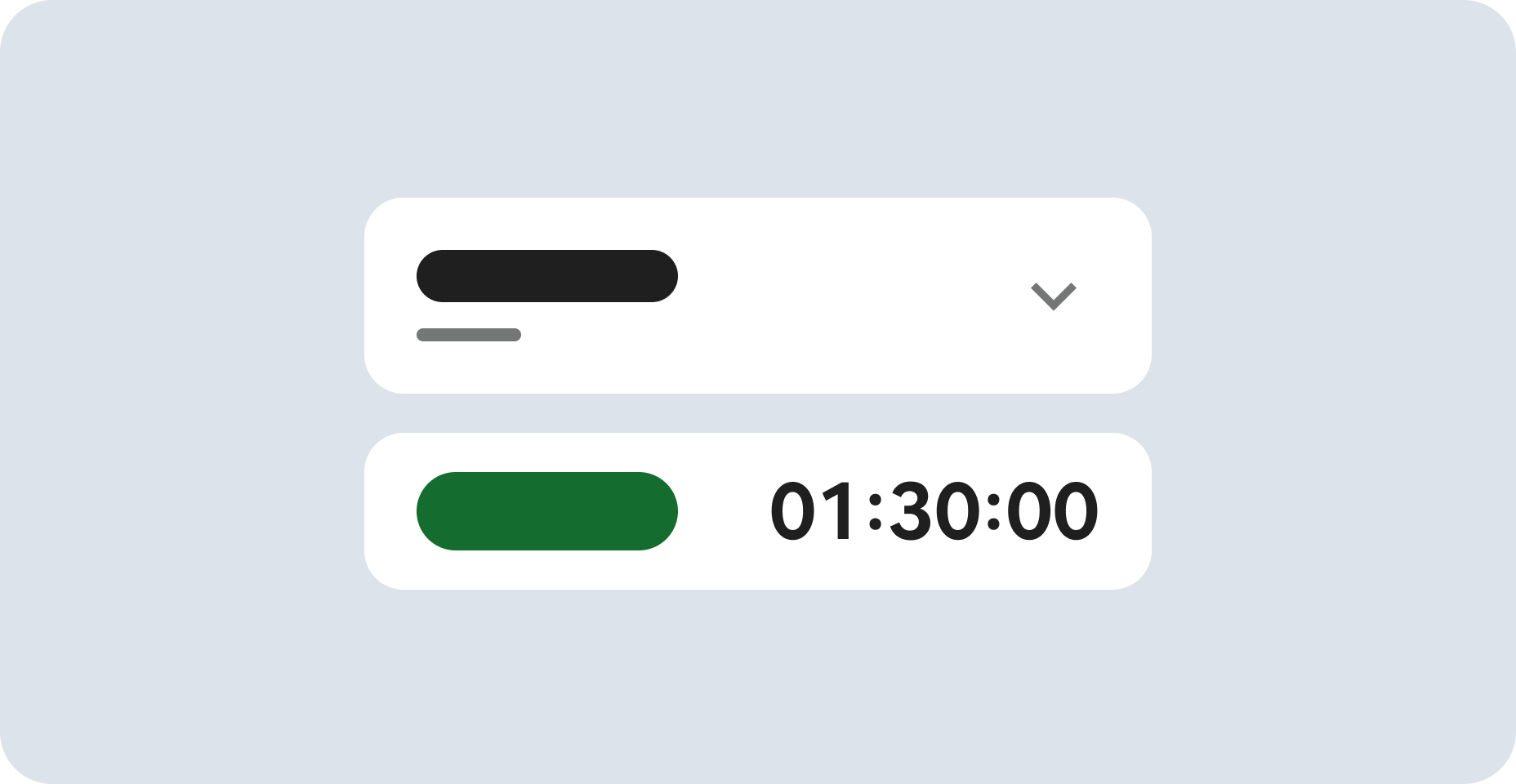
Before you begin
- Labs create a Google Cloud project and resources for a fixed time
- Labs have a time limit and no pause feature. If you end the lab, you'll have to restart from the beginning.
- On the top left of your screen, click Start lab to begin
Create a dataset
/ 25
Load Data to BigQuery Tables
/ 25
Explore and Investigate the Data with BigQuery
/ 10
Prepare Your Data
/ 10
Train an Unsupervised Model to Detect Anomalies
/ 10
Train a Supervised Machine Learning Model
/ 10
Predict Fraudulent Transactions on Test Data
/ 10
In this lab you will explore the financial transactions data for fraud analysis, apply feature engineering and machine learning techniques to detect fraudulent activities using BigQuery ML.
Public financial transactions data will be used. The data contains the following columns:
isfraud
flagThe target column isfraud includes the labels for the fraudulent transactions. Using these labels you will train supervised models for fraud detection and apply unsupervised models to detect anomalies.
