Bill Kapsalis
成为会员时间:2017
黄金联赛
55905 积分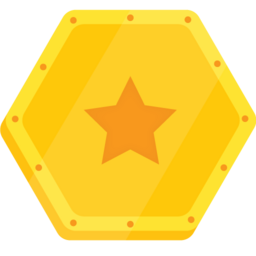
成为会员时间:2017
本课程介绍 Google Cloud 中的 AI 和机器学习 (ML) 服务,这些服务可构建预测式和生成式 AI 项目。本课程探讨从数据到 AI 的整个生命周期中可用的技术、产品和工具,包括 AI 基础、开发和解决方案。通过引人入胜的学习体验和实操练习,本课程可帮助数据科学家、AI 开发者和机器学习工程师提升技能和知识水平。
Complete the introductory Build a Data Mesh with Dataplex skill badge to demonstrate skills in the following: building a data mesh with Dataplex to facilitate data security, governance, and discovery on Google Cloud. You practice and test your skills in tagging assets, assigning IAM roles, and assessing data quality in Dataplex. A skill badge is an exclusive digital badge issued by Google Cloud in recognition of your proficiency with Google Cloud products and services and tests your ability to apply your knowledge in an interactive hands-on environment. Complete this Skill Badge, and the final assessment challenge lab, to receive a digital badge that you can share with your network.
In this course, you learn about containers and how to build, and package container images. The content in this course includes best practices for creating and securing containers, and provides an introduction to Cloud Run and Google Kubernetes Engine for application developers.
完成借助 Firebase 开发无服务器应用技能徽章中级课程, 展示您在以下方面的技能:借助 Firebase 设计无服务器 Web 应用架构以及构建无服务器 Web 应用; 利用 Firestore 管理数据库;利用 Cloud Build 自动完成部署流程; 以及将 Google 助理功能集成到您的应用中。 技能徽章 是由 Google Cloud 颁发的专属数字徽章,旨在认可 您在 Google Cloud 产品与服务方面的熟练度;您需要在 交互式实操环境中参加考核,证明自己运用所学知识的能力后才能获得。完成此技能 徽章课程和作为最终评估的实验室挑战赛,获得技能徽章, 在您的人际圈中炫出自己的技能。
完成中级技能徽章课程利用 BigQuery ML 处理预测模型的工程师数据, 展示自己在以下方面的技能:利用 Dataprep by Trifacta 构建 BigQuery 数据转换流水线; 利用 Cloud Storage、Dataflow 和 BigQuery 构建提取、转换和加载 (ETL) 工作流; 利用 BigQuery ML 构建机器学习模型; 以及利用 Cloud Composer 跨多个位置复制数据。 技能徽章是由 Google Cloud 颁发的专属数字徽章,旨在认可 您对 Google Cloud 产品与服务的熟练度;您需要在 交互式实操环境中参加考核,证明自己运用所学知识的能力后才能获得此徽章。完成技能徽章课程和 作为最终评估的实验室挑战赛,即可获得数字徽章, 在您的人际圈中炫出自己的技能。
本课程向您介绍扩散模型。这类机器学习模型最近在图像生成领域展现出了巨大潜力。扩散模型的灵感来源于物理学,特别是热力学。过去几年内,扩散模型成为热门研究主题并在整个行业开始流行。Google Cloud 上许多先进的图像生成模型和工具都是以扩散模型为基础构建的。本课程向您介绍扩散模型背后的理论,以及如何在 Vertex AI 上训练和部署此类模型。
随着企业对人工智能和机器学习的应用越来越广泛,以负责任的方式构建这些技术也变得更加重要。但对很多企业而言,真正践行 Responsible AI 并非易事。如果您有意了解如何在组织内践行 Responsible AI,本课程正适合您。 本课程将介绍 Google Cloud 目前如何践行 Responsible AI,以及从中总结的最佳实践和经验教训,便于您以此为框架构建自己的 Responsible AI 方法。
This short course on integrating applications with Gemini 1.0 Pro models on Google Cloud helps you discover the Gemini API and its generative AI models. The course teaches you how to access the Gemini 1.0 Pro and Gemini 1.0 Pro Vision models from code. It lets you test the capabilities of the models with text, image, and video prompts from an app.
