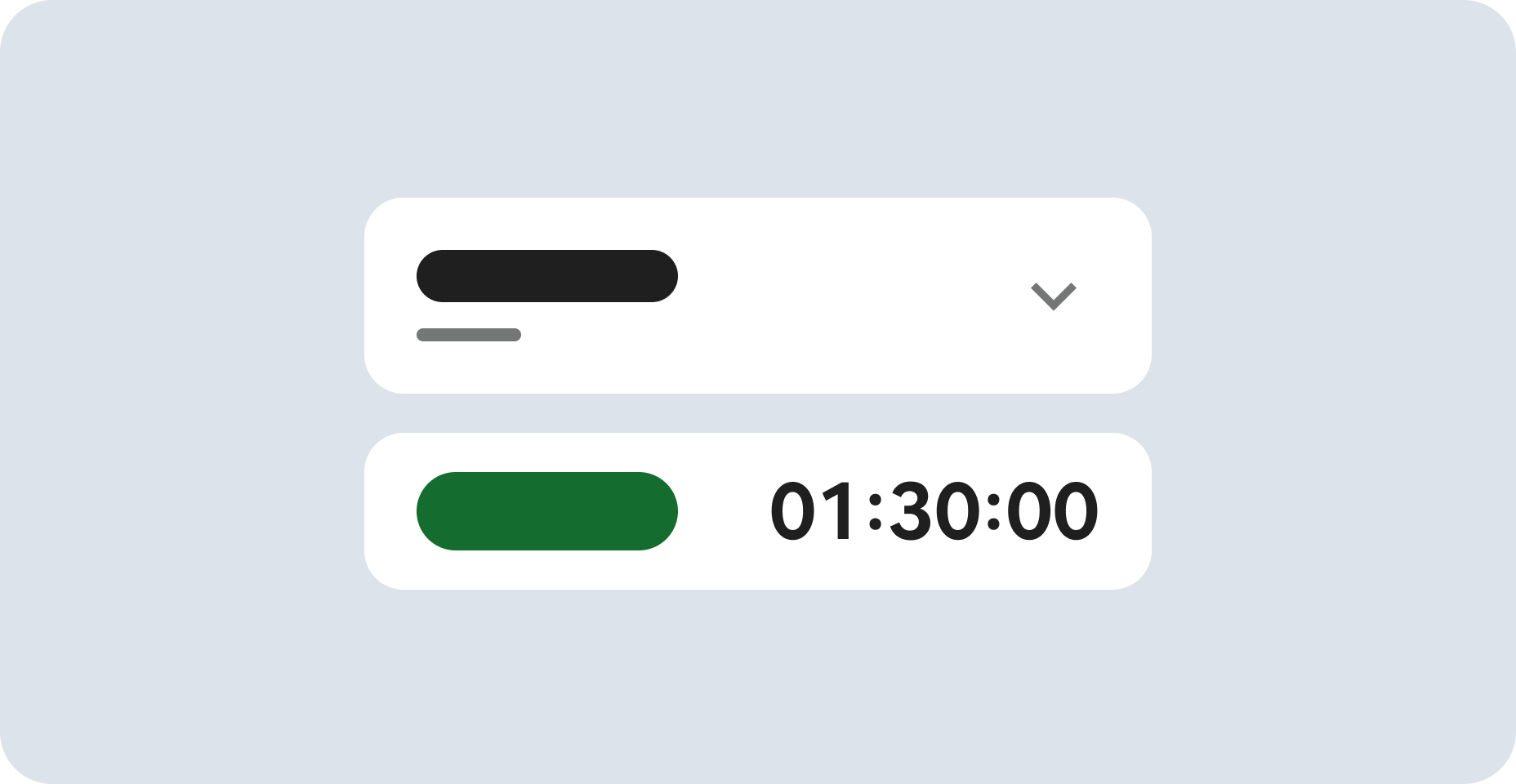
Before you begin
- Labs create a Google Cloud project and resources for a fixed time
- Labs have a time limit and no pause feature. If you end the lab, you'll have to restart from the beginning.
- On the top left of your screen, click Start lab to begin
Explore your billing data in BigQuery
/ 20
Run the query to get service.description column values
/ 20
Run a query to find out which services are used the most and least
/ 20
Run the query to get the region in which the Google Cloud service ran
/ 20
Run the query to find out which regions are used the most and the least by a service
/ 20
Looker Studio allows you to unlock the power of your data with interactive dashboards and beautiful reports that inspire smarter business decisions.
