Pieter Geelen
Member since 2023
Gold League
18880 points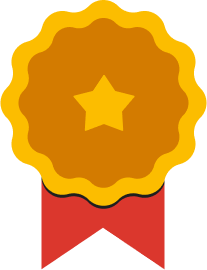
Member since 2023
In many IT organizations, incentives are not aligned between developers, who strive for agility, and operators, who focus on stability. Site reliability engineering, or SRE, is how Google aligns incentives between development and operations and does mission-critical production support. Adoption of SRE cultural and technical practices can help improve collaboration between the business and IT. This course introduces key practices of Google SRE and the important role IT and business leaders play in the success of SRE organizational adoption.
Google Cloud Fundamentals: Core Infrastructure introduces important concepts and terminology for working with Google Cloud. Through videos and hands-on labs, this course presents and compares many of Google Cloud's computing and storage services, along with important resource and policy management tools.
This course helps learners create a study plan for the PDE (Professional Data Engineer) certification exam. Learners explore the breadth and scope of the domains covered in the exam. Learners assess their exam readiness and create their individual study plan.
הקורס בוחן ניהול עלויות, אבטחה ותפעול בענן. ראשית, מוסבר איך עסקים יכולים לרכוש שירותי IT מספק שירותי ענן ולשמר חלק מהתשתית שלהם או לבחור לא לשמר אותה בכלל. שנית, הקורס מתאר איך האחריות על אבטחת נתונים מתחלקת בין ספק שירותי הענן לעסק, וסוקר את אבטחת ההגנה לעומק (defense-in-depth) שמובנית ב-Google Cloud. לבסוף, הקורס מתייחס לכך שצוותי IT ומנהלי העסק צריכים לשנות את החשיבה על ניהול משאבי IT בענן, ונוגע באופן שבו כלי ניטור המשאבים ב-Google Cloud יכולים לסייע להם לשמור על שליטה וניראות בסביבת הענן שלהם.
As organizations move their data and applications to the cloud, they must address new security challenges. The Trust and Security with Google Cloud course explores the basics of cloud security, the value of Google Cloud's multilayered approach to infrastructure security, and how Google earns and maintains customer trust in the cloud. Part of the Cloud Digital Leader learning path, this course aims to help individuals grow in their role and build the future of their business.
בארגונים מסורתיים רבים משתמשים במערכות ובאפליקציות מדורות קודמים, וקשה לבצע באמצעותן התאמה לעומס ופעולות מהירות הדרושות כדי לעמוד בציפיות מודרניות של לקוחות. מנהיגים עסקיים וקובעי מדיניות IT צריכים כל הזמן לבחור בין תחזוקה של מערכות מדורות קודמים לבין השקעה במוצרים ובשירותים חדשים. בקורס הזה נבחן את האתגרים הנובעים משימוש בתשתית IT מיושנת, ואיך בעלי עסקים יכולים לבצע מודרניזציה של תשתיות בעזרת טכנולוגיית ענן. הקורס מתחיל בהבנה מעמיקה של אפשרויות המחשוב השונות הזמינות בענן ופירוט היתרונות של כל אחת מהאפשרויות. לאחר מכן נבחן את האפשרויות למודרניזציה של האפליקציות ושל ממשקי API (ממשק תכנות יישומים). בקורס מתוארים גם מגוון פתרונות של Google Cloud שיכולים לשפר את תהליך פיתוח המערכות וניהולן בעסקים שונים, כמו Compute Engine, App Engine ו-Apigee.
Artificial intelligence (AI) and machine learning (ML) represent an important evolution in information technologies that are quickly transforming a wide range of industries. “Innovating with Google Cloud Artificial Intelligence” explores how organizations can use AI and ML to transform their business processes. Part of the Cloud Digital Leader learning path, this course aims to help individuals grow in their role and build the future of their business.
טכנולוגיית הענן לבדה מספקת לעסק חלק קטן בלבד מהערך האמיתי שלה. כשהיא משולבת עם נתונים בנפח רב מאוד, נוצרת העוצמה שמאפשרת להפיק ערך וליצור חוויות חדשות ללקוחות. במסגרת הקורס הזה תלמדו מהם נתונים, איך השתמשו בהם בעבר בחברות לצורך קבלת החלטות ולמה הם קריטיים כל כך ללמידה חישובית. בנוסף, בקורס הזה יוצגו ללומדים מושגים טכניים כמו נתונים מובְנים ולא מובְנים, מסד נתונים, מחסן נתונים (data warehouse) ואגמי נתונים (data lakes). בהמשך, הקורס יעסוק במוצרי Google Cloud הנפוצים ביותר בתחום הנתונים, ובמוצרים כאלה ששיעור השימוש בהם גדל במהירות הרבה ביותר.
