Omar Irfan Khan
Учасник із 2021
Золота ліга
Кількість балів: 21305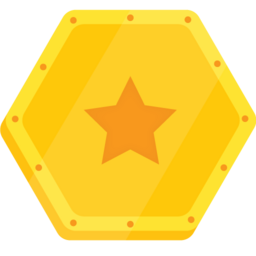
Учасник із 2021
This course will introduce you to the attention mechanism, a powerful technique that allows neural networks to focus on specific parts of an input sequence. You will learn how attention works, and how it can be used to improve the performance of a variety of machine learning tasks, including machine translation, text summarization, and question answering. This course is estimated to take approximately 45 minutes to complete.
This course introduces diffusion models, a family of machine learning models that recently showed promise in the image generation space. Diffusion models draw inspiration from physics, specifically thermodynamics. Within the last few years, diffusion models became popular in both research and industry. Diffusion models underpin many state-of-the-art image generation models and tools on Google Cloud. This course introduces you to the theory behind diffusion models and how to train and deploy them on Vertex AI.
Що більше штучний інтелект і машинне навчання використовуються в корпоративних середовищах, то нагальнішою стає потреба розробити принципи відповідального ставлення до них. Однак говорити про принципи відповідального використання штучного інтелекту легше, ніж застосовувати їх на практиці. Цей курс допоможе вам дізнатись, як запровадити відповідальну роботу зі штучним інтелектом у вашій організації. У цьому курсі ви дізнаєтеся про підхід Google Cloud до відповідального використання ШІ, а також отримаєте практичні поради й набудете досвіду, який допоможе вам розробити власний підхід до цього завдання.
In this course, you learn how Gemini, a generative AI-powered collaborator from Google Cloud, helps you secure your cloud environment and resources. You learn how to deploy example workloads into an environment in Google Cloud, identify security misconfigurations with Gemini, and remediate security misconfigurations with Gemini. Using a hands-on lab, you experience how Gemini improves your cloud security posture. Duet AI was renamed to Gemini, our next-generation model.
In this course, you learn how Gemini, a generative AI-powered collaborator from Google Cloud, helps you use Google products and services to develop, test, deploy, and manage applications. With help from Gemini, you learn how to develop and build a web application, fix errors in the application, develop tests, and query data. Using a hands-on lab, you experience how Gemini improves the software development lifecycle (SDLC). Duet AI was renamed to Gemini, our next-generation model.
This course is dedicated to equipping you with the knowledge and tools needed to uncover the unique challenges faced by MLOps teams when deploying and managing Generative AI models, and exploring how Vertex AI empowers AI teams to streamline MLOps processes and achieve success in Generative AI projects.
In this course, you'll learn about Google Vids, an online video creation and editing app available to select Google Workspace users. Through lessons and demos, you'll learn how to build and tell compelling stories through video at work. You'll also discover how to seamlessly incorporate media, audio and video clips, customize styles, and easily share your creations. Some Vids features use generative AI to help you work more efficiently. Remember, generative AI tools including Gemini, may suggest inaccurate or inappropriate information. Don’t rely on Gemini features as medical, legal, financial or other professional advice. It’s also important to remember that the Gemini feature suggestions don’t represent Google’s views, and should not be attributed to Google.
Gemini for Google Workspace provides users with access to generative AI features. This course delves into the capabilities of Gemini in Google Drive using video lessons, hands-on activities and practical examples. By the end of this course, you'll be equipped with the knowledge and skills to confidently utilize Gemini in Google Drive to improve your workflows.
Gemini for Google Workspace provides customers with access to generative AI features. This course delves into the capabilities of Gemini in Google Meet. Through video lessons, hands-on activities and practical examples, you will gain a comprehensive understanding of the Gemini features in Google Meet. You learn how to use Gemini to generate background images, improve your video quality, and translate captions. By the end of this course, you'll be equipped with the knowledge and skills to confidently utilize Gemini in Google Meet to maximize the effectiveness of your video conferences.
Gemini for Google Workspace provides customers with generative AI features in Google Workspace. In this mini-course, you learn about the key features of Gemini and how they can be used to improve productivity and efficiency in Google Sheets.
Gemini for Google Workspace provides customers with generative AI features in Google Workspace. In this mini-course, you learn about the key features of Gemini and how they can be used to improve productivity and efficiency in Google Slides.