In the last installment of the Dataflow course series, we will introduce the components of the Dataflow operational model. We will examine tools and techniques for troubleshooting and optimizing pipeline performance. We will then review testing, deployment, and reliability best practices for Dataflow pipelines. We will conclude with a review of Templates, which makes it easy to scale Dataflow pipelines to organizations with hundreds of users. These lessons will help ensure that your data platform is stable and resilient to unanticipated circumstances.
In this second installment of the Dataflow course series, we are going to be diving deeper on developing pipelines using the Beam SDK. We start with a review of Apache Beam concepts. Next, we discuss processing streaming data using windows, watermarks and triggers. We then cover options for sources and sinks in your pipelines, schemas to express your structured data, and how to do stateful transformations using State and Timer APIs. We move onto reviewing best practices that help maximize your pipeline performance. Towards the end of the course, we introduce SQL and Dataframes to represent your business logic in Beam and how to iteratively develop pipelines using Beam notebooks.
Complete the introductory Create and Manage AlloyDB Instances skill badge to demonstrate skills in the following: performing core AlloyDB operations and tasks, migrating to AlloyDB from PostgreSQL, administering an AlloyDB database, and accelerating analytical queries using the AlloyDB Columnar Engine. A skill badge is an exclusive digital badge issued by Google Cloud in recognition of your proficiency with Google Cloud products and services and tests your ability to apply your knowledge in an interactive hands-on environment. Complete this skill badge course, and the final assessment challenge lab, to receive a skill badge that you can share with your network.
Complete the intermediate Create and Manage Bigtable Instances skill badge to demonstrate skills in the following: creating instances, designing schemas, querying data, and performing administrative tasks in Bigtable including monitoring performance and configuring node autoscaling and replication. A skill badge is an exclusive digital badge issued by Google Cloud in recognition of your proficiency with Google Cloud products and services and tests your ability to apply your knowledge in an interactive hands-on environment. Complete this Skill Badge, and the final assessment challenge lab, to receive a digital badge that you can share with your network.
This course introduces participants to MLOps tools and best practices for deploying, evaluating, monitoring and operating production ML systems on Google Cloud. MLOps is a discipline focused on the deployment, testing, monitoring, and automation of ML systems in production. Learners will get hands-on practice using Vertex AI Feature Store's streaming ingestion at the SDK layer.
Complete the introductory Create and Manage Cloud Spanner Instances skill badge to demonstrate skills in the following: creating and interacting with Cloud Spanner instances and databases; loading Cloud Spanner databases using various techniques; backing up Cloud Spanner databases; defining schemas and understanding query plans; and deploying a Modern Web App connected to a Cloud Spanner instance. A skill badge is an exclusive digital badge issued by Google Cloud in recognition of your proficiency with Google Cloud products and services and tests your ability to apply your knowledge in an interactive hands-on environment. Complete this skill badge course, and the final assessment challenge lab, to receive a skill badge that you can share with your network.
Complete the introductory Create and Manage Cloud SQL for PostgreSQL Instances skill badge to demonstrate skills in the following: migrating, configuring, and managing Cloud SQL for PostgreSQL instances and databases. A skill badge is an exclusive digital badge issued by Google Cloud in recognition of your proficiency with Google Cloud products and services and tests your ability to apply your knowledge in an interactive hands-on environment. Complete this skill badge course, and the final assessment challenge lab, to receive a skill badge that you can share with your network.
Complete the introductory Migrate MySQL data to Cloud SQL using Database Migration Services skill badge to demonstrate skills in the following: migrating MySQL data to Cloud SQL using different job types and connectivity options available in Database Migration Service and migrating MySQL user data when running Database Migration Service jobs. A skill badge is an exclusive digital badge issued by Google Cloud in recognition of your proficiency with Google Cloud products and services and tests your ability to apply your knowledge in an interactive hands-on environment. Complete this skill badge quest, and the final assessment challenge lab, to receive a skill badge that you can share with your network.
This course is intended to give architects, engineers, and developers the skills required to help enterprise customers architect, plan, execute, and test database migration projects. Through a combination of presentations, demos, and hands-on labs participants move databases to Google Cloud while taking advantage of various services. This course covers how to move on-premises, enterprise databases like SQL Server to Google Cloud (Compute Engine and Cloud SQL) and Oracle to Google Cloud bare metal.
