Carlos Guerreiro
成为会员时间:2023
钻石联赛
19385 积分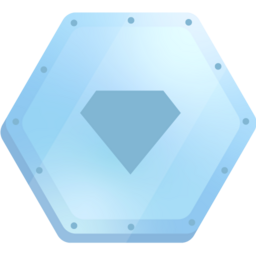
成为会员时间:2023
This course empowers you to develop scalable, performant LookML (Looker Modeling Language) models that provide your business users with the standardized, ready-to-use data that they need to answer their questions. Upon completing this course, you will be able to start building and maintaining LookML models to curate and manage data in your organization’s Looker instance.
In this quest, you will get hands-on experience with LookML in Looker. You will learn how to write LookML code to create new dimensions and measures, create derived tables and join them to Explores, filter Explores, and define caching policies in LookML.
完成“为 Looker 信息中心和报告准备数据”入门级技能徽章课程,展现以下方面的技能: 对数据进行过滤、排序和透视,将来自不同 Looker 探索的结果合并,以及使用函数和运算符构建 Looker 信息中心和报告以用于数据分析和可视化。 技能徽章是由 Google Cloud 颁发的专属数字徽章,旨在认可您在 Google Cloud 产品与服务方面的熟练度;您需要在交互式实操环境中参加考核, 证明自己运用所学知识的能力后才能获得。完成此技能徽章课程和作为最终评估的实验室挑战赛,获得技能徽章,在您的人际圈中炫出自己的技能。
完成入门级技能徽章课程在 Google Cloud 上为机器学习 API 准备数据,展示以下技能: 使用 Dataprep by Trifacta 清理数据、在 Dataflow 中运行数据流水线、在 Dataproc 中创建集群和运行 Apache Spark 作业,以及调用机器学习 API,包括 Cloud Natural Language API、Google Cloud Speech-to-Text API 和 Video Intelligence API。 技能徽章是由 Google Cloud 颁发的专属数字徽章,旨在认可您在 Google Cloud 产品与服务方面的熟练度; 您需要在交互式实操环境中参加考核,证明自己运用所学知识的能力后才能获得。完成此技能徽章课程和作为最终评估的实验室挑战赛, 获得技能徽章,在您的人际圈中炫出自己的技能。
“Google Cloud 基础知识:核心基础架构”介绍在使用 Google Cloud 时会遇到的重要概念和术语。本课程通过视频和实操实验来介绍并比较 Google Cloud 的多种计算和存储服务,并提供重要的资源和政策管理工具。
Earn a skill badge by completing the Cloud Run functions: 3 Ways course, where you learn how to use Cloud Run functions (including 2nd gen) through the Google Cloud console and on the command line. A skill badge is an exclusive digital badge issued by Google Cloud in recognition of your proficiency with Google Cloud products and services. It tests your ability to apply your knowledge in an interactive hands-on environment. Complete this skill badge course, including the final assessment challenge lab, to receive a digital badge that you can share with your network.
This course covers BigQuery fundamentals for professionals who are familiar with SQL-based cloud data warehouses in Redshift and want to begin working in BigQuery. Through interactive lecture content and hands-on labs, you learn how to provision resources, create and share data assets, ingest data, and optimize query performance in BigQuery. Drawing upon your knowledge of Redshift, you also learn about similarities and differences between Redshift and BigQuery to help you get started with data warehouses in BigQuery. After this course, you can continue your BigQuery journey by completing the skill badge quest titled Build and Optimize Data Warehouses with BigQuery.