Gemini for Google Workspace provides customers with access to generative AI features. This course delves into the capabilities of Gemini in Google Docs using video lessons, hands-on activities and practical examples. You learn how to use Gemini to generate written content based on prompts. You also explore using Gemini to edit text you’ve already written, helping you improve your overall productivity. By the end of this course, you'll be equipped with the knowledge and skills to confidently utilize Gemini in Google Docs to improve your writing.
Gemini for Google Workspace provides customers with generative AI features in Google Workspace. In this mini-course, you learn about the key features of Gemini and how they can be used to improve productivity and efficiency in Gmail.
Gemini for Google Workspace provides customers with generative AI features in Google Workspace. In this learning path, you learn about the key features of Gemini and how they can be used to improve productivity and efficiency in Google Workspace.
In this second installment of the Dataflow course series, we are going to be diving deeper on developing pipelines using the Beam SDK. We start with a review of Apache Beam concepts. Next, we discuss processing streaming data using windows, watermarks and triggers. We then cover options for sources and sinks in your pipelines, schemas to express your structured data, and how to do stateful transformations using State and Timer APIs. We move onto reviewing best practices that help maximize your pipeline performance. Towards the end of the course, we introduce SQL and Dataframes to represent your business logic in Beam and how to iteratively develop pipelines using Beam notebooks.
This course explores what ML is and what problems it can solve. The course also discusses best practices for implementing machine learning. You’re introduced to Vertex AI, a unified platform to quickly build, train, and deploy AutoML machine learning models. The course discusses the five phases of converting a candidate use case to be driven by machine learning, and why it’s important to not skip them. The course ends with recognizing the biases that ML can amplify and how to recognize them.
This course helps learners create a study plan for the PDE (Professional Data Engineer) certification exam. Learners explore the breadth and scope of the domains covered in the exam. Learners assess their exam readiness and create their individual study plan.
This course is part 1 of a 3-course series on Serverless Data Processing with Dataflow. In this first course, we start with a refresher of what Apache Beam is and its relationship with Dataflow. Next, we talk about the Apache Beam vision and the benefits of the Beam Portability framework. The Beam Portability framework achieves the vision that a developer can use their favorite programming language with their preferred execution backend. We then show you how Dataflow allows you to separate compute and storage while saving money, and how identity, access, and management tools interact with your Dataflow pipelines. Lastly, we look at how to implement the right security model for your use case on Dataflow.
Incorporating machine learning into data pipelines increases the ability to extract insights from data. This course covers ways machine learning can be included in data pipelines on Google Cloud. For little to no customization, this course covers AutoML. For more tailored machine learning capabilities, this course introduces Notebooks and BigQuery machine learning (BigQuery ML). Also, this course covers how to productionalize machine learning solutions by using Vertex AI.
Processing streaming data is becoming increasingly popular as streaming enables businesses to get real-time metrics on business operations. This course covers how to build streaming data pipelines on Google Cloud. Pub/Sub is described for handling incoming streaming data. The course also covers how to apply aggregations and transformations to streaming data using Dataflow, and how to store processed records to BigQuery or Bigtable for analysis. Learners get hands-on experience building streaming data pipeline components on Google Cloud by using QwikLabs.
The two key components of any data pipeline are data lakes and warehouses. This course highlights use-cases for each type of storage and dives into the available data lake and warehouse solutions on Google Cloud in technical detail. Also, this course describes the role of a data engineer, the benefits of a successful data pipeline to business operations, and examines why data engineering should be done in a cloud environment. This is the first course of the Data Engineering on Google Cloud series. After completing this course, enroll in the Building Batch Data Pipelines on Google Cloud course.
Complete the intermediate Engineer Data for Predictive Modeling with BigQuery ML skill badge to demonstrate skills in the following: building data transformation pipelines to BigQuery using Dataprep by Trifacta; using Cloud Storage, Dataflow, and BigQuery to build extract, transform, and load (ETL) workflows; and building machine learning models using BigQuery ML. A skill badge is an exclusive digital badge issued by Google Cloud in recognition of your proficiency with Google Cloud products and services and tests your ability to apply your knowledge in an interactive hands-on environment. Complete the skill badge course, and final assessment challenge lab, to receive a digital badge that you can share with your network.
