Xai Lex
メンバー加入日: 2022
ゴールドリーグ
43575 ポイント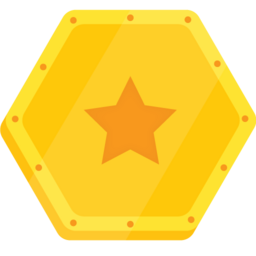
メンバー加入日: 2022
This Course is utilized to certify completion of The Skills Challenge at Next 2025.
このコースでは、AI のプライバシーと安全性に関する重要なトピックを紹介します。具体的には、Google Cloud プロダクトとオープンソース ツールを使用して AI のプライバシーと安全性の推奨プラクティスを実装するための実践的な方法とツールを検証します。
Engineer Data for Predictive Modeling with BigQuery ML スキルバッジを獲得できる中級コースを修了すると、 Dataprep by Trifacta を使用した BigQuery へのデータ変換パイプラインの構築、Cloud Storage、Dataflow、 BigQuery を使用した抽出、変換、読み込み(ETL)ワークフローの構築、BigQuery ML を使用した ML モデルの構築、 Cloud Composer を使用した複数ロケーション間でのデータのコピーに関するスキルを実証できます。 スキルバッジは、Google Cloud のプロダクトとサービスの習熟度を示す Google Cloud 発行の限定デジタルバッジで、インタラクティブなハンズオン環境での知識の応用力を証明するものです。 スキルバッジ コースと最終評価チャレンジラボを完了し、デジタルバッジを獲得して ネットワークで共有しましょう。
「Create ML Models with BigQuery ML」コースの中級スキルバッジを獲得できるアクティビティを修了すると、 BigQuery ML を使用して ML モデルを作成および評価し、データを予測するスキルを証明できます。 スキルバッジは、Google Cloud のプロダクトとサービスの習熟度を示す Google Cloud 発行の限定デジタルバッジで、 インタラクティブなハンズオン環境での知識の応用力を証明するものです。このスキル バッジ コースと最終評価チャレンジラボを修了し、スキルバッジを獲得して ネットワークで共有しましょう。
This course is an introduction to Vertex AI Notebooks, which are Jupyter notebook-based environments that provide a unified platform for the entire machine learning workflow, from data preparation to model deployment and monitoring. The course covers the following topics: (1) The different types of Vertex AI Notebooks and their features and (2) How to create and manage Vertex AI Notebooks.
「Prepare Data for ML APIs on Google Cloud」コースの入門スキルバッジを獲得できるアクティビティを修了すると、 Dataprep by Trifacta を使用したデータのクリーニング、Dataflow でのデータ パイプラインの実行、Dataproc でのクラスタの作成と Apache Spark ジョブの実行、 Cloud Natural Language API、Google Cloud Speech-to-Text API、Video Intelligence API などの ML API の呼び出しに関するスキルを証明できます。 スキルバッジは、Google Cloud のプロダクトとサービスの習熟度を示す Google Cloud 発行の限定デジタルバッジで、 インタラクティブなハンズオン環境での知識の応用力を証明するものです。このスキルバッジ コースと最終評価チャレンジラボを修了し、 スキルバッジを獲得してネットワークで共有しましょう。
このコースでは、AI の解釈可能性と透明性のコンセプトを紹介します。デベロッパーとエンジニアにとって AI の透明性が重要であることについて説明します。データと AI モデルの両方で解釈可能性と透明性を達成できる実践的な方法とツールを検証します。
このコースでは、責任ある AI および AI に関する原則のコンセプトを紹介します。AI / ML の実践における公平性とバイアスを特定し、バイアスを軽減するための実践的な手法を取り扱います。具体的には、Google Cloud プロダクトとオープンソース ツールを使用して責任ある AI のベスト プラクティスを実装するための実践的な方法とツールを検証します。
This course helps learners create a study plan for the PMLE (Professional Machine Learning Engineer) certification exam. Learners explore the breadth and scope of the domains covered in the exam. Learners assess their exam readiness and create their individual study plan.