Google Workspace 专用 Gemini 是一个插件,可在 Google Workspace 中为客户提供生成式 AI 功能。在本迷你课程中,您将了解 Gemini 的主要功能,以及如何在 Gmail 中使用这些功能来提高工作效率。
Google Workspace 专用 Gemini 是一个插件,可在 Google Workspace 中为客户提供生成式 AI 功能。在本学习路线中,您将了解 Gemini 的主要功能,以及如何在 Google Workspace 中使用它们来提高工作效率。
在本课程中,您将了解 Google Cloud 中依托生成式 AI 技术的协作工具 Gemini 如何帮助开发者构建应用。您将学习如何向 Gemini 输入提示,让其为您解释代码、推荐 Google Cloud 服务并为您的应用生成代码。您将通过实操实验体验 Gemini 对应用开发工作流的改进作用。 Duet AI 已更名为 Gemini,这是我们的新一代模型。
在本课程中,您将了解 Gemini(Google Cloud 推出的一款依托生成式 AI 的协作工具)如何帮助您使用 Google 产品和服务开发、测试、部署和管理应用。在 Gemini 的协助下,您可以学习如何开发和构建 Web 应用、修复应用中的错误、开发测试和查询数据。您可以通过实操实验了解如何利用 Gemini 来改进软件开发生命周期 (SDLC)。 Duet AI 已更名为 Gemini,这是我们的新一代模型。
在本课程中,您将了解 Gemini(Google Cloud 推出的一款依托生成式 AI 的协作工具)如何帮助工程师管理基础设施。您将了解如何向 Gemini 输入提示,让其查找和理解应用日志、创建 GKE 集群,以及研究如何创建构建环境。您可以通过实操实验了解如何利用 Gemini 来改进 DevOps 工作流。 Duet AI 已更名为 Gemini,这是我们的新一代模型。
在本课程中,您将了解 Gemini(Google Cloud 推出的一款依托生成式 AI 的协作工具)如何帮助您保护您的云环境和资源。您将学习如何将示例工作负载部署到 Google Cloud 环境中,以及如何借助 Gemini 识别和修复安全配置错误。您可以通过实操实验了解如何利用 Gemini 来改善云安全状况。 Duet AI 已更名为 Gemini,这是我们的新一代模型。
在本课程中,您将了解 Gemini(Google Cloud 推出的一款依托生成式 AI 的协作工具)如何帮助网络工程师创建、更新和维护 VPC 网络。您将学习如何向 Gemini 输入提示,让其针对您的网络组建和管理任务,提供您从搜索引擎所无法获得的具体指导。您可以通过实操实验了解如何利用 Gemini 更轻松地使用 Google Cloud VPC 网络。 Duet AI 已更名为 Gemini,这是我们的新一代模型。
在本课程中,您将了解 Gemini(Google Cloud 的生成式 AI 赋能的协作工具)如何帮助分析客户数据并预测产品销售情况。此外,您还将了解如何在 BigQuery 中使用客户数据来识别、开发新客户并对其进行分类。通过动手实验,您将体验 Gemini 如何改进数据分析和机器学习工作流。 Duet AI 已更名为 Gemini,这是我们的新一代模型。
在本课程中,您将了解 Gemini(Google Cloud 的生成式 AI 赋能的协作工具)如何帮助管理员预配基础设施。您将了解如何通过输入提示来让 Gemini 解释基础设施、GKE 集群的部署,以及现有基础设施的更新。您可以通过实操实验了解如何利用 Gemini 来改进 GKE 部署工作流。 Duet AI 已更名为 Gemini,这是我们的新一代模型。
完成 Introduction to Generative AI、Introduction to Large Language Models 和 Introduction to Responsible AI 三门课程,赢取技能徽章。通过最终测验,即表明您理解了生成式 AI 的基本概念。 技能徽章是由 Google Cloud 颁发的数字徽章,旨在认可您对 Google Cloud 产品与服务的了解程度。公开您的个人资料并将技能徽章添加到您的社交媒体个人资料中,以此来分享您获得的成就。
这是一节入门级微课程,旨在解释什么是负责任的 AI、它的重要性,以及 Google 如何在自己的产品中实现负责任的 AI。此外,本课程还介绍了 Google 的 7 个 AI 开发原则。
这是一节入门级微学习课程,探讨什么是大型语言模型 (LLM)、适合的应用场景以及如何使用提示调整来提升 LLM 性能,还介绍了可以帮助您开发自己的 Gen AI 应用的各种 Google 工具。
这是一节入门级微课程,旨在解释什么是生成式 AI、它的用途以及与传统机器学习方法的区别。该课程还介绍了可以帮助您开发自己的生成式 AI 应用的各种 Google 工具。
In this course, application developers learn how to design and develop cloud-native applications that seamlessly integrate components from the Google Cloud ecosystem. Through a combination of presentations, demos, and hands-on labs, participants learn how to create repeatable deployments by treating infrastructure as code, choose the appropriate application execution environment for an application, and monitor application performance. Completing one version of each lab is required. Each lab is available in Node.js. In most cases, the same labs are also provided in Python or Java. You may complete each lab in whichever language you prefer.