מהי טכנולוגיית ענן ומהו מדע הנתונים? וחשוב יותר, איך הם יכולים לעזור לכם, לצוות שלכם ולעסק שלכם? קורס המבוא הזה בנושא טרנספורמציה דיגיטלית מתאים למי שרוצה ללמוד על טכנולוגיית הענן כדי להתמקצע ולהצטיין בעבודתו וכדי לעזור בפיתוח העתיד של העסק. בקורס יוגדרו מונחי יסוד כגון הענן, נתונים וטרנספורמציה דיגיטלית. בנוסף, נבחן דוגמאות של חברות מרחבי העולם שמשתמשות בטכנולוגיית הענן כדי לבצע טרנספורמציה בעסק. הקורס כולל סקירה של סוגי ההזדמנויות שיש לחברות ושל האתגרים הנפוצים שחברות מתמודדות איתם במהלך טרנספורמציה דיגיטלית. הקורס גם מדגים איך עמודי התווך של פתרונות Google Cloud יכולים לעזור בתהליך. חשוב לומר: טרנספורמציה דיגיטלית לא קשורה רק לשימוש בטכנולוגיות חדשות. כדי הטרנספורמציה תהיה מלאה, ארגונים צריכים גם ליישם חדשנות ולפתח דפוס חשיבה שמקדם חדשנות בכל התחומים והצוותים. השיטות המומלצות המתוארות בקורס יעזרו לכם להשיג את המטרה הזו.
In this course, you learn how to do the kind of data exploration and analysis in Looker that would formerly be done primarily by SQL developers or analysts. Upon completion of this course, you will be able to leverage Looker's modern analytics platform to find and explore relevant content in your organization’s Looker instance, ask questions of your data, create new metrics as needed, and build and share visualizations and dashboards to facilitate data-driven decision making.
בקורס הזה נציג את הארכיטקטורה של טרנספורמרים ואת המודל של ייצוגים דו-כיווניים של מקודד מטרנספורמרים (BERT). תלמדו על החלקים השונים בארכיטקטורת הטרנספורמר, כמו מנגנון תשומת הלב, ועל התפקיד שלו בבניית מודל BERT. תלמדו גם על המשימות השונות שאפשר להשתמש ב-BERT כדי לבצע אותן, כמו סיווג טקסטים, מענה על שאלות והֶקֵּשׁ משפה טבעית. נדרשות כ-45 דקות כדי להשלים את הקורס הזה.
בקורס הזה לומדים בקצרה על ארכיטקטורת מקודד-מפענח, ארכיטקטורה עוצמתית ונפוצה ללמידת מכונה שמשתמשים בה במשימות של רצף לרצף, כמו תרגום אוטומטי, סיכום טקסט ומענה לשאלות. תלמדו על החלקים השונים בארכיטקטורת מקודד-מפענח, איך לאמן את המודלים האלה ואיך להשתמש בהם. בהדרכה המפורטת המשלימה בשיעור ה-Lab תקודדו ב-TensorFlow תרחיש שימוש פשוט בארכיטקטורת מקודד-מפענח: כתיבת שיר מאפס.
בקורס נלמד על מנגנון תשומת הלב, שיטה טובה מאוד שמאפשרת לרשתות נוירונים להתמקד בחלקים ספציפיים ברצף הקלט. נלמד איך עובד העיקרון של תשומת הלב, ואיך אפשר להשתמש בו כדי לשפר את הביצועים במגוון משימות של למידת מכונה, כולל תרגום אוטומטי, סיכום טקסט ומענה לשאלות.
בקורס נלמד על מודלים של דיפוזיה, משפחת מודלים של למידת מכונה שיצרו הרבה ציפיות לאחרונה בתחום של יצירת תמונות. מודלים של דיפוזיה שואבים השראה מפיזיקה, וספציפית מתרמודינמיקה. בשנים האחרונות, מודלים של דיפוזיה הפכו לפופולריים גם בתחום המחקר וגם בתעשייה. מודלים של דיפוזיה עומדים מאחורי הרבה מהכלים והמודלים החדשניים ליצירת תמונות ב-Google Cloud. בקורס הזה נלמד על התיאוריה שמאחורי מודלים של דיפוזיה, ואיך לאמן ולפרוס אותם ב-Vertex AI.