Generative AI applications can create new user experiences that were nearly impossible before the invention of large language models (LLMs). As an application developer, how can you use generative AI to build engaging, powerful apps on Google Cloud? In this course, you'll learn about generative AI applications and how you can use prompt design and retrieval augmented generation (RAG) to build powerful applications using LLMs. You'll learn about a production-ready architecture that can be used for generative AI applications and you'll build an LLM and RAG-based chat application.
このコースでは、Vertex AI ベクトル検索を紹介し、エンべディングのための大規模言語モデル(LLM)API を組み込んだ検索アプリケーションを構築するために使用する方法を説明します。このコースは、ベクトル検索とテキスト エンベディングの概念の説明、Vertex AI でベクトル検索を構築する実用的なデモ、ハンズオンラボで構成されています。
Build and Deploy Machine Learning Solutions on Vertex AI コースを修了して、 中級スキルバッジを獲得しましょう。このコースでは、Google Cloud の Vertex AI プラットフォーム、AutoML、カスタム トレーニング サービスを使用して、 ML モデルのトレーニング、評価、チューニング、説明、デプロイを行う方法を学びます。 このスキルバッジ コースは、データ サイエンティストと ML エンジニアのプロフェッショナルを 対象としています。 スキルバッジは、Google Cloud のプロダクトとサービスの習熟度を示す Google Cloud 発行の限定デジタルバッジで、 インタラクティブなハンズオン環境での知識の応用力を証明するものです。このスキルバッジと 最終評価チャレンジラボを完了し、デジタルバッジを獲得して ネットワークで共有しましょう。
このコースでは、Google Cloud で最先端の ML パイプラインに携わっている ML エンジニアおよびトレーナーたちから知識を吸収することができます。 最初のいくつかのモジュールで、ML パイプラインとメタデータの管理用 TensorFlow を基盤とする Google の本番環境向け機械学習プラットフォーム TensorFlow Extended(TFX)について説明します。パイプラインのコンポーネントについて、そして TFX を使用したパイプラインのオーケストレーションについて学習します。また、継続的インテグレーションと継続的デプロイを通じたパイプラインの自動化の方法と、ML メタデータの管理方法についても学習します。その後、焦点を変えて、TensorFlow、PyTorch、Scikit Learn、XGBoost などの複数の ML フレームワーク全体にわたる ML パイプラインの自動化と再利用の方法について説明します。 さらに、Google Cloud のもう 1 つのツール、Cloud Composer を継続的なトレーニング パイプラインのオーケストレーションに活用する方法についても学習します。最後は、MLflow を使用して機械学習の完全なライフサイクルを管理する方法の解説で締めくくります。
このコースでは、ML の実務担当者に、生成 AI モデルと予測 AI モデルの両方を評価するための重要なツール、手法、ベスト プラクティスを身につけていただきます。モデル評価は、ML システムが本番環境で信頼性が高く、正確で、高性能な結果を確実に提供するための重要な分野です。 参加者は、さまざまな評価指標、方法論のほか、さまざまなモデルタイプやタスクにおけるそれらの適切な適用について理解を深めます。このコースでは、生成 AI モデルによってもたらされる固有の課題に重点を置き、それらの課題に効果的に取り組むための戦略を提供します。参加者は、Google Cloud の Vertex AI プラットフォームを活用して、モデルの選択、最適化、継続的なモニタリングのための堅牢な評価プロセスを実装する方法を学びます。
このコースでは、生成 AI モデルのデプロイと管理において MLOps チームが直面する特有の課題に対処するために必要な知識とツールを提供し、AI チームが MLOps プロセスを合理化して生成 AI プロジェクトを成功させるうえで Vertex AI がどのように役立つかを説明します。
このコースは、大規模言語モデル(LLM)とは何か、どのようなユースケースで活用できるのか、プロンプトのチューニングで LLM のパフォーマンスを高めるにはどうすればよいかについて学習する、入門レベルのマイクロ ラーニング コースです。独自の生成 AI アプリを開発する際に利用できる Google ツールも紹介します。
この入門レベルのマイクロラーニング コースでは、生成 AI の概要、利用方法、従来の機械学習の手法との違いについて説明します。独自の生成 AI アプリを作成する際に利用できる Google ツールも紹介します。
