DAMODAR SELVAM
Mitglied seit 2019
Gold League
8470 Punkte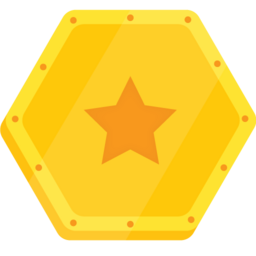
Mitglied seit 2019
Dieser Kurs vermittelt Ihnen eine Zusammenfassung der Encoder-Decoder-Architektur, einer leistungsstarken und gängigen Architektur, die bei Sequenz-zu-Sequenz-Tasks wie maschinellen Übersetzungen, Textzusammenfassungen und dem Question Answering eingesetzt wird. Sie lernen die Hauptkomponenten der Encoder-Decoder-Architektur kennen und erfahren, wie Sie diese Modelle trainieren und bereitstellen können. Im dazugehörigen Lab mit Schritt-für-Schritt-Anleitung können Sie in TensorFlow von Grund auf einen Code für eine einfache Implementierung einer Encoder-Decoder-Architektur erstellen, die zum Schreiben von Gedichten dient.
In diesem Kurs erfahren Sie, wie Sie mithilfe von Deep Learning ein Modell zur Bilduntertitelung erstellen. Sie lernen die verschiedenen Komponenten eines solchen Modells wie den Encoder und Decoder und die Schritte zum Trainieren und Bewerten des Modells kennen. Nach Abschluss dieses Kurses haben Sie folgende Kompetenzen erworben: Erstellen eigener Modelle zur Bilduntertitelung und Verwenden der Modelle zum Generieren von Untertiteln
In diesem Kurs werden Diffusion-Modelle vorgestellt, eine Gruppe verschiedener Machine Learning-Modelle, die kürzlich einige vielversprechende Fortschritte im Bereich Bildgenerierung gemacht haben. Diffusion-Modelle basieren auf physikalischen Konzepten der Thermodynamik und sind in den letzten Jahren in der Forschung und Industrie sehr beliebt geworden. Dabei stützen sich Diffusion-Modelle auf viele innovative Modelle und Tools zur Bildgenerierung in Google Cloud. In diesem Kurs werden Ihnen die theoretischen Grundlagen der Diffusion-Modelle erläutert und wie Sie diese Modelle über Vertex AI trainieren und bereitstellen können.
In diesem Kurs wird der Aufmerksamkeitsmechanismus vorgestellt. Dies ist ein leistungsstarkes Verfahren, das die Fokussierung neuronaler Netzwerke auf bestimmte Abschnitte einer Eingabesequenz ermöglicht. Sie erfahren, wie der Aufmerksamkeitsmechanismus funktioniert und wie Sie damit die Leistung verschiedener Machine Learning-Tasks wie maschinelle Übersetzungen, Zusammenfassungen von Texten und Question Answering verbessern können.
In diesem Einführungskurs im Microlearning-Format wird untersucht, was Large Language Models (LLM) sind, für welche Anwendungsfälle sie genutzt werden können und wie die LLM-Leistung durch Feinabstimmung von Prompts gesteigert werden kann. Darüber hinaus werden Tools von Google behandelt, die das Entwickeln eigener Anwendungen basierend auf generativer KI ermöglichen.
In diesem Einführungskurs im Microlearning-Format wird erklärt, was generative KI ist, wie sie genutzt wird und wie sie sich von herkömmlichen Methoden für Machine Learning unterscheidet. Darüber hinaus werden Tools von Google behandelt, mit denen Sie eigene Anwendungen basierend auf generativer KI entwickeln können.
This advanced-level quest is unique amongst the other catalog offerings. The labs have been curated to give IT professionals hands-on practice with topics and services that appear in the Google Cloud Certified Professional Data Engineer Certification. From Big Query, to Dataprep, to Cloud Composer, this quest is composed of specific labs that will put your Google Cloud data engineering knowledge to the test. Be aware that while practice with these labs will increase your skills and abilities, you will need other preparation, too. The exam is quite challenging and external studying, experience, and/or background in cloud data engineering is recommended. Looking for a hands on challenge lab to demonstrate your skills and validate your knowledge? On completing this quest, enroll in and finish the additional challenge lab at the end of the Engineer Data in the Google Cloud to receive an exclusive Google Cloud digital badge.
Incorporating machine learning into data pipelines increases the ability to extract insights from data. This course covers ways machine learning can be included in data pipelines on Google Cloud. For little to no customization, this course covers AutoML. For more tailored machine learning capabilities, this course introduces Notebooks and BigQuery machine learning (BigQuery ML). Also, this course covers how to productionalize machine learning solutions by using Vertex AI.