完成“在 Google Cloud 上设置应用开发环境”课程,赢取技能徽章;通过该课程,您将了解如何使用以下技术的基本功能来构建和连接以存储为中心的云基础设施: Cloud Storage、Identity and Access Management、Cloud Functions 和 Pub/Sub。 技能徽章是由 Google Cloud 颁发的专属数字徽章, 旨在认可您在 Google Cloud 产品与服务方面的熟练度;您需要在交互式实操环境中参加考核,证明自己运用所学知识的能力后才能获得。完成此技能徽章课程和作为最终评估的实验室挑战赛,获得技能徽章,在您的人际圈中炫出自己的技能。
Complete the intermediate Optimize Costs for Google Kubernetes Engine skill badge to demonstrate skills in the following: creating and managing multi-tenant clusters, monitoring resource usage by namespace, configuring cluster and pod autoscaling for efficiency, setting up load balancing for optimal resource distribution, and implementing liveness and readiness probes to ensure application health and cost-effectiveness. A skill badge is an exclusive digital badge issued by Google Cloud in recognition of your proficiency with Google Cloud products and services and tests your ability to apply your knowledge in an interactive hands-on environment. Complete this skill badge course and the final assessment challenge lab to receive a skill badge that you can share with your network.
完成Implement Cloud Security Fundamentals on Google Cloud技能徽章中级课程, 展示自己在以下方面的技能:使用 Identity and Access Management (IAM) 创建和分配角色; 创建和管理服务账号;跨虚拟私有云 (VPC) 网络实现专用连接; 使用 Identity-Aware Proxy 限制应用访问权限; 使用 Cloud Key Management Service (KMS) 管理密钥和加密数据;创建专用 Kubernetes 集群。 技能徽章是由 Google Cloud 颁发的专有数字徽章,旨在认可 您在 Google Cloud 产品与服务方面的熟练度;您需要在 交互式实操环境中参加考核,证明自己运用所学知识的能力后才能获得。完成此技能 徽章课程和作为最终评估的实验室挑战赛,即可获得技能徽章, 并在您的社交圈中秀一秀自己的能力。
In this course, you learn how to do the kind of data exploration and analysis in Looker that would formerly be done primarily by SQL developers or analysts. Upon completion of this course, you will be able to leverage Looker's modern analytics platform to find and explore relevant content in your organization’s Looker instance, ask questions of your data, create new metrics as needed, and build and share visualizations and dashboards to facilitate data-driven decision making.
This course teaches participants techniques for monitoring and improving infrastructure and application performance in Google Cloud. Using a combination of presentations, demos, hands-on labs, and real-world case studies, attendees gain experience with full-stack monitoring, real-time log management and analysis, debugging code in production, tracing application performance bottlenecks, and profiling CPU and memory usage.
The Generative AI Explorer - Vertex Quest is a collection of labs on how to use Generative AI on Google Cloud. Through the labs, you will learn about how to use the models in the Vertex AI PaLM API family, including text-bison, chat-bison, and textembedding-gecko. You will also learn about prompt design, best practices, and how it can be used for ideation, text classification, text extraction, text summarization, and more. You will also learn how to tune a foundation model by training it via Vertex AI custom training and deploy it to a Vertex AI endpoint.
本课程指导学员运用久经考验的设计模式在 Google Cloud 上构建高度可靠且高效的解决方案。它是“Google Compute Engine 架构设计”或“Google Kubernetes Engine 架构设计”课程的延续,并假定您有使用其中任何一门课程所涵盖技术的实践经验。通过一系列演示、设计活动和动手实验,学员可以了解如何定义及平衡业务要求和技术要求,以便设计可靠性和可用性高、安全且经济实惠的 Google Cloud 部署。
随着企业对人工智能和机器学习的应用越来越广泛,以负责任的方式构建这些技术也变得更加重要。但对很多企业而言,真正践行 Responsible AI 并非易事。如果您有意了解如何在组织内践行 Responsible AI,本课程正适合您。 本课程将介绍 Google Cloud 目前如何践行 Responsible AI,以及从中总结的最佳实践和经验教训,便于您以此为框架构建自己的 Responsible AI 方法。
The course begins with a discussion about data: how to improve data quality and perform exploratory data analysis. We describe Vertex AI AutoML and how to build, train, and deploy an ML model without writing a single line of code. You will understand the benefits of Big Query ML. We then discuss how to optimize a machine learning (ML) model and how generalization and sampling can help assess the quality of ML models for custom training.
This course explores what ML is and what problems it can solve. The course also discusses best practices for implementing machine learning. You’re introduced to Vertex AI, a unified platform to quickly build, train, and deploy AutoML machine learning models. The course discusses the five phases of converting a candidate use case to be driven by machine learning, and why it’s important to not skip them. The course ends with recognizing the biases that ML can amplify and how to recognize them.
