Adrian Kosmaczewski
Jest członkiem od 2024
Liga diamentowa
18880 pkt.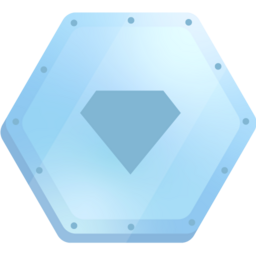
Jest członkiem od 2024
Im szerzej wykorzystuje się w firmach sztuczną inteligencję i systemy uczące się, tym większej wagi nabiera odpowiedzialne podejście do opracowywania tych technologii. Wielu organizacjom trudniej jest jednak wprowadzić zasady odpowiedzialnej AI w praktyce niż tylko o tym rozmawiać. To szkolenie jest przeznaczone dla osób, które chcą się dowiedzieć, jak wdrożyć odpowiedzialną AI w swojej organizacji. W jego trakcie dowiesz się, jak robimy to w Google Cloud, oraz poznasz sprawdzone metody i wnioski z naszych działań w tym zakresie. Pomoże Ci to opracować własne podejście do odpowiedzialnej AI.
Aby zdobyć odznakę umiejętności, ukończ szkolenia Introduction to Generative AI, Introduction to Large Language Models i Introduction to Responsible AI. Zdaj test i pokaż, że rozumiesz podstawowe koncepcje związane z generatywną AI. Odznaka umiejętności to cyfrowa odznaka wydawana przez Google Cloud, która potwierdza Twoją wiedzę o produktach i usługach Google Cloud. Ustaw swój profil jako publiczny i dodaj odznakę umiejętności do profilu w mediach społecznościowych, aby pochwalić się swoim osiągnięciem.
Celem tego szybkiego szkolenia dla początkujących jest wyjaśnienie, czym jest odpowiedzialna AI i dlaczego jest ważna, oraz przedstawienie, jak Google wprowadza ją w swoich usługach. Szkolenie zawiera także wprowadzenie do siedmiu zasad Google dotyczących sztucznej inteligencji.
To szybkie szkolenie dla początkujących wyjaśnia, czym są duże modele językowe (LLM) oraz jakie są ich zastosowania. Przedstawia również możliwości zwiększenia ich wydajności przez dostrajanie przy użyciu promptów oraz narzędzia Google, które pomogą Ci tworzyć własne aplikacje korzystające z generatywnej AI.
Celem tego szybkiego szkolenia dla początkujących jest wyjaśnienie, czym jest generatywna AI oraz jakie są jej zastosowania. Szkolenie przedstawia również różnice pomiędzy tą technologią a tradycyjnymi systemami uczącymi się, a także narzędzia Google, które pomogą Ci tworzyć własne aplikacje korzystające z generatywnej AI.
This course covers how to implement the various flavors of production ML systems— static, dynamic, and continuous training; static and dynamic inference; and batch and online processing. You delve into TensorFlow abstraction levels, the various options for doing distributed training, and how to write distributed training models with custom estimators. This is the second course of the Advanced Machine Learning on Google Cloud series. After completing this course, enroll in the Image Understanding with TensorFlow on Google Cloud course.
This course takes a real-world approach to the ML Workflow through a case study. An ML team faces several ML business requirements and use cases. The team must understand the tools required for data management and governance and consider the best approach for data preprocessing. The team is presented with three options to build ML models for two use cases. The course explains why they would use AutoML, BigQuery ML, or custom training to achieve their objectives.
This course explores the benefits of using Vertex AI Feature Store, how to improve the accuracy of ML models, and how to find which data columns make the most useful features. This course also includes content and labs on feature engineering using BigQuery ML, Keras, and TensorFlow.
This course covers designing and building a TensorFlow input data pipeline, building ML models with TensorFlow and Keras, improving the accuracy of ML models, writing ML models for scaled use, and writing specialized ML models.
The course begins with a discussion about data: how to improve data quality and perform exploratory data analysis. We describe Vertex AI AutoML and how to build, train, and deploy an ML model without writing a single line of code. You will understand the benefits of Big Query ML. We then discuss how to optimize a machine learning (ML) model and how generalization and sampling can help assess the quality of ML models for custom training.
This course introduces the AI and machine learning (ML) offerings on Google Cloud that build both predictive and generative AI projects. It explores the technologies, products, and tools available throughout the data-to-AI life cycle, encompassing AI foundations, development, and solutions. It aims to help data scientists, AI developers, and ML engineers enhance their skills and knowledge through engaging learning experiences and practical hands-on exercises.