In this course, application developers learn how to design and develop cloud-native applications that seamlessly integrate managed services from Google Cloud. Through a combination of presentations, demos, and hands-on labs, participants learn how to develop more secure applications, implement federated identity management, and integrate application components by using messaging, event-driven processing, and API gateways. Completing one version of each lab is required. Each lab is available in Node.js. In most cases, the same labs are also provided in Python or Java. You may complete each lab in whichever language you prefer. This is the second course of the Developing Applications with Google Cloud series. After completing this course, enroll in the App Deployment, Debugging, and Performance course.
“Google Cloud 基础知识:核心基础架构”介绍在使用 Google Cloud 时会遇到的重要概念和术语。本课程通过视频和实操实验来介绍并比较 Google Cloud 的多种计算和存储服务,并提供重要的资源和政策管理工具。
This course describes different types of computer vision use cases and then highlights different machine learning strategies for solving these use cases. The strategies vary from experimenting with pre-built ML models through pre-built ML APIs and AutoML Vision to building custom image classifiers using linear models, deep neural network (DNN) models or convolutional neural network (CNN) models. The course shows how to improve a model's accuracy with augmentation, feature extraction, and fine-tuning hyperparameters while trying to avoid overfitting the data. The course also looks at practical issues that arise, for example, when one doesn't have enough data and how to incorporate the latest research findings into different models. Learners will get hands-on practice building and optimizing their own image classification models on a variety of public datasets in the labs they will work on.
In this course, you will be learning from ML Engineers and Trainers who work with the state-of-the-art development of ML pipelines here at Google Cloud. The first few modules will cover about TensorFlow Extended (or TFX), which is Google’s production machine learning platform based on TensorFlow for management of ML pipelines and metadata. You will learn about pipeline components and pipeline orchestration with TFX. You will also learn how you can automate your pipeline through continuous integration and continuous deployment, and how to manage ML metadata. Then we will change focus to discuss how we can automate and reuse ML pipelines across multiple ML frameworks such as tensorflow, pytorch, scikit learn, and xgboost. You will also learn how to use another tool on Google Cloud, Cloud Composer, to orchestrate your continuous training pipelines. And finally, we will go over how to use MLflow for managing the complete machine learning life cycle.
This course introduces participants to MLOps tools and best practices for deploying, evaluating, monitoring and operating production ML systems on Google Cloud. MLOps is a discipline focused on the deployment, testing, monitoring, and automation of ML systems in production. Machine Learning Engineering professionals use tools for continuous improvement and evaluation of deployed models. They work with (or can be) Data Scientists, who develop models, to enable velocity and rigor in deploying the best performing models.