本课程介绍 Vertex AI Studio,这是一种用于生成式 AI 模型原型设计和自定义的工具。通过沉浸式课程、互动式演示和实操实验,您将探索生成式 AI 工作流,了解如何将 Vertex AI Studio 用于多模态 Gemini 应用、提示设计和模型调优。本课程的目的在于帮助您利用 Vertex AI Studio,在自己的项目中充分发掘这些模型的潜力。
本课程教您如何使用深度学习来创建图片标注模型。您将了解图片标注模型的不同组成部分,例如编码器和解码器,以及如何训练和评估模型。学完本课程,您将能够自行创建图片标注模型并用来生成图片说明。
本课程向您介绍 Transformer 架构和 Bidirectional Encoder Representations from Transformers (BERT) 模型。您将了解 Transformer 架构的主要组成部分,例如自注意力机制,以及该架构如何用于构建 BERT 模型。您还将了解可以使用 BERT 的不同任务,例如文本分类、问答和自然语言推理。完成本课程估计需要大约 45 分钟。
本课程将向您介绍注意力机制,这是一种强大的技术,可令神经网络专注于输入序列的特定部分。您将了解注意力的工作原理,以及如何使用它来提高各种机器学习任务的性能,包括机器翻译、文本摘要和问题解答。
本课程简要介绍了编码器-解码器架构,这是一种功能强大且常见的机器学习架构,适用于机器翻译、文本摘要和问答等 sequence-to-sequence 任务。您将了解编码器-解码器架构的主要组成部分,以及如何训练和部署这些模型。在相应的实验演示中,您将在 TensorFlow 中从头编写简单的编码器-解码器架构实现代码,以用于诗歌生成。
本课程向您介绍扩散模型。这类机器学习模型最近在图像生成领域展现出了巨大潜力。扩散模型的灵感来源于物理学,特别是热力学。过去几年内,扩散模型成为热门研究主题并在整个行业开始流行。Google Cloud 上许多先进的图像生成模型和工具都是以扩散模型为基础构建的。本课程向您介绍扩散模型背后的理论,以及如何在 Vertex AI 上训练和部署此类模型。
完成 Introduction to Generative AI、Introduction to Large Language Models 和 Introduction to Responsible AI 三门课程,赢取技能徽章。通过最终测验,即表明您理解了生成式 AI 的基本概念。 技能徽章是由 Google Cloud 颁发的数字徽章,旨在认可您对 Google Cloud 产品与服务的了解程度。公开您的个人资料并将技能徽章添加到您的社交媒体个人资料中,以此来分享您获得的成就。
这是一节入门级微课程,旨在解释什么是负责任的 AI、它的重要性,以及 Google 如何在自己的产品中实现负责任的 AI。此外,本课程还介绍了 Google 的 7 个 AI 开发原则。
这是一节入门级微学习课程,探讨什么是大型语言模型 (LLM)、适合的应用场景以及如何使用提示调整来提升 LLM 性能,还介绍了可以帮助您开发自己的 Gen AI 应用的各种 Google 工具。
这是一节入门级微课程,旨在解释什么是生成式 AI、它的用途以及与传统机器学习方法的区别。该课程还介绍了可以帮助您开发自己的生成式 AI 应用的各种 Google 工具。
This course is part 1 of a 3-course series on Serverless Data Processing with Dataflow. In this first course, we start with a refresher of what Apache Beam is and its relationship with Dataflow. Next, we talk about the Apache Beam vision and the benefits of the Beam Portability framework. The Beam Portability framework achieves the vision that a developer can use their favorite programming language with their preferred execution backend. We then show you how Dataflow allows you to separate compute and storage while saving money, and how identity, access, and management tools interact with your Dataflow pipelines. Lastly, we look at how to implement the right security model for your use case on Dataflow.
Incorporating machine learning into data pipelines increases the ability to extract insights from data. This course covers ways machine learning can be included in data pipelines on Google Cloud. For little to no customization, this course covers AutoML. For more tailored machine learning capabilities, this course introduces Notebooks and BigQuery machine learning (BigQuery ML). Also, this course covers how to productionalize machine learning solutions by using Vertex AI.