Processing streaming data is becoming increasingly popular as streaming enables businesses to get real-time metrics on business operations. This course covers how to build streaming data pipelines on Google Cloud. Pub/Sub is described for handling incoming streaming data. The course also covers how to apply aggregations and transformations to streaming data using Dataflow, and how to store processed records to BigQuery or Bigtable for analysis. Learners get hands-on experience building streaming data pipeline components on Google Cloud by using QwikLabs.
Data pipelines typically fall under one of the Extract and Load (EL), Extract, Load and Transform (ELT) or Extract, Transform and Load (ETL) paradigms. This course describes which paradigm should be used and when for batch data. Furthermore, this course covers several technologies on Google Cloud for data transformation including BigQuery, executing Spark on Dataproc, pipeline graphs in Cloud Data Fusion and serverless data processing with Dataflow. Learners get hands-on experience building data pipeline components on Google Cloud using Qwiklabs.
This quest of "Challenge Labs" gives the student preparing for the Google Cloud Certified Professional Cloud Architect certification hands-on practice with common business/technology solutions using Google Cloud architectures. Challenge Labs do not provide the "cookbook" steps, but require solutions to be built with minimal guidance, across many Google Cloud technologies. All labs have activity tracking, and in order to earn this badge you must score 100% in each lab. This quest is not easy and will put your Google Cloud technology skills to the test! Be aware that while practice with these labs will increase your knowledge and abilities, additional study, experience, and background in cloud architecture is recommended to prepare for this certification. Complete this quest to receive an exclusive Google Cloud digital badge.
The two key components of any data pipeline are data lakes and warehouses. This course highlights use-cases for each type of storage and dives into the available data lake and warehouse solutions on Google Cloud in technical detail. Also, this course describes the role of a data engineer, the benefits of a successful data pipeline to business operations, and examines why data engineering should be done in a cloud environment. This is the first course of the Data Engineering on Google Cloud series. After completing this course, enroll in the Building Batch Data Pipelines on Google Cloud course.
In this introductory-level course, you get hands-on practice with the Google Cloud’s fundamental tools and services. Optional videos are provided to provide more context and review for the concepts covered in the labs. Google Cloud Essentials is a recommendeded first course for the Google Cloud learner - you can come in with little or no prior cloud knowledge, and come out with practical experience that you can apply to your first Google Cloud project. From writing Cloud Shell commands and deploying your first virtual machine, to running applications on Kubernetes Engine or with load balancing, Google Cloud Essentials is a prime introduction to the platform’s basic features.
This course introduces the Google Cloud big data and machine learning products and services that support the data-to-AI lifecycle. It explores the processes, challenges, and benefits of building a big data pipeline and machine learning models with Vertex AI on Google Cloud.
This fundamental-level quest is unique amongst the other quest offerings. The labs have been curated to give IT professionals hands-on practice with topics and services that appear in the Google Cloud Certified Professional Cloud Architect Certification. From IAM, to networking, to Kubernetes engine deployment, this quest is composed of specific labs that will put your Google Cloud knowledge to the test. Be aware that while practice with these labs will increase your skills and abilities, we recommend that you also review the exam guide and other available preparation resources.
This course version is for non-English only. If you wish to take this course in English, please enroll here: Elastic Google Cloud Infrastructure: Scaling and Automation. If you wish to take it in another language, change your language in settings to see availability.
This accelerated on-demand course introduces participants to the comprehensive and flexible infrastructure and platform services provided by Google Cloud with a focus on Compute Engine. Through a combination of video lectures, demos, and hands-on labs, participants explore and deploy solution elements, including infrastructure components such as networks, virtual machines and applications services. You will learn how to use the Google Cloud through the console and Cloud Shell. You'll also learn about the role of a cloud architect, approaches to infrastructure design, and virtual networking configuration with Virtual Private Cloud (VPC), Projects, Networks, Subnetworks, IP addresses, Routes, and Firewall rules.
This accelerated on-demand course introduces participants to the comprehensive and flexible infrastructure and platform services provided by Google Cloud with a focus on Compute Engine. Through a combination of video lectures, demos, and hands-on labs, participants explore and deploy solution elements, including infrastructure components such as networks, systems and applications services. This course also covers deploying practical solutions including customer-supplied encryption keys, security and access management, quotas and billing, and resource monitoring.
This course equips students to build highly reliable and efficient solutions on Google Cloud using proven design patterns. It is a continuation of the Architecting with Google Compute Engine or Architecting with Google Kubernetes Engine courses and assumes hands-on experience with the technologies covered in either of those courses. Through a combination of presentations, design activities, and hands-on labs, participants learn to define and balance business and technical requirements to design Google Cloud deployments that are highly reliable, highly available, secure, and cost-effective.
This content is deprecated. Please see the latest version of the course, here.