完成入门级技能徽章课程在 Google Cloud 上为机器学习 API 准备数据,展示以下技能: 使用 Dataprep by Trifacta 清理数据、在 Dataflow 中运行数据流水线、在 Dataproc 中创建集群和运行 Apache Spark 作业,以及调用机器学习 API,包括 Cloud Natural Language API、Google Cloud Speech-to-Text API 和 Video Intelligence API。 技能徽章是由 Google Cloud 颁发的专属数字徽章,旨在认可您在 Google Cloud 产品与服务方面的熟练度; 您需要在交互式实操环境中参加考核,证明自己运用所学知识的能力后才能获得。完成此技能徽章课程和作为最终评估的实验室挑战赛, 获得技能徽章,在您的人际圈中炫出自己的技能。
Earn the intermediate skill badge by completing the Build and Deploy Machine Learning Solutions with Vertex AI course, where you will learn how to use Google Cloud's Vertex AI platform, AutoML, and custom training services to train, evaluate, tune, explain, and deploy machine learning models. This skill badge course is for professional Data Scientists and Machine Learning Engineers. A skill badge is an exclusive digital badge issued by Google Cloud in recognition of your proficiency with Google Cloud products and services and tests your ability to apply your knowledge in an interactive hands-on environment. Complete this Skill Badge, and the final assessment challenge lab, to receive a digital badge that you can share with your network.
In this course, you apply your knowledge of classification models and embeddings to build a ML pipeline that functions as a recommendation engine. This is the fifth and final course of the Advanced Machine Learning on Google Cloud series.
This course introduces the products and solutions to solve NLP problems on Google Cloud. Additionally, it explores the processes, techniques, and tools to develop an NLP project with neural networks by using Vertex AI and TensorFlow.
This course covers how to implement the various flavors of production ML systems— static, dynamic, and continuous training; static and dynamic inference; and batch and online processing. You delve into TensorFlow abstraction levels, the various options for doing distributed training, and how to write distributed training models with custom estimators. This is the second course of the Advanced Machine Learning on Google Cloud series. After completing this course, enroll in the Image Understanding with TensorFlow on Google Cloud course.
This course takes a real-world approach to the ML Workflow through a case study. An ML team faces several ML business requirements and use cases. The team must understand the tools required for data management and governance and consider the best approach for data preprocessing. The team is presented with three options to build ML models for two use cases. The course explains why they would use AutoML, BigQuery ML, or custom training to achieve their objectives.
This course explores the benefits of using Vertex AI Feature Store, how to improve the accuracy of ML models, and how to find which data columns make the most useful features. This course also includes content and labs on feature engineering using BigQuery ML, Keras, and TensorFlow.
This course covers building ML models with TensorFlow and Keras, improving the accuracy of ML models and writing ML models for scaled use.
The course begins with a discussion about data: how to improve data quality and perform exploratory data analysis. We describe Vertex AI AutoML and how to build, train, and deploy an ML model without writing a single line of code. You will understand the benefits of Big Query ML. We then discuss how to optimize a machine learning (ML) model and how generalization and sampling can help assess the quality of ML models for custom training.
This course explores what ML is and what problems it can solve. The course also discusses best practices for implementing machine learning. You’re introduced to Vertex AI, a unified platform to quickly build, train, and deploy AutoML machine learning models. The course discusses the five phases of converting a candidate use case to be driven by machine learning, and why it’s important to not skip them. The course ends with recognizing the biases that ML can amplify and how to recognize them.
This course is part 1 of a 3-course series on Serverless Data Processing with Dataflow. In this first course, we start with a refresher of what Apache Beam is and its relationship with Dataflow. Next, we talk about the Apache Beam vision and the benefits of the Beam Portability framework. The Beam Portability framework achieves the vision that a developer can use their favorite programming language with their preferred execution backend. We then show you how Dataflow allows you to separate compute and storage while saving money, and how identity, access, and management tools interact with your Dataflow pipelines. Lastly, we look at how to implement the right security model for your use case on Dataflow.
Incorporating machine learning into data pipelines increases the ability to extract insights from data. This course covers ways machine learning can be included in data pipelines on Google Cloud. For little to no customization, this course covers AutoML. For more tailored machine learning capabilities, this course introduces Notebooks and BigQuery machine learning (BigQuery ML). Also, this course covers how to productionalize machine learning solutions by using Vertex AI.
Data pipelines typically fall under one of the Extract and Load (EL), Extract, Load and Transform (ELT) or Extract, Transform and Load (ETL) paradigms. This course describes which paradigm should be used and when for batch data. Furthermore, this course covers several technologies on Google Cloud for data transformation including BigQuery, executing Spark on Dataproc, pipeline graphs in Cloud Data Fusion and serverless data processing with Dataflow. Learners get hands-on experience building data pipeline components on Google Cloud using Qwiklabs.
