The right Cloud learning for you
Upskill yourself or your team with Google Cloud Skills Boost. From beginners to experts, find the credentials and trainings you need to achieve your goals.Skill up on AI today
AI isn’t a future state. It’s here to stay. With more than a decade of AI innovation and integration in the cloud, Google is your trusted partner in navigating this new reality.Developer? Become an Innovator.
Continuous learning starts with community. Join the Innovators community and fuel your cloud journey with 35 free monthly credits for hands-on learning with Google Cloud Skills Boost.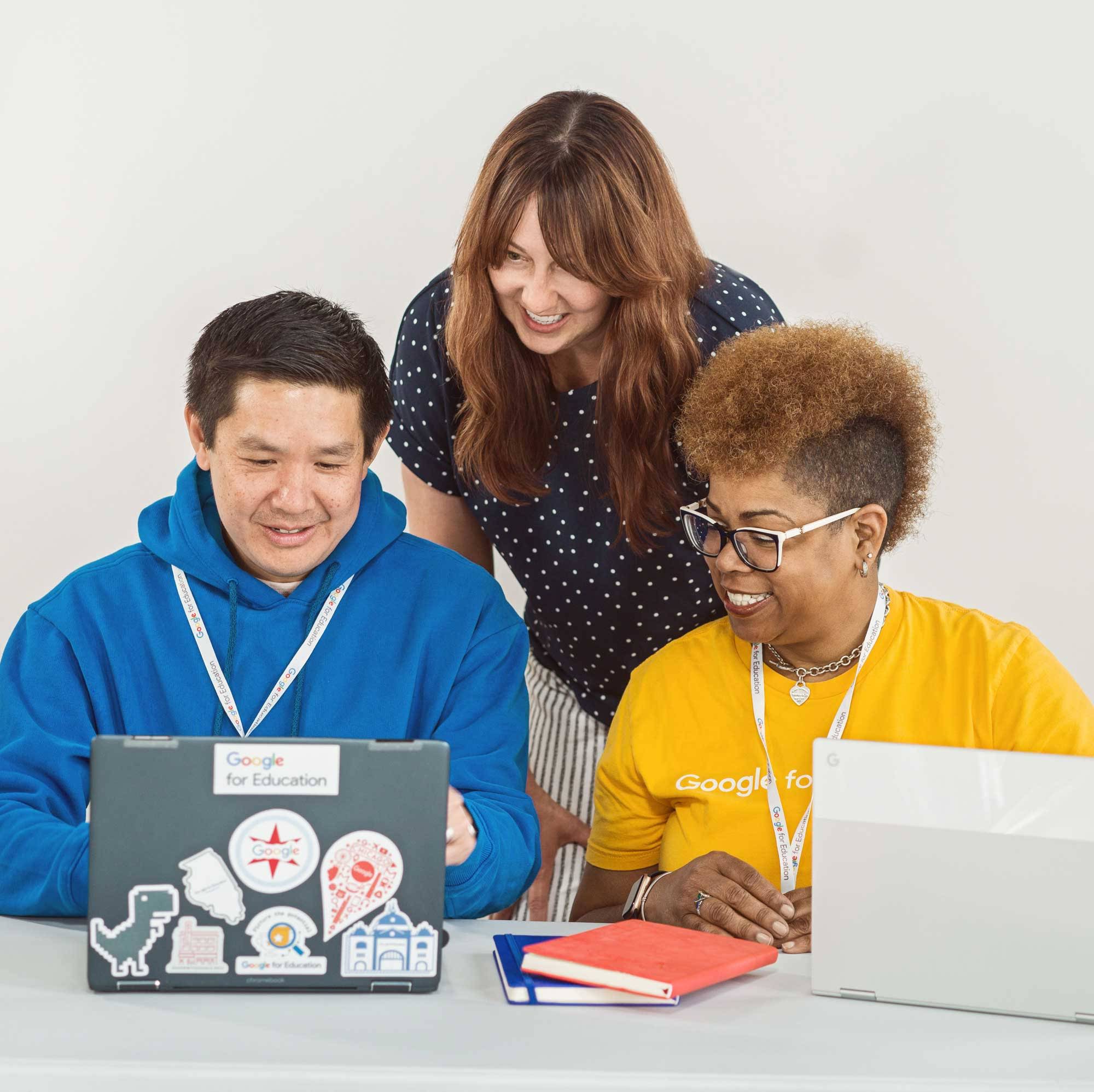
Teams that get hands-on learning and instructor-led training see better business results.
77%
of learners prefer some level of instructor-led training vs. on-demand training alone.90%
of instructor-led learning is completed vs. less than 5% of self-paced.133%
increase in employee retention when companies invest in hands-on learning.Which Google Cloud credential is right for you?
Certifications can be a big step, and a big investment. Need to build your skills first? Explore our Skill Badges and Certificates.Certifications
Prove your cloud expertise and validate your skills with industry-recognized certifications.
Explore certificationsSkill Badges
These hands-on digital credentials demonstrate how your skills apply in real-world scenarios.
Explore skill badgesCertificates
Certificates are a great way to start your cloud career and build the skills for in-demand roles.
Explore certificates