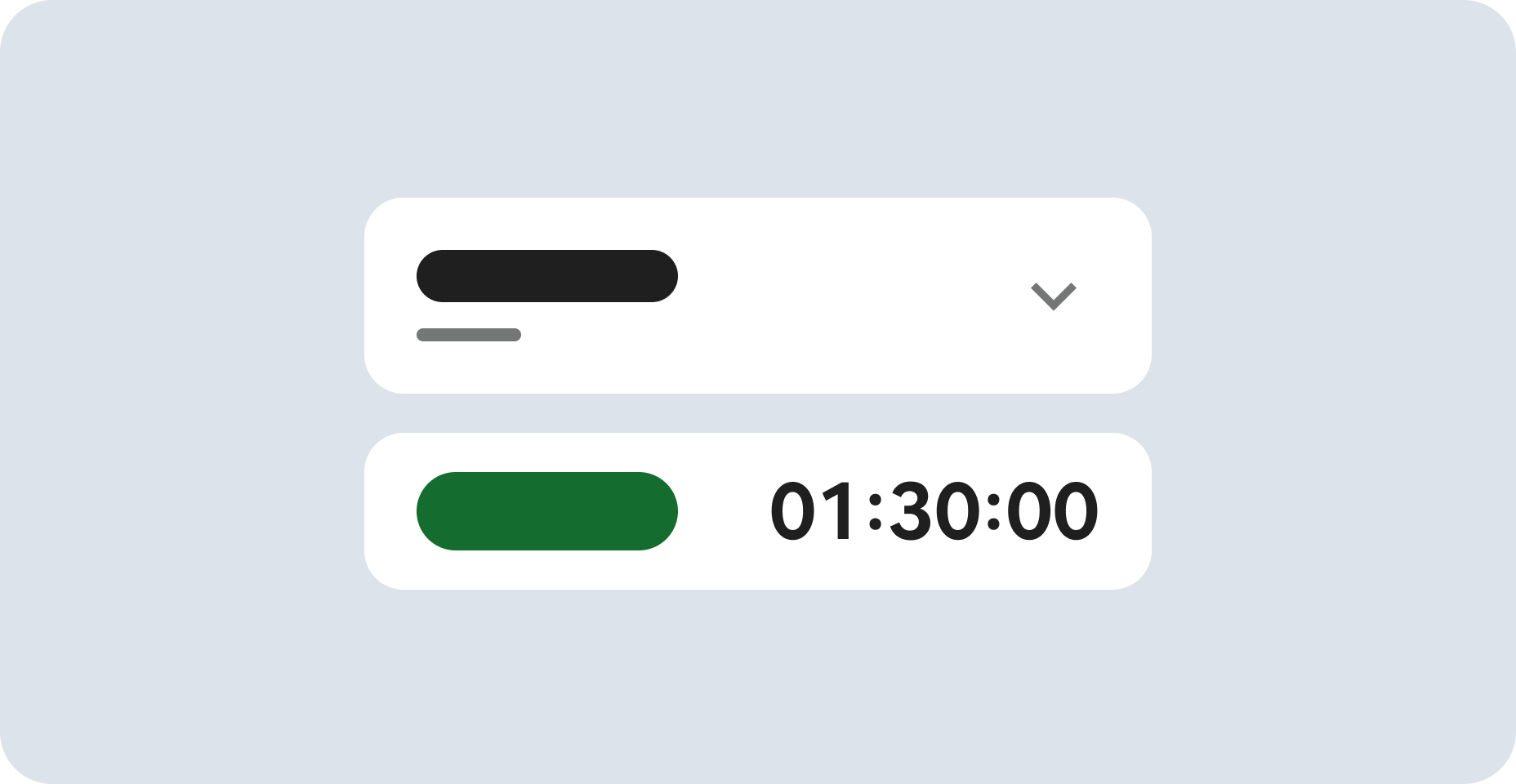
Before you begin
- Labs create a Google Cloud project and resources for a fixed time
- Labs have a time limit and no pause feature. If you end the lab, you'll have to restart from the beginning.
- On the top left of your screen, click Start lab to begin
Create a Cloud Storage Bucket
/ 50
Create a dataset
/ 50
Vertex AI AutoML helps developers with limited ML expertise train high quality image recognition models. Once you upload images to the AutoML UI, you can train a model that will be immediately available on Google Cloud for generating predictions via an easy to use REST API.
