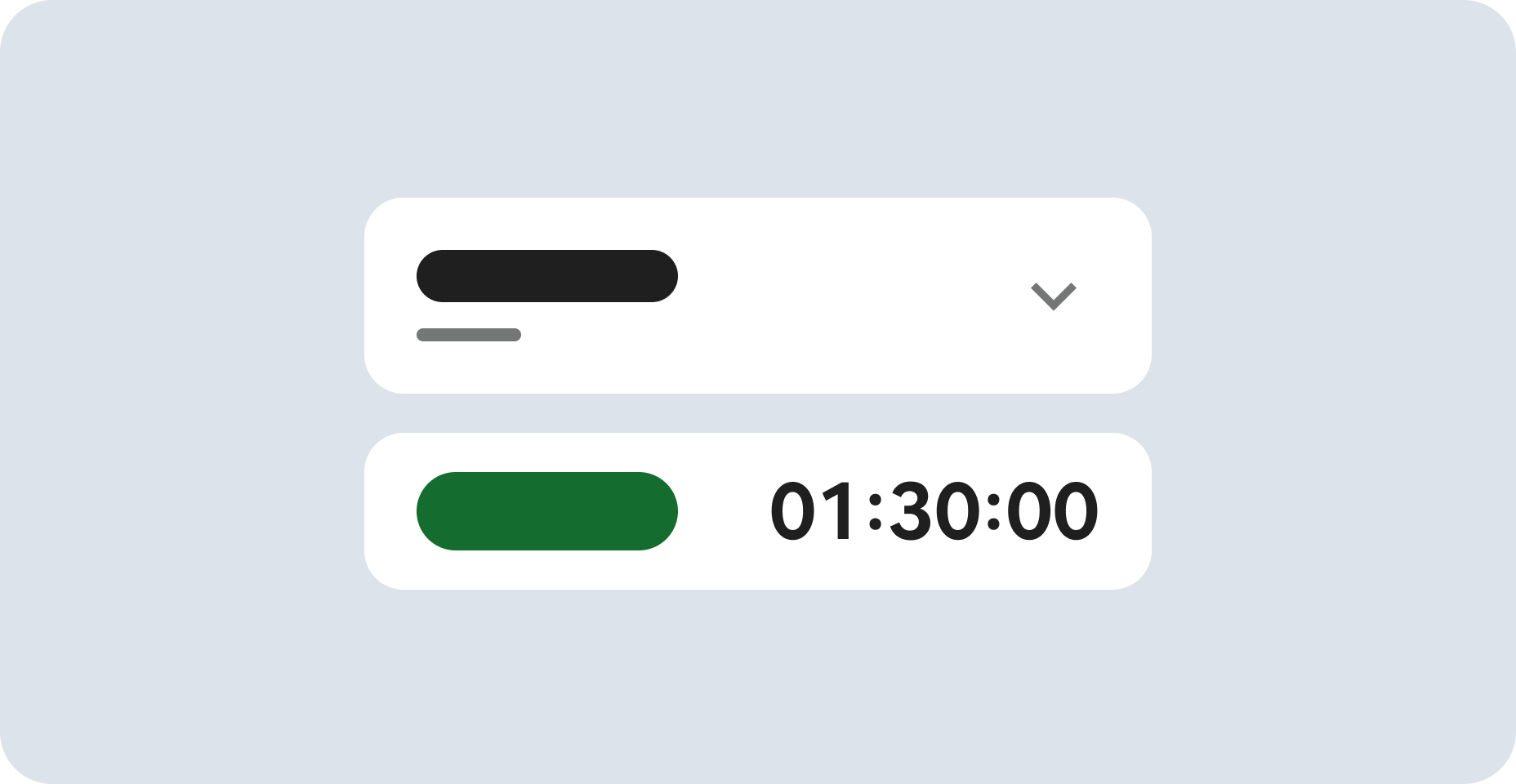
Before you begin
- Labs create a Google Cloud project and resources for a fixed time
- Labs have a time limit and no pause feature. If you end the lab, you'll have to restart from the beginning.
- On the top left of your screen, click Start lab to begin
In this lab, you will open a Dataflow project, use pipeline filtering, and execute the pipeline locally and on the cloud.
In this lab, you learn how to write a simple Dataflow pipeline and run it both locally and on the cloud.
For each lab, you get a new Google Cloud project and set of resources for a fixed time at no cost.
Sign in to Qwiklabs using an incognito window.
Note the lab's access time (for example, 1:15:00
), and make sure you can finish within that time.
