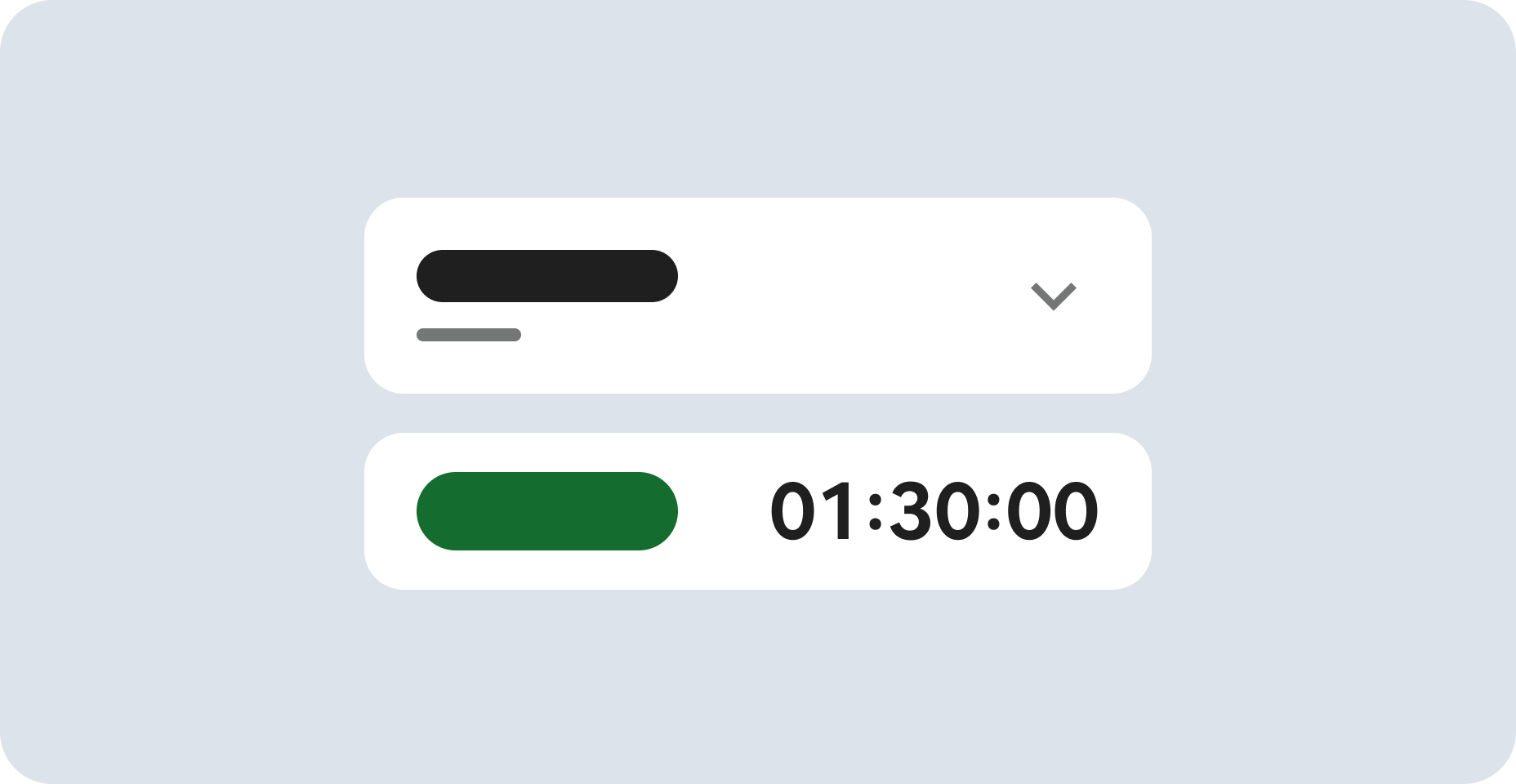
Before you begin
- Labs create a Google Cloud project and resources for a fixed time
- Labs have a time limit and no pause feature. If you end the lab, you'll have to restart from the beginning.
- On the top left of your screen, click Start lab to begin
Explore weather data
/ 25
Explore New York citizen complaints data
/ 25
Saving a new table of weather data
/ 20
Find correlation between weather and complaints
/ 30
In this lab you will analyze historical weather observations from NOAA using BigQuery, then use the weather data in conjunction with citizen complaints to 311 in New York City - very similar to Reto Meier's blog post that demonstrates how data variables can correlate to each other.
You will try out several aspects of Google Cloud that are of great benefit to scientists:
The end-result is that you will find what types of citizen complaints are correlated to weather. For example, you will find (not surprisingly) that complaints about residential furnaces are most common when it is cold outside.
In this lab, you'll:
CORR
function to determine positive, negative, or no correlation between datasets.This is a introductory level lab and assumes some experience with BigQuery and SQL. If you have never worked with BigQuery or MySQL, the BigQuery: Qwik Start - Console lab can get you up to speed with these Google Cloud services.