Processing streaming data is becoming increasingly popular as streaming enables businesses to get real-time metrics on business operations. This course covers how to build streaming data pipelines on Google Cloud. Pub/Sub is described for handling incoming streaming data. The course also covers how to apply aggregations and transformations to streaming data using Dataflow, and how to store processed records to BigQuery or Bigtable for analysis. Learners get hands-on experience building streaming data pipeline components on Google Cloud by using QwikLabs.
Data pipelines typically fall under one of the Extract and Load (EL), Extract, Load and Transform (ELT) or Extract, Transform and Load (ETL) paradigms. This course describes which paradigm should be used and when for batch data. Furthermore, this course covers several technologies on Google Cloud for data transformation including BigQuery, executing Spark on Dataproc, pipeline graphs in Cloud Data Fusion and serverless data processing with Dataflow. Learners get hands-on experience building data pipeline components on Google Cloud using Qwiklabs.
This course helps learners create a study plan for the PDE (Professional Data Engineer) certification exam. Learners explore the breadth and scope of the domains covered in the exam. Learners assess their exam readiness and create their individual study plan.
The two key components of any data pipeline are data lakes and warehouses. This course highlights use-cases for each type of storage and dives into the available data lake and warehouse solutions on Google Cloud in technical detail. Also, this course describes the role of a data engineer, the benefits of a successful data pipeline to business operations, and examines why data engineering should be done in a cloud environment. This is the first course of the Data Engineering on Google Cloud series. After completing this course, enroll in the Building Batch Data Pipelines on Google Cloud course.
This course introduces the Google Cloud big data and machine learning products and services that support the data-to-AI lifecycle. It explores the processes, challenges, and benefits of building a big data pipeline and machine learning models with Vertex AI on Google Cloud.
完成为 AWS 专业人员构建 Google Cloud 基础设施课程, 赢取技能徽章。在此课程中,您将学习如何配置 IAM 权限, 使用 Kubernetes 编排工作负载,使用 Compute Engine 托管 Web 应用, 以及配置负载均衡。 技能徽章是由 Google Cloud 颁发的专属数字徽章, 旨在认可您在 Google Cloud 产品与服务方面的熟练度。 您需要在交互式实操环境中参加考核,证明自己运用所学知识的能力后 才能获得此徽章。完成此技能徽章课程和作为最终评估的实验室挑战赛, 获得数字徽章,在您的人际圈中秀出自己的技能。
This is the fourth course of a four-course series for cloud architects and engineers with existing AWS knowledge. It compares Google Cloud and AWS solutions and guides professionals on their use. This course focuses on deploying and monitoring applications in Google Cloud. The learners apply the knowledge of monitoring and application deployment processes in AWS to explore the differences with Google Cloud. Learners get hands-on practice building and managing Google Cloud resources.
This is the third course of a four-course series for cloud architects and engineers with existing AWS knowledge, and it compares Google Cloud and AWS solutions and guides professionals on their use. This course focuses on Storage Options and containers in Google Cloud. The learners apply the knowledge of storage and containers in AWS to explore the similarities and differences with storage and containers in Google Cloud. Learners get hands-on practice building and managing Google Cloud resources.
This is the second course of a four-course series for cloud architects and engineers with existing AWS knowledge. It aims to compare Google Cloud and AWS solutions and guide professionals on their use. This course focuses on compute resources and load balancing in Google Cloud. The learner will apply the knowledge of using virtual machines and load balancers in AWS to explore the similarities and differences with configuring and managing compute resources and load balancers in Google Cloud. Learners will get hands-on practice building and managing Google Cloud resources.
This is the first course of a four-course series for cloud architects and engineers with existing AWS knowledge, and it compares Google Cloud and AWS solutions and guides professionals on their use. This course focuses on Identity and Access Management (IAM) and networking in Google Cloud. The learners apply the knowledge of access management and networking in AWS to explore the similarities and differences with access management and networking in Google Cloud. Learners get hands-on practice building and managing Google Cloud resources.