In this lab, you upload images to Cloud Storage and use them to train a custom model to recognize different types of clouds (cumulus, cumulonimbus, etc.).
In this lab, you do the following:
Read these instructions. Labs are timed and you cannot pause them. The timer, which starts when you click Start Lab, shows how long Google Cloud resources will be made available to you.
This hands-on lab lets you do the lab activities yourself in a real cloud environment, not in a simulation or demo environment. It does so by giving you new, temporary credentials that you use to sign in and access Google Cloud for the duration of the lab.
To complete this lab, you need:
Since this is a temporary account, which will last only as long as this lab:
Cloud Shell is a virtual machine that contains development tools. It offers a persistent 5-GB home directory and runs on Google Cloud. Cloud Shell provides command-line access to your Google Cloud resources. gcloud
is the command-line tool for Google Cloud. It comes pre-installed on Cloud Shell and supports tab completion.
Click the Activate Cloud Shell button () at the top right of the console.
Click Continue.
It takes a few moments to provision and connect to the environment. When you are connected, you are also authenticated, and the project is set to your PROJECT_ID.
(Output)
(Example output)
(Output)
(Example output)
Click Check my progress to verify the objective.
In order to train a model to classify images of clouds, you need to provide labelled training data so the model can develop an understanding of the image features associated with different types of clouds. In this example your model will learn to classify three different types of clouds: cirrus, cumulus, and cumulonimbus. To use AutoML you need to put your training images in Cloud Storage.
Run the following command in Cloud Shell:
The training images are publicly available in a Cloud Storage bucket.
If you click on the individual image files in each folder you can see the photos you'll be using to train your model for each type of cloud.
Now that your training data is in Cloud Storage, you need a way for AutoML to access it. You'll create a CSV file where each row contains a URL to a training image and the associated label for that image. This CSV file has been created for you; you just need to update it with your bucket name.
Once that command completes, click the Refresh button at the top of the Storage browser. Confirm that you see the data.csv file in your bucket.
Open the Vertex AI Dataset tab. Your page should now resemble the following:
At the top of the console, click + CREATE.
Type clouds for the Dataset name.
Select Image classification (Single-label).
Click Create.
Choose Select import files from Cloud Storage and add the file name to the URL for the file you just uploaded - your-bucket-name/data.csv
An easy way to get this link is to go back to the Cloud Console, click on the data.csv file and then go to the URI field.
It will take 2 - 5 minutes for your images to import. Once the import has completed, you'll be brought to a page with all the images in your dataset.
Click Check my progress to verify the objective.
After the import completes, you will be redirected to Browse tab to see the images you uploaded.
Try filtering by different labels in the left menu (i.e. click cumulus) to review the training images:
If any images are labeled incorrectly you can click on the image to switch the label:
You're ready to start training your model! AutoML handles this for you automatically, without requiring you to write any of the model code.
To train your clouds model, click TRAIN NEW MODEL.
On the Training method tab, click Continue.
On the Model details tab, click Continue.
On the Training options tab, click Continue.
On the Explainability tab, click Continue.
On the Compute and pricing tab, set the node hours to 8.
There are a few ways to generate predictions. In this lab, you'll use the UI to upload images. You'll see how your model does classifying these two images (the first is a cirrus cloud, the second is a cumulonimbus).
Return to the Cloudshell terminal.
Download these images to your local machine.
Expected Output:
Test your understanding of AutoML by completing the short quiz on the topics covered in this lab.
Use the knowledge you have gained in the lab to generate predictions.
Check if the model can predict the type of Cloud in an image:
CLOUD1-JSON
as the input file.Lets check if our model can predict the type of Cloud in the image:
CLOUD2-JSON
as the input file.You've learned how to train your own custom machine learning model and generate predictions on it through the web UI. Now you've got what it takes to train a model on your own image dataset.
When you have completed your lab, click End Lab. Qwiklabs removes the resources you’ve used and cleans the account for you.
You will be given an opportunity to rate the lab experience. Select the applicable number of stars, type a comment, and then click Submit.
The number of stars indicates the following:
You can close the dialog box if you don't want to provide feedback.
For feedback, suggestions, or corrections, please use the Support tab.
This content is not currently available
We will notify you via email when it becomes available
Great!
We will contact you via email if it becomes available
One lab at a time
Confirm to end all existing labs and start this one