With Looker Studio, you can:
In this lab, you create data visualizations with Looker Studio. You first explore a sample Google Cloud bill and learn how to export the billing data to BigQuery — Google's serverless, highly scalable enterprise data warehouse that is designed to make data analysts more productive with unmatched price-performance.
After running a few SQL queries on your billing data, you export those metrics to Looker Studio, where you explore the service's chief features and build your own billing data visualizations.
In this lab, you learn how to:
Once you're ready, scroll down and follow the steps below to get your lab environment set up.
Read these instructions. Labs are timed and you cannot pause them. The timer, which starts when you click Start Lab, shows how long Google Cloud resources are made available to you.
This hands-on lab lets you do the lab activities in a real cloud environment, not in a simulation or demo environment. It does so by giving you new, temporary credentials you use to sign in and access Google Cloud for the duration of the lab.
To complete this lab, you need:
Click the Start Lab button. If you need to pay for the lab, a dialog opens for you to select your payment method. On the left is the Lab Details pane with the following:
Click Open Google Cloud console (or right-click and select Open Link in Incognito Window if you are running the Chrome browser).
The lab spins up resources, and then opens another tab that shows the Sign in page.
Tip: Arrange the tabs in separate windows, side-by-side.
If necessary, copy the Username below and paste it into the Sign in dialog.
You can also find the Username in the Lab Details pane.
Click Next.
Copy the Password below and paste it into the Welcome dialog.
You can also find the Password in the Lab Details pane.
Click Next.
Click through the subsequent pages:
After a few moments, the Google Cloud console opens in this tab.
In this task you explore your billing data and identify the dataset you want to focus on. This lab provides sample data for you to use.
In the Google Cloud console, in the Navigation menu (), click Billing.
In the Billing window, click Manage billing accounts.
Click GCP Cost Management Billing Demo.
The Billing Account Overview window opens.
In the Billing account pane, click Manage billing account.
The Google Cloud projects associated with this billing account are listed:
These four Google Cloud projects illustrate a common enterprise schema, where you have different projects for development, production, storage, and sandbox testing.
In the Cloud console, in the Navigation menu (), click Billing > Overview.
This window provides an overview of the Billing Account.
Scroll down to explore the data and charts that track your billing account.
In the Cloud console, in the Navigation menu (), click Billing > Reports.
This window provides a report for your billing account. Notice the cost trends per project, when prices rise and fall, and the ability to filter the report.
In this task you use BigQuery to easily query and filter large datasets, aggregate results, and perform complex operations to optimize data analysis. BigQuery is a fully managed data warehouse that runs on Google Cloud.
The billing data you explored in the previous section was exported to BigQuery when this lab was spun up. This section describes the process for information purposes only.
In the Google Cloud console, in the Navigation menu (), click Billing > Billing export.
The Billing export window opens with the BigQuery Export tab selected by default.
Click Edit settings to show the export options.
Click Projects, and then select the project that contains your billing account.
Click Billing export dataset, and then set the BigQuery dataset where you want to host this data.
Click Save.
This initiates a job where your billing data is saved as a table in the selected BigQuery dataset.
Note that a billing export takes some time to populate (a few hours to a day).
The billing data for this lab has already been exported to a table in BigQuery. Following BigQuery's project.dataset.table
convention, the full path to the billing data is:
You use this path to query project data.
In this task you use an SQL query in BigQuery to see what information is available. The billing data was automatically exported into BigQuery when the lab was spun up.
In the Cloud console, in the Navigation menu (), click BigQuery.
Under Select a recent project, click the tile with your Project ID.
In the Welcome dialog, click Done.
The BigQuery console opens. The Explorer pane displays your project. Your project name is your Project ID.
In the Query Editor, type the following, and then click Run:
SELECT *
returns all column values from a specified table.
You should see the resulting table in the Query results section.
Click Check my progress to verify your performed task. If you have successfully run a query to get all the column values from a table, you will receive an assessment score.
Under the table in Query results, there are 1 million plus rows of data.
You found the answer to this question by looking at the BigQuery table created in your first SQL query. For more complicated questions, you would run more complicated SQL queries to analyze your data and gain valuable insights.
In this task, you ask two questions, and use BigQuery to get that information. You then use Looker Studio to build reports with data visualizations to share those insights.
In the previous task you explored a sample billing account in BigQuery that has millions of rows of information. For this information to be useful you must be able to analyze the data to obtain specific information. In BigQuery, you run SQL queries to answer questions to obtain that specific information.
