DAMODAR SELVAM
成为会员时间:2019
黄金联赛
8470 积分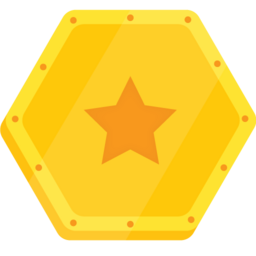
成为会员时间:2019
本课程简要介绍了编码器-解码器架构,这是一种功能强大且常见的机器学习架构,适用于机器翻译、文本摘要和问答等 sequence-to-sequence 任务。您将了解编码器-解码器架构的主要组成部分,以及如何训练和部署这些模型。在相应的实验演示中,您将在 TensorFlow 中从头编写简单的编码器-解码器架构实现代码,以用于诗歌生成。
本课程教您如何使用深度学习来创建图片标注模型。您将了解图片标注模型的不同组成部分,例如编码器和解码器,以及如何训练和评估模型。学完本课程,您将能够自行创建图片标注模型并用来生成图片说明。
本课程向您介绍扩散模型。这类机器学习模型最近在图像生成领域展现出了巨大潜力。扩散模型的灵感来源于物理学,特别是热力学。过去几年内,扩散模型成为热门研究主题并在整个行业开始流行。Google Cloud 上许多先进的图像生成模型和工具都是以扩散模型为基础构建的。本课程向您介绍扩散模型背后的理论,以及如何在 Vertex AI 上训练和部署此类模型。
本课程将向您介绍注意力机制,这是一种强大的技术,可令神经网络专注于输入序列的特定部分。您将了解注意力的工作原理,以及如何使用它来提高各种机器学习任务的性能,包括机器翻译、文本摘要和问题解答。
这是一节入门级微学习课程,探讨什么是大型语言模型 (LLM)、适合的应用场景以及如何使用提示调整来提升 LLM 性能,还介绍了可以帮助您开发自己的 Gen AI 应用的各种 Google 工具。
这是一节入门级微课程,旨在解释什么是生成式 AI、它的用途以及与传统机器学习方法的区别。该课程还介绍了可以帮助您开发自己的生成式 AI 应用的各种 Google 工具。
This advanced-level quest is unique amongst the other catalog offerings. The labs have been curated to give IT professionals hands-on practice with topics and services that appear in the Google Cloud Certified Professional Data Engineer Certification. From Big Query, to Dataprep, to Cloud Composer, this quest is composed of specific labs that will put your Google Cloud data engineering knowledge to the test. Be aware that while practice with these labs will increase your skills and abilities, you will need other preparation, too. The exam is quite challenging and external studying, experience, and/or background in cloud data engineering is recommended. Looking for a hands on challenge lab to demonstrate your skills and validate your knowledge? On completing this quest, enroll in and finish the additional challenge lab at the end of the Engineer Data in the Google Cloud to receive an exclusive Google Cloud digital badge.
Incorporating machine learning into data pipelines increases the ability to extract insights from data. This course covers ways machine learning can be included in data pipelines on Google Cloud. For little to no customization, this course covers AutoML. For more tailored machine learning capabilities, this course introduces Notebooks and BigQuery machine learning (BigQuery ML). Also, this course covers how to productionalize machine learning solutions by using Vertex AI.
Processing streaming data is becoming increasingly popular as streaming enables businesses to get real-time metrics on business operations. This course covers how to build streaming data pipelines on Google Cloud. Pub/Sub is described for handling incoming streaming data. The course also covers how to apply aggregations and transformations to streaming data using Dataflow, and how to store processed records to BigQuery or Bigtable for analysis. Learners get hands-on experience building streaming data pipeline components on Google Cloud by using QwikLabs.
Data pipelines typically fall under one of the Extract and Load (EL), Extract, Load and Transform (ELT) or Extract, Transform and Load (ETL) paradigms. This course describes which paradigm should be used and when for batch data. Furthermore, this course covers several technologies on Google Cloud for data transformation including BigQuery, executing Spark on Dataproc, pipeline graphs in Cloud Data Fusion and serverless data processing with Dataflow. Learners get hands-on experience building data pipeline components on Google Cloud using Qwiklabs.