このコースでは、Google Cloud 上で本番環境の ML システムをデプロイ、評価、モニタリング、運用するための MLOps ツールとベスト プラクティスについて説明します。MLOps は、本番環境 ML システムのデプロイ、テスト、モニタリング、自動化に重点を置いた規範です。 受講者は、SDK レイヤで Vertex AI Feature Store のストリーミング取り込みを使用する実践的な演習を受けられます。
このコースでは、Google Cloud 上で本番環境の ML システムをデプロイ、評価、モニタリング、運用するための MLOps ツールとベスト プラクティスについて説明します。MLOps は、本番環境 ML システムのデプロイ、テスト、モニタリング、自動化に重点を置いた規範です。機械学習エンジニアリングの担当者は、ツールを活用して、デプロイしたモデルの継続的な改善と評価を行います。また、データ サイエンティストと協力して、あるいは自らがデータ サイエンティストとして、最も効果的なモデルを迅速かつ正確にデプロイできるようモデルを開発します。
In this course, you apply your knowledge of classification models and embeddings to build a ML pipeline that functions as a recommendation engine. This is the fifth and final course of the Advanced Machine Learning on Google Cloud series.
This course introduces the products and solutions to solve NLP problems on Google Cloud. Additionally, it explores the processes, techniques, and tools to develop an NLP project with neural networks by using Vertex AI and TensorFlow.
This course describes different types of computer vision use cases and then highlights different machine learning strategies for solving these use cases. The strategies vary from experimenting with pre-built ML models through pre-built ML APIs and AutoML Vision to building custom image classifiers using linear models, deep neural network (DNN) models or convolutional neural network (CNN) models. The course shows how to improve a model's accuracy with augmentation, feature extraction, and fine-tuning hyperparameters while trying to avoid overfitting the data. The course also looks at practical issues that arise, for example, when one doesn't have enough data and how to incorporate the latest research findings into different models. Learners will get hands-on practice building and optimizing their own image classification models on a variety of public datasets in the labs they will work on.
このコースでは、本番環境で高パフォーマンスな ML システムを構築するためのコンポーネントとベスト プラクティスについて学習します。また、ML システムを構築するうえで最も一般的な考慮事項を紹介します。これには、静的トレーニング、動的トレーニング、静的な推論、動的な推論、分散型 TensorFlow、TPU などが含まれます。このコースでは、優れた予測能力にとどまらない、優れた ML システムの特性を探索することに焦点を当てています。
このコースでは、ML ワークフローに対する実践的なアプローチを取り上げます。具体的には、いくつかの ML のビジネス要件とユースケースに取り組む ML チームをケーススタディ形式で紹介します。このチームは、データ マネジメントとガバナンスに必要なツールを理解し、データの前処理に最適なアプローチを検討する必要があります。 2 つのユースケースに対して ML モデルを構築するための 3 つのオプションがチームに提示されます。このコースでは、チームの目標を達成するために、AutoML、BigQuery ML、カスタム トレーニングを使用する理由について説明します。