In this course, you learn how to create APIs that utilize multiple services and how you can use custom code on Apigee. You will also learn about fault handling, and how to share logic between proxies. You learn about traffic management and caching. You also create a developer portal, and publish your API to the portal. You learn about logging and analytics, as well as CI/CD and the different deployment models supported by Apigee. Through a combination of lectures, hands-on labs, and supplemental materials, you will learn how to design, build, secure, deploy, and manage API solutions using Google Cloud's Apigee API Platform.This is the third and final course of the Developing APIs with Google Cloud's Apigee API Platform course series.
In this course, you learn how to secure your APIs. You explore the security concerns you will encounter for your APIs. You learn about OAuth, the primary authorization method for REST APIs. You will learn about JSON Web Tokens (JWTs) and federated security. You also learn about securing against malicious requests, safely sending requests across a public network, and how to secure your data for users of Apigee. Through a combination of lectures, hands-on labs, and supplemental materials, you will learn how to design, build, secure, deploy, and manage API solutions using Google Cloud's Apigee API Platform. This is the second course of the Developing APIs with Google Cloud's Apigee API Platform series. After completing this course, enroll in the API Development on Google Cloud's Apigee API Platform course.
In this course, you learn how to design APIs, and how to use OpenAPI specifications to document them. You learn about the API life cycle, and how the Apigee API platform helps you manage all aspects of the life cycle. You learn about how APIs can be designed using API proxies, and how APIs are packaged as API products to be used by app developers. Through a combination of lectures, hands-on labs, and supplemental materials, you will learn how to design, build, secure, deploy, and manage API solutions using Google Cloud's Apigee API Platform. This is the first course of the Developing APIs with Google Cloud's Apigee API Platform series. After completing this course, enroll in the API Security on Google Cloud's Apigee API Platform course.
Під час курсу ви зможете ознайомитися з продуктами й сервісами Google Cloud для роботи з масивами даних і машинним навчанням, які підтримують життєвий цикл роботи з даними для тренування моделей штучного інтелекту. У курсі розглядаються процеси, проблеми й переваги створення конвеєру масиву даних і моделей машинного навчання з Vertex AI у Google Cloud.
Щоб отримати кваліфікаційний значок, пройдіть курс Налаштування середовища для розробки додатка в Google Cloud. У ньому ви навчитеся створювати й підключати хмарну інфраструктуру, спрямовану на зберігання даних, за допомогою базових можливостей таких технологій, як Cloud Storage, система керування ідентифікацією і доступом, Cloud Functions та Pub/Sub. Кваліфікаційний значок – це ексклюзивна цифрова відзнака, яка підтверджує, що ви вмієте працювати з продуктами й сервісами Google Cloud, а також застосовувати ці знання в інтерактивному практичному середовищі. Щоб отримати кваліфікаційний значок і показати його колегам, пройдіть цей курс і підсумковий тест.
Пройдіть вступний кваліфікаційний курс Налаштування розподілу навантаження в Compute Engine, щоб продемонструвати свої навички написання команд gcloud і використання Cloud Shell, створення й розгортання віртуальних машин у Compute Engine, а також налаштування мережі й розподілювачів навантаження HTTP. Кваліфікаційний значок – це ексклюзивний цифровий значок від Google Cloud, який засвідчує, що ви знаєтеся на продуктах і сервісах цієї платформи й можете застосовувати ці знання в інтерактивному практичному середовищі. Щоб отримати кваліфікаційний значок і показати його колегам, пройдіть цей курс і підсумковий тест.
Пройдіть квест Create and Manage Cloud Resources й отримайте skill badge. Ви навчитеся виконувати наведені нижче дії. Писати команди gcloud і використовувати Cloud Shell, створювати й розгортати віртуальні машини в Compute Engine, запускати контейнерні додатки за допомогою Google Kubernetes Engine, а також налаштовувати розподілювачі навантаження для мережі й HTTP.Skill badge – це ексклюзивна цифрова винагорода, яка підтверджує, що ви вмієте працювати з продуктами й сервісами Google Cloud, а також застосовувати ці знання в інтерактивному практичному середовищі. Щоб отримати skill badge й показати його колегам, пройдіть цей квест і підсумковий тест.