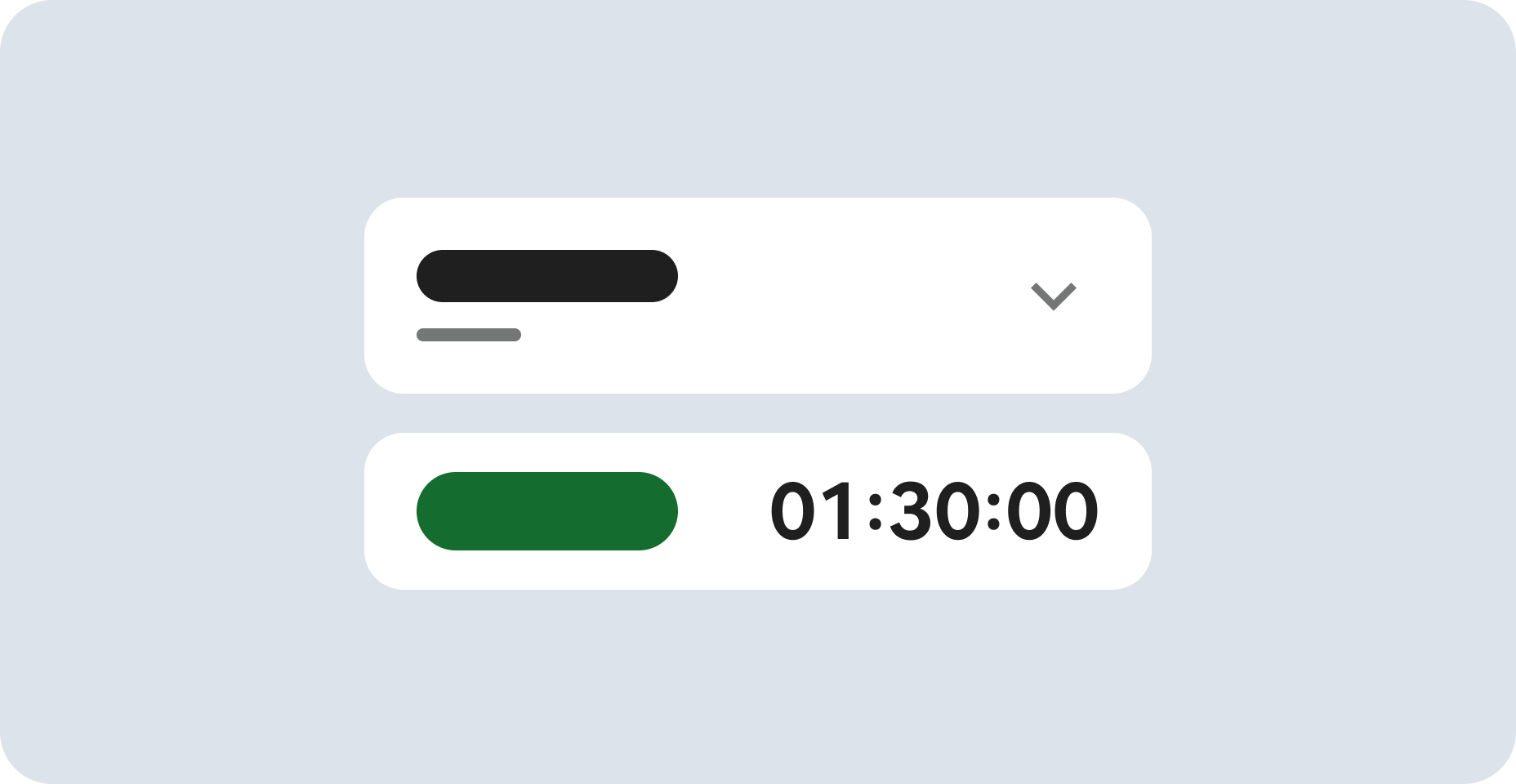
Before you begin
- Labs create a Google Cloud project and resources for a fixed time
- Labs have a time limit and no pause feature. If you end the lab, you'll have to restart from the beginning.