The two key components of any data pipeline are data lakes and warehouses. This course highlights use-cases for each type of storage and dives into the available data lake and warehouse solutions on Google Cloud in technical detail. Also, this course describes the role of a data engineer, the benefits of a successful data pipeline to business operations, and examines why data engineering should be done in a cloud environment. This is the first course of the Data Engineering on Google Cloud series. After completing this course, enroll in the Building Batch Data Pipelines on Google Cloud course.
Processing streaming data is becoming increasingly popular as streaming enables businesses to get real-time metrics on business operations. This course covers how to build streaming data pipelines on Google Cloud. Pub/Sub is described for handling incoming streaming data. The course also covers how to apply aggregations and transformations to streaming data using Dataflow, and how to store processed records to BigQuery or Bigtable for analysis. Learners get hands-on experience building streaming data pipeline components on Google Cloud by using QwikLabs.
This course helps learners create a study plan for the PDE (Professional Data Engineer) certification exam. Learners explore the breadth and scope of the domains covered in the exam. Learners assess their exam readiness and create their individual study plan.
This course introduces the Google Cloud big data and machine learning products and services that support the data-to-AI lifecycle. It explores the processes, challenges, and benefits of building a big data pipeline and machine learning models with Vertex AI on Google Cloud.
These labs help you get started with the skills you need to develop and train ML models. At the end of each lab, you’ll have hands-on experience with one or more of Google Cloud’s powerful data tools. Complete this game to earn a badge, and you’ll be one step closer to completing the challenge. Race the clock to increase your score and watch your name rise on the leaderboard!
These labs help you get started with the skills you need to analyze sports-related data. At the end of each lab, you’ll have hands-on experience with one or more of Google Cloud’s powerful data tools. Complete this game to earn a badge, and you’ll be one step closer to completing the challenge. Race the clock to increase your score and watch your name rise on the leaderboard!
This is the second of two Quests of hands-on labs derived from the exercises from the book Data Science on Google Cloud Platform, 2nd Edition by Valliappa Lakshmanan, published by O'Reilly Media, Inc. In this second Quest, covering chapter 9 through the end of the book, you extend the skills practiced in the first Quest, and run full-fledged machine learning jobs with state-of-the-art tools and real-world data sets, all using Google Cloud tools and services.
Welcome to the Learn to Earn Cloud Data Challenge! These labs help you get started with data analysis skills. At the end of each lab, you’ll have hands-on experience with one or more of Google Cloud’s powerful data tools. Complete this game to earn a badge, and you’ll be one step closer to completing the challenge. Race the clock to increase your score and watch your name rise on the leaderboard!
完成中级技能徽章课程使用 BigQuery 构建数据仓库,展示以下技能: 联接数据以创建新表、排查联接故障、使用并集附加数据、创建日期分区表, 以及在 BigQuery 中使用 JSON、数组和结构体。 技能徽章是 Google Cloud 颁发的专属数字徽章, 旨在认可您在 Google Cloud 产品与服务方面的熟练度; 您需要在交互式实操环境中参加考核,证明自己运用所学知识的能力后 才能获得。完成此技能徽章课程和作为最终评估的实验室挑战赛, 获得数字徽章,在您的人际圈中炫出自己的技能。
Welcome to the Learn To Earn Cloud Challenge data plus track! "The Google Cloud Certified Professional Data Engineer certification is associated with the highest paying salary in IT," according to the most recent Global Knowledge skills and salary report (published August 2021). Complete this game to earn the Data Plus game badge, and be eligible for a +bonus+ prize in the Learn to Earn Cloud Challenge. See "what's next" below for details and requirements; you’ll need to earn at least 2 additional challenge badges to qualify. You'll learn next-level data skills to add to your resume. Race the clock to increase your score and watch your name rise on the leaderboard. Good luck!
In this quest, you will gain hands-on experience on several topics in Google Workspace Administration including security, provisioning users and groups, managing applications, and managing Google Meet.
Welcome to the Learn To Earn Cloud Challenge security track! These eight labs give you the keys to understanding GCP's powerful security suite. At the end of each lab, you'll have hands-on experience with securing your cloud. Complete this game to earn the Security game badge, and you'll be one step closer to collecting all four badges (see "what's next" below for more information). Race the clock to increase your score and watch your name rise on the leaderboard. Good luck!