You want to find which service types are most and least used, so you must determine:
For the answers, you run SQL queries on the billing data hosted in BigQuery.
In the Query editor, clear the current query.
In the Query editor, type the following, and then click Run:
This query reveals which service is associated with each log.
The service.description
column tells you what Google Cloud service is associated with each log. The GROUP BY
keyword aggregates result-set rows that share common criteria (in this case the service description) and returns all of the unique entries found for such criteria.
In the Query results section, in the Results tab, you see that the four projects use 15 different types of Google Cloud services.
Click Check my progress to verify your performed task. If you have successfully run a query to get service.description column values, you will receive an assessment score.
In the Query editor, clear the current query.
In the Query editor, type the following, and then click Run:
This query determines which service types are most and least used.
The COUNT(*)
function returns the number of rows that share the same criteria (in this case the service description).
BigQuery shows your results in the Query results section in a table with two columns: description and num. Compare the numbers in the num column to determine which service type was the most and least used.
Click Check my progress to verify your performed task. If you have successfully run a query to find out which services are used the most and least, you will receive an assessment score.
Open Looker Studio in a new tab.
Click Create > Explorer.
Select Country and Enter Company name.
Agree to the Terms of Service, and then click Continue.
In email preferences, select Yes to all (this is connected to your temporary student email).
Click Continue.
In the Add Data pane, click Create New Data.
In the Google Connectors window, select BigQuery.
Click Authorize.
In Recent Projects, select Custom Query.
In Billing Project, select your project ID.
In the Customer Query pane, type the query you used previously:
Click Connect.
Click Apply.
Click Untitled Explorer, and rename this report as Services Breakdown.
In the Setup pane, in the Metric section, hold the pointer over Record Count, and click X to remove that metric.
Click Add metric, and select num.
You may have to scroll down to see the num menu option.
Click Add a Chart, select the Pie chart.
Looker Studio generates a pie chart on the use of services.
Click Save.
Close the Looker Studio browser tab and return to the BigQuery console browser tab. You are now ready to answer the second question.
To find which regions are most and least used across all four projects, you must determine:
In the Query editor, clear the current query.
In the Query editor, type the following, and then click Run:
The results are a single region column that lists the regions the Google Cloud service ran in. A null
region means the region is not known.
Click Check my progress to verify your performed task. If you have successfully run a query to get the regions that the Google Cloud service ran in, you will see an assessment score.
In the Query editor, clear the current query.
In the Query editor, type the following, and then click Run:
Your results are two columns, region and num. Compare the results to determine which regions are most and least used.
Click Check my progress to verify your performed task. If you have successfully run the query to find out which regions are used the most and the least by a service, you will receive an assessment score.
Open Looker Studio in a new tab.
Click Create > Explorer.
In the Add Data pane, click Create New Data.
In the Google Connectors window, select BigQuery.
In Recent Projects, select Custom Query.
In Billing Project, select your project ID.
Type the query you used previously:
Click Connect.
Click Apply.
Click Untitled Explorer, and rename this report to Regions Breakdown.
In the Setup pane, in the Metric section, hold the pointer over Record Count, and click X to remove that metric.
Click Add metric, and select num.
Click Add a Chart, select the Pie chart.
Looker Studio generates a pie chart on the use of services.
You have successfully created two data visualizations from your billing data queries.
Click SAVE in the top-right corner to save your visualization.
Click Looker Studio
To see the visualizations you just created, click Regions Breakdown.
In this lab, you explored the billing service in the console and learned how to export your billing data to BigQuery. After exploring the data with SQL queries, you exported your aggregated data to Looker Studio, where you generated pie chart visualizations of service and region consumption.
...helps you make the most of Google Cloud technologies. Our classes include technical skills and best practices to help you get up to speed quickly and continue your learning journey. We offer fundamental to advanced level training, with on-demand, live, and virtual options to suit your busy schedule. Certifications help you validate and prove your skill and expertise in Google Cloud technologies.
Manual Last Updated January 22, 2025
Lab Last Tested September 04, 2024
Copyright 2025 Google LLC. All rights reserved. Google and the Google logo are trademarks of Google LLC. All other company and product names may be trademarks of the respective companies with which they are associated.
This content is not currently available
We will notify you via email when it becomes available
Great!
We will contact you via email if it becomes available
One lab at a time
Confirm to end all existing labs and start this one