- On the top left of your screen, click Start lab to begin
Enable Google Cloud APIs
/ 20
Open a Vertex AI Workbench instance
/ 20
Run the lab notebook
/ 60
In this lab, you use BigQuery for data processing and exploratory data analysis and the Vertex AI platform to train and deploy a custom TensorFlow Regressor model to predict customer lifetime value. The goal of the lab is to introduce to Vertex AI through a high value real world use case - predictive CLV. You start with a local BigQuery and TensorFlow workflow that you may already be familiar with and progress toward training and deploying your model in the cloud with Vertex AI.
In this lab, you will:
Read these instructions. Labs are timed and you cannot pause them. The timer, which starts when you click Start Lab, shows how long Google Cloud resources are made available to you.
This hands-on lab lets you do the lab activities in a real cloud environment, not in a simulation or demo environment. It does so by giving you new, temporary credentials you use to sign in and access Google Cloud for the duration of the lab.
To complete this lab, you need:
Click the Start Lab button. If you need to pay for the lab, a dialog opens for you to select your payment method. On the left is the Lab Details pane with the following:
Click Open Google Cloud console (or right-click and select Open Link in Incognito Window if you are running the Chrome browser).
The lab spins up resources, and then opens another tab that shows the Sign in page.
Tip: Arrange the tabs in separate windows, side-by-side.
If necessary, copy the Username below and paste it into the Sign in dialog.
You can also find the Username in the Lab Details pane.
Click Next.
Copy the Password below and paste it into the Welcome dialog.
You can also find the Password in the Lab Details pane.
Click Next.
Click through the subsequent pages:
After a few moments, the Google Cloud console opens in this tab.
Cloud Shell is a virtual machine that is loaded with development tools. It offers a persistent 5GB home directory and runs on the Google Cloud. Cloud Shell provides command-line access to your Google Cloud resources.
Click Activate Cloud Shell at the top of the Google Cloud console.
Click through the following windows:
When you are connected, you are already authenticated, and the project is set to your Project_ID,
gcloud
is the command-line tool for Google Cloud. It comes pre-installed on Cloud Shell and supports tab-completion.
Output:
Output:
gcloud
, in Google Cloud, refer to the gcloud CLI overview guide.
Open a new Cloud Shell terminal by clicking the Cloud Shell icon in the top right corner of the Google Cloud Console.
In your Cloud Shell terminal, use gcloud
to enable the services used in the lab:
Click Check my progress to verify the objective.
In the Google Cloud console, from the Navigation menu (), select Vertex AI.
Click Enable All Recommended APIs.
On the left-hand side, click Workbench.
At the top of the Workbench page, ensure you are in the Instances view.
Click Open JupyterLab next to the instance name to launch the JupyterLab interface. This will open a new tab in your browser.
Your terminal window will open in a new tab. You can now run commands in the terminal to interact with your Workbench instance.
Click Check my progress to verify the objective.
y
and press Enter to confirm the installation.In the file browser, open
When prompted, select the Python 3 kernel.
Continue the lab in the notebook, and run each cell by clicking the Run icon at the top of the screen.
Alternatively, you can execute the code in a cell with SHIFT + ENTER.
Read the narrative and make sure you understand what's happening in each cell.
Click Check my progress to verify the objective.
In this lab, you ran a machine learning experimentation workflow using Google Cloud BigQuery for data storage and analysis and Vertex AI machine learning services to train and deploy a TensorFlow model to predict customer lifetime value.
...helps you make the most of Google Cloud technologies. Our classes include technical skills and best practices to help you get up to speed quickly and continue your learning journey. We offer fundamental to advanced level training, with on-demand, live, and virtual options to suit your busy schedule. Certifications help you validate and prove your skill and expertise in Google Cloud technologies.
Manual Last Updated October 7, 2024
Lab Last Tested October 7, 2024
Copyright 2025 Google LLC All rights reserved. Google and the Google logo are trademarks of Google LLC. All other company and product names may be trademarks of the respective companies with which they are associated.
Ce contenu n'est pas disponible pour le moment
Nous vous préviendrons par e-mail lorsqu'il sera disponible
Parfait !
Nous vous contacterons par e-mail s'il devient disponible
One lab at a time
Confirm to end all existing labs and start this one