Welcome to the Learn To Earn Cloud Challenge architecture track! These eight labs give you a blueprint of GCP's building blocks. At the end of each lab, you'll have hands-on experience with another tool or service to add to your resume. Complete this game to earn the Architecture game badge, and you'll be one step closer to collecting all four Learn to Earn Cloud Challenge badges (see "what's next" below for more information). Race the clock to increase your score and watch your name rise on the leaderboard. Good luck!
Welcome to the Learn To Earn Cloud Challenge data track! These eight labs give you a deep dive into GCP's data universe. At the end of each lab, you'll have another in-demand skill to add to your list. Complete this game to earn the Data game badge, and you'll be one step closer to collecting all four Learn to Earn Cloud Challenge badges (see "what's next" below for more information). Race the clock to increase your score and watch your name rise on the leaderboard. Good luck!
Welcome to the Learn To Earn Cloud Challenge! These eight labs give you a quick hands-on introduction to eight different GCP tools and services. At the end of each lab, you'll have another skill to add to your list. Complete this game to earn the Essentials game badge, and you'll be one step closer to collecting all four Learn to Earn Cloud Challenge badges (see "what's next" below for more information). Race the clock to increase your score and watch your name rise on the leaderboard. Good luck!
Earn the advanced skill badge by completing the Use Machine Learning APIs on Google Cloud course, where you learn the basic features for the following machine learning and AI technologies: Cloud Vision API, Cloud Cloud Translation API, and Cloud Natural Language API. A skill badge is an exclusive digital badge issued by Google Cloud in recognition of your proficiency with Google Cloud products and services and tests your ability to apply your knowledge in an interactive hands-on environment. Complete this skill badge course, and the final assessment challenge lab, to receive a skill badge that you can share with your network.
In honor of the Launch of the Google Cloud Community and the Learning & Certification Hub (bit.ly/3gNtlMB), we’ve devised a fun little game to catapult your skills in BigQuery and give you a chance to win the First. Ever. Community. Learning. Challenge. (Pause for effect) Not only will participating in this game give you hands-on practice to boost key skills in BQ, but simply participating will earn you some sweet online badges. Plus, the winner of the game will be rewarded...handsomely . More details below... Complete every activity in this game and earn a Skill Badge which you can share across your network with pride. Complete every activity AND post a screenshot of your newly minted Skill Badge in the Learning Forum area of the Community (bit.ly/35LoLIB) to earn a special Community Badge which you can then flaunt over other community members shamelessly. The Community member at the top of the scoreboard at the time the game is closed will be rewarded with something objectively …
This advanced-level quest is unique amongst the other catalog offerings. The labs have been curated to give IT professionals hands-on practice with topics and services that appear in the Google Cloud Certified Professional Data Engineer Certification. From Big Query, to Dataprep, to Cloud Composer, this quest is composed of specific labs that will put your Google Cloud data engineering knowledge to the test. Be aware that while practice with these labs will increase your skills and abilities, you will need other preparation, too. The exam is quite challenging and external studying, experience, and/or background in cloud data engineering is recommended. Looking for a hands on challenge lab to demonstrate your skills and validate your knowledge? On completing this quest, enroll in and finish the additional challenge lab at the end of the Engineer Data in the Google Cloud to receive an exclusive Google Cloud digital badge.
This is the first of two Quests of hands-on labs is derived from the exercises from the book Data Science on Google Cloud Platform, 2nd Edition by Valliappa Lakshmanan, published by O'Reilly Media, Inc. In this first Quest, covering up through chapter 8, you are given the opportunity to practice all aspects of ingestion, preparation, processing, querying, exploring and visualizing data sets using Google Cloud tools and services.
In this advanced-level quest, you will learn how to harness serious Google Cloud computing power to run big data and machine learning jobs. The hands-on labs will give you use cases, and you will be tasked with implementing big data and machine learning practices utilized by Google’s very own Solutions Architecture team. From running Big Query analytics on tens of thousands of basketball games, to training TensorFlow image classifiers, you will quickly see why Google Cloud is the go-to platform for running big data and machine learning jobs.