The data for this lab is from the Kaggle site. If you do not have a Kaggle account, it's free to create one.
What you'll learn:
In this lab, you will use the BigQuery interface for feature engineering, model development, evaluation and prediction.
Participants that prefer Notebooks as the model development interface may choose to build models in AI Platform Notebooks instead of BigQuery ML. Then at the end of the lab, you can also complete the optional section. You can import open source libraries and create custom models or you can call BigQuery ML models within Notebooks using BigQuery magic commands.
If you want to train models in an automated way without any coding, you can use Google Cloud AutoML which builds models using state-of-the-art algorithms. The training process for AutoML would take almost 2 hours, that's why it is recommended to initiate it at the beginning of the lab, as soon as the data is prepared, so that you can see the results at the end. Check for the "Attention" phrase at the end of the data preparation step.
Read these instructions. Labs are timed and you cannot pause them. The timer, which starts when you click Start Lab, shows how long Google Cloud resources are made available to you.
This hands-on lab lets you do the lab activities in a real cloud environment, not in a simulation or demo environment. It does so by giving you new, temporary credentials you use to sign in and access Google Cloud for the duration of the lab.
To complete this lab, you need:
Click the Start Lab button. If you need to pay for the lab, a dialog opens for you to select your payment method. On the left is the Lab Details pane with the following:
Click Open Google Cloud console (or right-click and select Open Link in Incognito Window if you are running the Chrome browser).
The lab spins up resources, and then opens another tab that shows the Sign in page.
Tip: Arrange the tabs in separate windows, side-by-side.
If necessary, copy the Username below and paste it into the Sign in dialog.
You can also find the Username in the Lab Details pane.
Click Next.
Copy the Password below and paste it into the Welcome dialog.
You can also find the Password in the Lab Details pane.
Click Next.
Click through the subsequent pages:
After a few moments, the Google Cloud console opens in this tab.
Cloud Shell is a virtual machine that is loaded with development tools. It offers a persistent 5GB home directory and runs on the Google Cloud. Cloud Shell provides command-line access to your Google Cloud resources.
Click Activate Cloud Shell at the top of the Google Cloud console.
Click through the following windows:
When you are connected, you are already authenticated, and the project is set to your Project_ID,
gcloud
is the command-line tool for Google Cloud. It comes pre-installed on Cloud Shell and supports tab-completion.
Output:
Output:
gcloud
, in Google Cloud, refer to the gcloud CLI overview guide.
unzip
command:You will see that 1 file has been inflated.
finance
in Cloud Shell:Successful execution of the above command will result with the output:
Click Check my progress to verify the objective.
To load your data into BigQuery you can either use the BigQuery user interface or the command terminal in Cloud Shell. Choose one of the options below to load your data.
finance.fraud_data
by executing the following command:The option --autodetect
will read the schema of the table (the variable names, types, etc.) automatically.
You can load data from your Cloud Storage bucket by opening BigQuery in the Cloud Console.
In the Create table pop-up window, set Source
as Google Cloud Storage and select the raw csv file in your Cloud Storage bucket.
Enter table name as fraud_data and select the Auto detect option under Schema so that the variable names will be read from the first line of the raw file automatically.
Click Create table.
The loading process can take one or two minutes.
Click Check my progress to verify the objective.
If you haven't opened BigQuery in the Cloud Console, yet, do it now.
Next you'll start exploring the data in order to understand it better and prepare it for machine learning models.
Add the following queries to the query EDITOR, then click RUN, then explore the data.
Click (+) SQL query to start the next query. This will let you compare results easily when you're done.
Look in the isFraud
column for 1 = yes.
isFraud
):PAUSE and REFLECT:
isfraud
number by the total number of observations you get the proportion of fraudulent transactions.In the next section, you will see how to handle these questions and improve the data for machine learning models.
Click Check my progress to verify the objective.
You can improve the modelling data by adding new features, filtering unnecessary transaction types, and increasing the proportion of the target variable isFraud
by applying undersampling.
Based on your findings from the analysis phase, you only need to analyse the transaction types "TRANSFER"
and "CASH_OUT"
and filter the rest. You can also compute new variables from the existing amount values.
The dataset contains an extremely unbalanced target for fraud (fraud rate in the raw data = 0.0013%). Having rare events is common for fraud. In order to make the pattern of fraudulent behaviour more obvious for the machine learning algorithms, and also make it easy to interpret the results, stratify the data and increase the proportion of the fraudulent flags.
Run the query.
Create a TEST data table by selecting a random sample of 20%:
This data will be kept separate and not be included in training. You will use it for scoring the model at the final stage.
BigQuery ML and AutoML will automatically partition the model data as TRAIN and VALIDATE while using the machine learning algorithms in order to test the error rate on both the training and validation data and avoid overfitting.
The sample data that you created for modelling contains approximately 228k rows of banking transactions.