This quest of "Challenge Labs" gives the student preparing for the Google Cloud Certified Professional Cloud Architect certification hands-on practice with common business/technology solutions using Google Cloud architectures. Challenge Labs do not provide the "cookbook" steps, but require solutions to be built with minimal guidance, across many Google Cloud technologies. All labs have activity tracking, and in order to earn this badge you must score 100% in each lab. This quest is not easy and will put your Google Cloud technology skills to the test! Be aware that while practice with these labs will increase your knowledge and abilities, additional study, experience, and background in cloud architecture is recommended to prepare for this certification. Complete this quest to receive an exclusive Google Cloud digital badge.
The two key components of any data pipeline are data lakes and warehouses. This course highlights use-cases for each type of storage and dives into the available data lake and warehouse solutions on Google Cloud in technical detail. Also, this course describes the role of a data engineer, the benefits of a successful data pipeline to business operations, and examines why data engineering should be done in a cloud environment. This is the first course of the Data Engineering on Google Cloud series. After completing this course, enroll in the Building Batch Data Pipelines on Google Cloud course.
在此入门级挑战任务中,您可以使用 Google Cloud Platform 的基本工具和服务,开展真枪实弹的操作实训。“GCP 基本功能”是我们为 Google Cloud 学员推荐的第一项挑战任务。云知识储备微乎其微甚至零基础?不用担心!这项挑战任务会为您提供真枪实弹的实操经验,助您快速上手 GCP 项目。无论是要编写 Cloud Shell 命令还是部署您的第一台虚拟机,亦或是通过负载平衡机制或在 Kubernetes Engine 上运行应用,都可以通过“GCP 基本功能”了解该平台的基本功能之精要。点此观看 1 分钟视频,了解每个实验涉及的主要概念。
This course introduces the Google Cloud big data and machine learning products and services that support the data-to-AI lifecycle. It explores the processes, challenges, and benefits of building a big data pipeline and machine learning models with Vertex AI on Google Cloud.
This fundamental-level quest is unique amongst the other quest offerings. The labs have been curated to give IT professionals hands-on practice with topics and services that appear in the Google Cloud Certified Professional Cloud Architect Certification. From IAM, to networking, to Kubernetes engine deployment, this quest is composed of specific labs that will put your Google Cloud knowledge to the test. Be aware that while practice with these labs will increase your skills and abilities, we recommend that you also review the exam guide and other available preparation resources.
This course version is for non-English only. If you wish to take this course in English, please enroll here: Elastic Google Cloud Infrastructure: Scaling and Automation. If you wish to take it in another language, change your language in settings to see availability.
这门自助式速成课程向学员介绍 Google Cloud 提供的灵活全面的基础架构和平台服务,其中着重介绍了 Compute Engine。学员将通过一系列视频讲座、演示和动手实验,探索和部署各种解决方案元素,包括网络、虚拟机和应用服务等基础架构组件。您将学习如何通过控制台和 Cloud Shell 使用 Google Cloud。您还将了解云架构师角色、基础架构设计方法以及虚拟网络配置和虚拟私有云 (VPC)、项目、网络、子网、IP 地址、路由及防火墙规则。
这门自助式速成课程向学员介绍 Google Cloud 提供的灵活全面的基础架构和平台服务,着重介绍了 Compute Engine。学员将通过一系列视频讲座、演示和动手实验,探索和部署各种解决方案元素,包括网络、系统和应用服务等基础架构组件。本课程的内容还包括如何部署实用的解决方案,包括客户提供的加密密钥、安全和访问权限管理、配额和结算,以及资源监控。
本课程指导学员运用久经考验的设计模式在 Google Cloud 上构建高度可靠且高效的解决方案。它是“Google Compute Engine 架构设计”或“Google Kubernetes Engine 架构设计”课程的延续,并假定您有使用其中任何一门课程所涵盖技术的实践经验。通过一系列演示、设计活动和动手实验,学员可以了解如何定义及平衡业务要求和技术要求,以便设计可靠性和可用性高、安全且经济实惠的 Google Cloud 部署。
This content is deprecated. Please see the latest version of the course, here.