In the last installment of the Dataflow course series, we will introduce the components of the Dataflow operational model. We will examine tools and techniques for troubleshooting and optimizing pipeline performance. We will then review testing, deployment, and reliability best practices for Dataflow pipelines. We will conclude with a review of Templates, which makes it easy to scale Dataflow pipelines to organizations with hundreds of users. These lessons will help ensure that your data platform is stable and resilient to unanticipated circumstances.
In this second installment of the Dataflow course series, we are going to be diving deeper on developing pipelines using the Beam SDK. We start with a review of Apache Beam concepts. Next, we discuss processing streaming data using windows, watermarks and triggers. We then cover options for sources and sinks in your pipelines, schemas to express your structured data, and how to do stateful transformations using State and Timer APIs. We move onto reviewing best practices that help maximize your pipeline performance. Towards the end of the course, we introduce SQL and Dataframes to represent your business logic in Beam and how to iteratively develop pipelines using Beam notebooks.
The course begins with a discussion about data: how to improve data quality and perform exploratory data analysis. We describe Vertex AI AutoML and how to build, train, and deploy an ML model without writing a single line of code. You will understand the benefits of Big Query ML. We then discuss how to optimize a machine learning (ML) model and how generalization and sampling can help assess the quality of ML models for custom training.
This course explores what ML is and what problems it can solve. The course also discusses best practices for implementing machine learning. You’re introduced to Vertex AI, a unified platform to quickly build, train, and deploy AutoML machine learning models. The course discusses the five phases of converting a candidate use case to be driven by machine learning, and why it’s important to not skip them. The course ends with recognizing the biases that ML can amplify and how to recognize them.
Incorporating machine learning into data pipelines increases the ability to extract insights from data. This course covers ways machine learning can be included in data pipelines on Google Cloud. For little to no customization, this course covers AutoML. For more tailored machine learning capabilities, this course introduces Notebooks and BigQuery machine learning (BigQuery ML). Also, this course covers how to productionalize machine learning solutions by using Vertex AI.
This course is part 1 of a 3-course series on Serverless Data Processing with Dataflow. In this first course, we start with a refresher of what Apache Beam is and its relationship with Dataflow. Next, we talk about the Apache Beam vision and the benefits of the Beam Portability framework. The Beam Portability framework achieves the vision that a developer can use their favorite programming language with their preferred execution backend. We then show you how Dataflow allows you to separate compute and storage while saving money, and how identity, access, and management tools interact with your Dataflow pipelines. Lastly, we look at how to implement the right security model for your use case on Dataflow.
Processing streaming data is becoming increasingly popular as streaming enables businesses to get real-time metrics on business operations. This course covers how to build streaming data pipelines on Google Cloud. Pub/Sub is described for handling incoming streaming data. The course also covers how to apply aggregations and transformations to streaming data using Dataflow, and how to store processed records to BigQuery or Bigtable for analysis. Learners get hands-on experience building streaming data pipeline components on Google Cloud by using QwikLabs.
Data pipelines typically fall under one of the Extract and Load (EL), Extract, Load and Transform (ELT) or Extract, Transform and Load (ETL) paradigms. This course describes which paradigm should be used and when for batch data. Furthermore, this course covers several technologies on Google Cloud for data transformation including BigQuery, executing Spark on Dataproc, pipeline graphs in Cloud Data Fusion and serverless data processing with Dataflow. Learners get hands-on experience building data pipeline components on Google Cloud using Qwiklabs.
This course helps learners create a study plan for the PDE (Professional Data Engineer) certification exam. Learners explore the breadth and scope of the domains covered in the exam. Learners assess their exam readiness and create their individual study plan.
The two key components of any data pipeline are data lakes and warehouses. This course highlights use-cases for each type of storage and dives into the available data lake and warehouse solutions on Google Cloud in technical detail. Also, this course describes the role of a data engineer, the benefits of a successful data pipeline to business operations, and examines why data engineering should be done in a cloud environment. This is the first course of the Data Engineering on Google Cloud series. After completing this course, enroll in the Building Batch Data Pipelines on Google Cloud course.
This course introduces the Google Cloud big data and machine learning products and services that support the data-to-AI lifecycle. It explores the processes, challenges, and benefits of building a big data pipeline and machine learning models with Vertex AI on Google Cloud.