このコースでは、Vertex AI Feature Store を使用するメリット、ML モデルの精度を向上させる方法、最も有効な特徴を抽出できるデータ列の見極め方について説明します。また、BigQuery ML、Keras、TensorFlow を使用した特徴量エンジニアリングに関するコンテンツとラボも用意されています。
このコースでは、TensorFlow と Keras を使用した ML モデルの構築、ML モデルの精度の向上、スケーリングに対応した ML モデルの作成について取り上げます。
このコースでは、まず、データ品質を向上させる方法や探索的データ分析を行う方法など、データについての議論から始めます。Vertex AI AutoML について確認し、コードを一切記述せずに ML モデルを構築、トレーニング、デプロイする方法を説明します。また、BigQuery ML のメリットを確認します。その後、ML モデルを最適化する方法、一般化とサンプリングを活用してカスタム トレーニング向けに ML モデルの品質を評価する方法を説明します。
このコースでは、予測 AI と生成 AI の両方のプロジェクトを構築できる、Google Cloud の AI および機械学習(ML)サービスについて紹介します。AI の基盤、開発、ソリューションを含むデータから AI へのライフサイクル全体で利用可能なテクノロジー、プロダクト、ツールについて説明するとともに、魅力的な学習体験と実践的なハンズオン演習を通じて、データ サイエンティスト、AI 開発者、ML エンジニアの方々がスキルや知識を強化できるようサポートすることを目指しています。
Implement Load Balancing on Compute Engine スキルバッジを獲得できる入門コースを修了すると、次のスキルを実証できます: gcloud コマンドの記述と Cloud Shell の使用、Compute Engine における仮想マシンの作成とデプロイ、 ネットワーク ロードバランサと HTTP ロードバランサの構成。 スキルバッジは、Google Cloud の プロダクトとサービスに関する習熟度を示す Google Cloud 発行の限定デジタルバッジです。 これは、インタラクティブなハンズオン環境での知識の応用力を 証明するものです。この入門コースと最終評価チャレンジラボを完了し、 スキルバッジを獲得しましょう。このスキルバッジはネットワークで共有できます。
このコースでは、実績ある設計パターンを利用して、信頼性と効率に優れたソリューションを Google Cloud で構築する方法を学習します。本コースは、Architecting with Google Compute Engine または Architecting with Google Kubernetes Engine のコースの続きで、これらのコースで取り上げているテクノロジーの実践経験があることを前提としています。参加者は、講義、設計アクティビティ、ハンズオンラボを通して、ビジネス要件と技術要件を定義し、バランスを取りながら、信頼性、可用性、安全性、費用対効果に優れた Google Cloud のデプロイを設計する方法を学びます。
多くの IT 組織では、アジリティを求める開発者と、安定性を重視する運用担当者の間で、インセンティブが調整されていません。サイト信頼性エンジニアリング(SRE)は、Google が開発と運用の間のインセンティブを調整し、ミッション クリティカルな本番環境サポートを行う方法です。SRE の文化的および技術的手法を導入することで、ビジネスと IT の連携を改善できます。このコースでは、Google の SRE の主な手法を紹介し、SRE の組織的な導入を成功させるうえで IT リーダーとビジネス リーダーが果たす重要な役割について説明します。
Google Cloud Fundamentals: Core Infrastructure では、Google Cloud に関する重要なコンセプトと用語について説明します。このコースでは動画とハンズオンラボを通じて学習を進めていきます。Google Cloud の多数のコンピューティング サービスとストレージ サービス、そしてリソースとポリシーを管理するための重要なツールについて比較しながら説明します。
「Google Cloud におけるアプリ開発環境の設定」コースを完了すると、スキルバッジを獲得できます。このコースでは、 Cloud Storage、Identity and Access Management、Cloud Functions、Pub/Sub のテクノロジーの基本機能を使用して、ストレージ中心のクラウド インフラストラクチャを構築し接続する方法を学びます。 スキルバッジは、Google Cloud のプロダクトとサービスの習熟度を示す Google Cloud 発行の限定デジタルバッジです。 インタラクティブなハンズオン環境で知識の応用力が試されます。このスキルバッジと最終評価チャレンジラボを完了し、スキルバッジを獲得してネットワークで共有しましょう。
この入門レベルのクエストでは、Google Cloud の基本的なツールやサービスに関する実践演習を行います。「Google Cloud Essentials」は Qwiklabs で特に人気のあるクエストですが、それはクラウドの予備知識がほとんどなくても、あらゆる Google Cloud プロジェクトに応用できる実際的な経験を積めるからです。 「Google Cloud Essentials」では、Cloud Shell コマンドの記述、初めての仮想マシンのデプロイ、Kubernetes Engine 上でのアプリケーション実行と負荷分散など、Google Cloud の主な機能を紹介します。主なコンセプトは 1 分間のビデオで説明されています。