You can also manually partition your data set as TRAIN/VALIDATE and TEST, especially when you want to compare models from different environments such as AutoML or AI Platform and have consistency.
PAUSE and REFLECT:
Click Check my progress to verify the objective.
Unsupervised methods are commonly used in fraud detection to explore the abnormal behaviour in the data. It also helps when there are no labels for fraud or the event rate is very low and the number of occurrences does not allow you to build a supervised model.
In this section, you will use k-means clustering algorithm to create segments of transactions, analyse each segment and detect the ones with anomalies.
kmeans
:This will create a k-means model called model_unsupervised
with 5 clusters using the selected variables from fraud_data_model
.
Once the model finishes training you will see it appear under Finance > Models.
The k-means algorithm creates an output variable called centroid_id. Each transaction is assigned to a centroid_id. The transactions that are similar/closer to each other are assigned to the same cluster by the algorithm.
The Davies-Bouldin index shows an indication of how homogeneous the clusters are. The lower the value is, the more distant the clusters are from each other which is the desired outcome.
The numeric features are displayed with bar charts for each centroid (cluster) in the Evaluation tab. The numbers next to the bars show the average value of the variables within each cluster. As a best practice, the input variables can be standardized or grouped into buckets in order to avoid the impact of large numbers or outliers in the distance calculations for clustering. For the sake of simplicity, this lab uses the original variables in this exercise.
The categorical variables that are used as input are displayed separately. You can see the distribution of TRANSFER and CASH_OUT transactions in each segment below.
The charts might look different for your model, focus on the smaller segments and try to interpret the distributions.
The target variable isFraud
hasn't been used in this unsupervised model. In this exercise, it is preferred to save that variable for profiling and use it to explore the distribution of fraudulent activities within each cluster.
Score the test data (fraud_data_test
) using this model and see the number of fraud events in each centroid_id
. The clustering algorithms create homogeneous groups of observations. In this query, ML.PREDICT
will call the model and generate the centroid_id
for each transaction in the test data.
Run the following code in new query:
PAUSE and REFLECT:
Click Check my progress to verify the objective.
Now you are ready to start building supervised models using BigQuery ML to predict the likelihood of having fraudulent transactions. Start with a simple model - use BigQuery ML to create a binary logistic regression model for classification. This model will attempt to predict if the transaction is likely to be fraudulent or not.
For all non-numeric (categorical) variables, BigQuery ML automatically performs a one-hot encoding transformation. This transformation generates a separate feature for each unique value in the variable. In this exercise, one-hot encoding will be performed for the variable TYPE automatically by BigQuery ML.
You will see model_supervised_initial
table added under Finance > Models when it is ready.
Once the model is created you can get the model metadata, training, and evaluation stats from BigQuery Console UI.
On the Evaluation tab, you will find various performance metrics specific to the classification model.
Understanding performance of the model is a key topic in machine learning. Since you performed a logistic regression for classification, the following key concepts are useful to understand:
The chart in this wikipedia page explains the concepts of precision and recall nicely.
The ROC value for this regression model is very high. You can get a better understanding of the accuracy by testing the outcomes for different probability thresholds.
Now, look at the most influential features in the model.
The weights are standardized to eliminate the impact of the scale of the variables using the standardize option. The larger weights are the more important ones. The sign of the weight indicates the direction, depending on the direct or inverse relationship with the target.
PAUSE and REFLECT:
oldbalanceOrig
and type
are the most important variables.Click Check my progress to verify the objective.
Now do a fun exercise - create a new model and train the two models to get better accuracy.
Next, you will compare the 2 models you created and choose the best one.
Improve the existing logistic regression model by adding new variables.
After creating the model, you can evaluate the performance of the classifier using ML.EVALUATE
function. The ML.EVALUATE
function evaluates the outcome or predicted values against actual data.
PAUSE and REFLECT:
The last step in machine learning is to use the champion model to predict the outcome on new datasets.
The machine learning algorithms in BQML create a nested variable called predicted_<target_name\>_probs
. This variable includes the probability scores for the model decision. The decision for your model is either being fraudulent or genuine.
PAUSE and REFLECT:
Click Check my progress to verify the objective.
...helps you make the most of Google Cloud technologies. Our classes include technical skills and best practices to help you get up to speed quickly and continue your learning journey. We offer fundamental to advanced level training, with on-demand, live, and virtual options to suit your busy schedule. Certifications help you validate and prove your skill and expertise in Google Cloud technologies.
Manual Last Updated December 27, 2024
Lab Last Tested December 27, 2024
Copyright 2025 Google LLC All rights reserved. Google and the Google logo are trademarks of Google LLC. All other company and product names may be trademarks of the respective companies with which they are associated.
This content is not currently available
We will notify you via email when it becomes available
Great!
We will contact you via email if it becomes available
One lab at a time
Confirm to end all existing labs and start this one