There is no pause feature. You can restart if needed, but you have to start at the beginning.
When ready, click Start lab.
Note your lab credentials (Username and Password). You will use them to sign in to the Google Cloud Console.
Click Open Google Console.
Click Use another account and copy/paste credentials for this lab into the prompts.
If you use other credentials, you'll receive errors or incur charges.
Accept the terms and skip the recovery resource page.
Google Cloud Shell is a virtual machine that is loaded with development tools. It offers a persistent 5GB home directory and runs on the Google Cloud.
Google Cloud Shell provides command-line access to your Google Cloud resources.
In Cloud console, on the top right toolbar, click the Open Cloud Shell button.
Click Continue.
It takes a few moments to provision and connect to the environment. When you are connected, you are already authenticated, and the project is set to your PROJECT_ID. For example:
gcloud is the command-line tool for Google Cloud. It comes pre-installed on Cloud Shell and supports tab-completion.
Output:
Example output:
Output:
Example output:
Before you begin your work on Google Cloud, you need to ensure that your project has the correct permissions within Identity and Access Management (IAM).
In the Google Cloud console, on the Navigation menu (), select IAM & Admin > IAM.
Confirm that the default compute Service Account {project-number}-compute@developer.gserviceaccount.com
is present and has the editor
role assigned. The account prefix is the project number, which you can find on Navigation menu > Cloud Overview > Dashboard.
editor
role, follow the steps below to assign the required role.729328892908
).{project-number}
with your project number.You will be running all code from a curated training VM.
In the console, on the Navigation menu (), click Compute Engine > VM instances.
Locate the line with the instance called training-vm.
On the far right, under Connect, click on SSH to open a terminal window.
In this lab, you will enter CLI commands on the training-vm.
Follow these instructions to create a bucket.
In the Console, on the Navigation menu, click Cloud Storage > Buckets.
Click + Create.
Specify the following, and leave the remaining settings as their defaults:
Property | Value (type value or select option as specified) |
---|---|
Name | |
Location type | Multi-region |
Click Create.
If you get the Public access will be prevented
prompt, select Enforce public access prevention on this bucket
and click Confirm.
Record the name of your bucket. You will need it in subsequent tasks.
You can use $BUCKET
in terminal commands. And if you need to enter the bucket name <your-bucket> in a text field in the console, you can quickly retrieve the name with echo $BUCKET
.
The goal of this lab is to become familiar with the structure of a Dataflow project and learn how to execute a Dataflow pipeline.
Return to the training-vm SSH terminal and navigate to the directory /training-data-analyst/courses/data_analysis/lab2/python
and view the file grep.py
.
View the file with Nano. Do not make any changes to the code:
Can you answer these questions about the file grep.py
?
There are three transforms in the pipeline:
grep.py
:The output file will be output.txt
. If the output is large enough, it will be sharded into separate parts with names like: output-00000-of-00001
.
Examine the output file(s).
You can replace "-*" below with the appropriate suffix:
Does the output seem logical?
grepc.py
:Save the file and close Nano by pressing the CTRL+X key, then type Y, and press Enter.
Because this is such a small job, running on the cloud will take significantly longer than running it locally (on the order of 7-10 minutes).
Return to the browser tab for the console.
On the Navigation menu, click VIEW ALL PRODUCTS. In the Analytics section, click Dataflow and click on your job to monitor progress.
Wait for the Job status to be Succeeded.
Examine the output in the Cloud Storage bucket.
On the Navigation menu, click Cloud Storage > Buckets and click on your bucket.
Click the javahelp directory.
This job generates the file output.txt
. If the file is large enough, it will be sharded into multiple parts with names like: output-0000x-of-000y
. You can identify the most recent file by name or by the Last modified field.
Alternatively, you can download the file via the training-vm SSH terminal and view it:
When you have completed your lab, click End Lab. Google Cloud Skills Boost removes the resources you’ve used and cleans the account for you.
You will be given an opportunity to rate the lab experience. Select the applicable number of stars, type a comment, and then click Submit.
The number of stars indicates the following:
You can close the dialog box if you don't want to provide feedback.
For feedback, suggestions, or corrections, please use the Support tab.
Copyright 2022 Google LLC All rights reserved. Google and the Google logo are trademarks of Google LLC. All other company and product names may be trademarks of the respective companies with which they are associated.
This content is not currently available
We will notify you via email when it becomes available
Great!
We will contact you via email if it becomes available
One lab at a time
Confirm to end all existing labs and start this one