Read these instructions. Labs are timed and you cannot pause them. The timer, which starts when you click Start Lab, shows how long Google Cloud resources are made available to you.
This hands-on lab lets you do the lab activities in a real cloud environment, not in a simulation or demo environment. It does so by giving you new, temporary credentials you use to sign in and access Google Cloud for the duration of the lab.
To complete this lab, you need:
Click the Start Lab button. If you need to pay for the lab, a dialog opens for you to select your payment method. On the left is the Lab Details pane with the following:
Click Open Google Cloud console (or right-click and select Open Link in Incognito Window if you are running the Chrome browser).
The lab spins up resources, and then opens another tab that shows the Sign in page.
Tip: Arrange the tabs in separate windows, side-by-side.
If necessary, copy the Username below and paste it into the Sign in dialog.
You can also find the Username in the Lab Details pane.
Click Next.
Copy the Password below and paste it into the Welcome dialog.
You can also find the Password in the Lab Details pane.
Click Next.
Click through the subsequent pages:
After a few moments, the Google Cloud console opens in this tab.
The Welcome to BigQuery in the Cloud Console message box opens. This message box provides a link to the quickstart guide and the release notes.
The BigQuery console opens.
The Add data window opens.
Click Star a project by name under Additional sources.
Enter bigquery-public-data
and click STAR.
In the BigQuery console you see two projects in the Explorer pane, one named your lab project ID, and one named bigquery-public-data.
In the Explorer pane of the BigQuery console, expand bigquery-public-data dataset. In the Type to search field search for noaa_gsod and select the gsod2014 table.
In the Table (gsod2014) window, click the Preview tab.
Examine the columns and some of the data values.
Click Query > In new tab then paste the following query:
Click Check my progress below to verify you're on track in this lab.
In the Explorer pane of the BigQuery Console, select the newly added bigquery-public-data project, in the Type to search field search for the new_york_311 dataset, then select 311_service_requests table.
Then click on the Preview tab. Your console should resemble the following:
Examine the columns and some of the data values.
If the editor has been closed, click "+" (Create SQL query) icon.
Paste the following into the query EDITOR:
Click RUN.
Look at the results to determine what the most common complaints are. You will try to determine if these complaints correlate to weather in a later part of this lab.
Click Check my progress below to verify you're on track in this lab.
In the Explorer pane of the BigQuery Console, click on the three dots next to your Project ID
and then select Create dataset.
In the Create dataset dialog, set the Dataset ID to demos
and leave the other options at their default values.
Click Create dataset. Your project now has a dataset named demos
.
Click "+" (Create SQL query) icon and then run the following query:
In the query EDITOR section, click More > Query settings.
In the Query settings dialog, set the following fields. Leave all others at their default value.
Destination:
select Set a destination table for query results
Dataset:
Type demos and select your dataset.
Table Id
: Type nyc_weather
Results size
: check Allow large results (no size limit)
Click SAVE
Click RUN.
The results are now saved in the dataset you created (demos).
Navigate back to More > Query settings and, in the Destination option select Save query results in a temporary table. This removes the demos dataset as a destination for future queries.
Click SAVE to close the query settings.
Click Check my progress below to verify you're on track in this lab.
Strong correlation, as measured by the CORR function
, indicates a close and consistent relationship between two variables. As the value of one variable increases, the value of the other variable also tends to increase (positive correlation) or decrease (negative correlation) in a predictable way. Strong correlation is generally considered to be a value greater than or equal to 0.7, in absolute terms. This means that the changes in one variable can explain at least 49% of the changes in the other variable.
Next you will compare the number of complaints received and daily temperature using the CORR function.
The results indicate that Heating complaints are negatively correlated with temperature (i.e., more heating calls on cold days) and calls about dead trees are positively correlated with temperature (i.e., more calls on hot days).
Next, compare the number of complaints and wind speed with the CORR function.
Click Check my progress below to verify you're on track in this lab.
In this lab you were able to query the data without setting up any clusters, creating any indexes, etc. You were also able to mash up the two datasets and correlate the results, which gave you some interesting insights.
Read more on the CORR() function in BigQuery.
Learn more about BigQuery public data sets.
...helps you make the most of Google Cloud technologies. Our classes include technical skills and best practices to help you get up to speed quickly and continue your learning journey. We offer fundamental to advanced level training, with on-demand, live, and virtual options to suit your busy schedule. Certifications help you validate and prove your skill and expertise in Google Cloud technologies.
Manual Last Updated March 20, 2025
Lab Last Tested March 20, 2025
Copyright 2025 Google LLC All rights reserved. Google and the Google logo are trademarks of Google LLC. All other company and product names may be trademarks of the respective companies with which they are associated.
Diese Inhalte sind derzeit nicht verfügbar
Bei Verfügbarkeit des Labs benachrichtigen wir Sie per E-Mail
Sehr gut!
Bei Verfügbarkeit kontaktieren wir Sie per E-Mail
One lab at a time
Confirm to end all existing labs and start this one